diff --git a/docs/en/alab/annotation_labs_releases/release_notes_6_2_0.md b/docs/en/alab/annotation_labs_releases/release_notes_6_2_0.md
index 19d0c123a6..7aa6056870 100644
--- a/docs/en/alab/annotation_labs_releases/release_notes_6_2_0.md
+++ b/docs/en/alab/annotation_labs_releases/release_notes_6_2_0.md
@@ -362,7 +362,7 @@ Users can confidently upload and import files, knowing that the system will enfo
### There should be a way to "batch" clear predicted labels in a Section after pre-annotation
-Version 6.2 of Generative AI Lab introduces a significant enhancement improving the application's security and robustness by restricting the types of files that can be uploaded or imported. This change ensures that only supported and safe file types are processed, providing a more secure and efficient user experience, maintaining the platform's integrity and reliability while enhancing its security.
+Version 6.2 of Generative AI Lab introduces a tool to allow annotators to remove large sections of pre-annotated labels in bulk instead of removing them individually.
**Key Features of This Improvement:**
diff --git a/docs/en/alab/de_identify.md b/docs/en/alab/de_identify.md
index 53198019e2..84e6bdabdb 100644
--- a/docs/en/alab/de_identify.md
+++ b/docs/en/alab/de_identify.md
@@ -6,7 +6,7 @@ seotitle: Generative AI Lab | John Snow Labs
title: De-Identification
permalink: /docs/en/alab/de_identify
key: docs-training
-modify_date: "2024-08-26"
+modify_date: "2024-12-03"
use_language_switcher: "Python-Scala"
show_nav: true
sidebar:
@@ -15,19 +15,12 @@ sidebar:
-## Introducing Support for De-Identification in Generative AI Lab 6.4
-We are happy to announce the release of Generative AI Lab 6.4, bringing exciting new features and enhancements. Leading this release is the support for de-identification projects, which enables users to anonymize documents containing sensitive data, such as PII (Personally Identifiable Information) and PHI (Protected Health Information). This ensures robust data privacy and compliance with privacy regulations while maintaining the utility of the data for further analysis and processing.
-
-Additionally, version 6.4 enhances collaboration and quality control in the annotation process by allowing annotators to view completions submitted by reviewers. Annotators can now view and clone reviewed submissions, make corrections, or add comments directly on the annotated chunks, providing clear communication and improving overall annotation quality. The new release also simplifies the identification of differences between two completions by automatically highlighting discrepancies, streamlining the validation process.
-
-Alongside these major updates, this release includes numerous improvements and bug fixes, making Generative AI Lab more efficient and user-friendly than ever.
-
-
-
## Support for De-identification
-Version 6.4 of the Generative AI Lab introduces a new de-identification feature, enabling users to anonymize documents containing sensitive information such as PII (Personally Identifiable Information) and PHI (Protected Health Information). This functionality is intended to protect data privacy and ensure compliance with privacy regulations while preserving the data’s usefulness for subsequent analysis and processing.
+Version 6.4 of Generative AI Lab introduces a new de-identification feature, enabling users to anonymize documents containing sensitive information such as PII (Personally Identifiable Information) and PHI (Protected Health Information). This functionality is intended to protect data privacy and ensure compliance with privacy regulations while preserving the data’s usefulness for subsequent analysis and processing.
+
+Version 6.7 of Generative AI Lab improves on the original feature to allow for custom methods of de-identification for each entity label and support for the newest John Snow Labs De-identification Pipeline.
-**De-identification Projects:** When creating a new project in the Generative AI Lab, users can mark it as De-Identification specific. These projects allow the use of manually trained or pre-trained text-based NER models, together with prompts, rules, and custom labels created by the user for identifying sensitive data inside of tasks. Once the sensitive data is identified (either automatically or manually) and validated by human users, it can be exported for further processing.
+**De-identification Projects** When creating a new project in the Generative AI Lab, users can mark it as De-Identification specific. These projects allow the use of manually trained or pre-trained text-based NER models, together with prompts, rules, and custom labels created by the user for identifying sensitive data inside of tasks. Once the sensitive data is identified (either automatically or manually) and validated by human users, it can be exported for further processing.
When creating De-identification projects make sure you only target sensitive entities as part of your project configuration and avoid annotating relevant data you need for downstream processing as all those entities will be removed when exporting the project tasks as de-identified documents. The best practice, in this case, is to re-use de-identification specific models combined with custom prompts/rules.
**Exporting De-identified Documents:** The tasks of your project with PII/PHI labeled entities can be exported as de-identified documents. During the export process, labeled entities will be replaced by the label names, or special characters (such as "*"), or obfuscated and replaced with fake data. This ensures that sensitive information is removed and not available for downstream analysis.
@@ -47,110 +40,92 @@ Generative AI Lab supports four kinds of de-identification:
### Working with de-identification projects
-**Step 1.** When creating a new project, after defining the project name and general settings, check the de-identification option at the bottom of the Project setup page, and select the type of anonymization you prefer.
+To create a De-identification project, in the first step ot he Project Configuration wizzard, select the `De-identification` template available under the `TEXT` tab.
-
+
-**Step 2.** Configure your project to reuse sensitive labels from existing NER Models, Rules, Prompts. It is also possible to create custom labels that can be used to manually annotate the entities you want to anonymize in your documents.
+### Creating a De-identification Project
+Users can use the de-identification feature if a valid license is available in the application:
+1. **Create a New Project**:
+ During the project configuration, select **De-identification** as the project type.
+2. **Automatic Pipeline Download**:
+ A default de-identification pipeline (`clinical_deidentification`) will automatically download if not previously available or it will use the default de-identification project template. All the downloaded pipelines are available on the **Pipeline** page.
+
+
-When selecting pre-annotation resources for your project, ensure that no critical downstream data is inadvertently identified and removed. For instance, if you pre-annotate documents with models, rules, or prompts that identify diseases, those labels will be anonymized upon export, rendering them unavailable to document consumers.
+
-To mitigate this, employ pre-trained or custom de-identification models and augment them with rules and prompts tailored to your specific use cases (e.g., unique identifiers present in your documents). You can also selectively include specific labels from each model in your project configuration. For example, if age information is essential for your consumers, you can exclude this label from the project configuration to retain the data in your document.
+### New Pipeline Tab and Customization
+In the **Reuse Resource** page, a new **Pipelines Tab** is now available for de-identification projects. Here, all the downloaded de-identification pipelines are listed. Users can also use and apply pre-trained and trained models, rules, and zero-shot prompts.
-
-**Step 3.** Pre-annotate your documents, then have your team review them for any overlooked sensitive data. Once your project is set up and tasks are imported, use the pre-annotation feature to automatically identify sensitive information.
-Incorporate a review process where your team checks the pre-annotations using the standard annotation workflow, making manual corrections or annotations to any sensitive segments as necessary. Ensure that all sensitive information is accurately labeled for effective de-identification.
+
-
-**Step 4.** Export De-identified Documents. After completing the labeling process, proceed to export the de-identified documents. Ensure the "Export with De-identification" option is selected on the export page to generate de-identified documents.
+In the **Customize Labels** page, users can first select the overall de-identification strategies to use. Furthermore, it is also possible to specify parand use entity level configurations.
-During the export process, de-identification is executed based on the type of anonymization selected during project setup. This de-identification option can be updated at any time if necessary.
+
-
+Users can also upload custom obfuscation configurations in JSON format via the Customize Labels page, enabling the seamless reuse of obfuscation rules across multiple projects.
-**Step 5.** Import the de-identified tasks in a new project for further processing. These tasks, once exported, can be re-imported into any text-based project in case you need to extract additional data or in case you want to use them for model training/tuning.
+
-
+
-> **_HOW TO:_** De-identification projects can be easily identified without opening them. A small de-identification icon is displayed in the bottom left corner of the project card, clearly indicating the project's status.
+### De-identification Process
+The de-identification process is similar to the existing pre-annotation workflow:
-> **_LIMITATION:_** Projects must be designated as de-identification projects during their initial creation. It is not possible to convert existing projects or newly created non-de-identification projects into de-identification projects.
+1. **Import Tasks**
-
-### Export of De-identified tasks
-**Completion Submission:** Pre-annotations alone are not sufficient for exporting de-identified data. Only starred completions are considered during the export of de-identified tasks. This means that each task intended for de-identified export must be validated by a human user, with at least one completion marked with a star by an annotator, reviewer, or manager.
+ Initially, tasks are imported, and the `NonDeidentified` tag is automatically added to the tasks. It helps users know which tasks have been deidentified and which are yet to be de-identified.
-**Multiple Submissions:** In instances where multiple submissions exist from various annotators, the de-identification process will prioritize the starred completion from the highest priority user as specified on the Teams page. This ensures that de-identification is based on the most relevant and prioritized annotations.
+ 
-This new de-identification feature significantly enhances data privacy by anonymizing sensitive document information. We are confident that this feature will empower users to handle sensitive data responsibly while maintaining the integrity and usability of their datasets.
+2. **Pre-annotate/De-identify**
-
-## Support for De-identification Pipelines
-Version 6.7.0 updates the existing de-identification feature, which has been significantly expanded to give more control over how de-identification is applied, how different entities are treated, and how to integrate pre-trained de-identification pipelines, models, rules, and zero-shot prompts to help identify and anonymize sensitive data.
+ Click the **De-identification (pre-annotate)** button to deploy the de-identification pipeline and pre-annotate your tasks. During the pre-annotation stage, there is a status indicator (the colored circle) next to each task that changes to either green, red, or grey, just like the pre-annotation status.
-De-identification has now moved from the Project Details page to the Content Type page during Project Configuration, where it is a separate project type.
+ 
-
+3. **Labeling Page**
-### Creating a De-identification Project:
-Users can use the de-identification feature if a valid license is available in the application:
-1. **Create a New Project**:
- During the project configuration, select **De-identification** as the project type.
-2. **Automatic Pipeline Download**:
- A default de-identification pipeline (`clinical_deidentification`) will automatically download if not previously available or it will use the default de-identification project template. All the downloaded pipelines are available on the **Pipeline** page.
-
-
-### New Pipeline Tab and Customization:
-In the **Reuse Resource** page, a new **Pipelines Tab** is now available for de-identification projects. Here, all the downloaded de-identification pipelines are listed. Users can also use and apply pre-trained and trained models, rules, and zero-shot prompts.
+ On the labeling page, users can either make corrections or accept the predictions made by the pipeline.
-
+ 
-In the **Customize Labels** page, users can configure the type of de-identification. Apart from all the deidentification types that are already supported, in version 6.7.0, users can even configure **different de-identification types for different labels** as well.
+4. **Re-run De-identification**
-
-Additionally, users can upload custom obfuscation files in JSON format on the Customize Labels page.
+ After saving and submitting the tasks, users can click the de-identify button again to run the de-identification process. This will change the content of your tasks by applying the specified de-identification configurations on all automatic and manual annotations. You can then view the de-identification results on the labeling page. Users can click the **De-identification View** button (located next to the Compare Completion button), to view the de-identified tasks in comparison with the original version. All de-identified completions will show **(De-identified)** next to the completion ID.
-
+ 
-
+
-### De-identification Process:
-The de-identification process remains similar to the existing pre-annotation workflow:
+### Exporting De-identified Tasks
-1. **Import Tasks**:
- Initially, tasks are imported, and the `NonDeidentified` tag is automatically added to the tasks. It helps users to know which tasks have been deidentified and which are yet to be de-identified.
- 
+Only de-identified completions submitted as **ground truth** are exported. Also, if a task has multiple ground truths from different users, the completion from the user with the **highest priority** will be exported.
-3. **Pre-annotate/De-identify**:
- Click the **De-identification (pre-annotate)** button to deploy the de-identification pipeline and pre-annotate and de-identify tasks. Once the task is pre-annotated and de-identified, the de-identification status changes to either green, red, or grey, just like pre-annotation status.
+
- 
+These updates are built on top of the current structure, ensuring ease of use and a smooth transition without disrupting productivity.
-5. **Labeling Page**:
- On the labeling page, users can either make corrections or accept the predictions made by the pipeline.
+> **_HOW TO:_** De-identification projects can be easily identified without opening them. A small de-identification icon is displayed in the bottom left corner of the project card, clearly indicating the project's status.
- 
-7. **Re-run De-identification**:
- After saving or submitting the tasks, users can click the de-identify button again to run the process on either manually annotated completions or all completions and can view the de-identification in real-time from the labeling page. Users can click the **De-identification View** button (located next to the Compare Completion button), to view the de-identified tasks in real-time. All de-identified completions will show **(De-identified)** next to the completion ID.
+> **_LIMITATION:_** Projects must be designated as de-identification projects during their initial creation. It is not possible to convert existing projects or newly created non-de-identification projects into de-identification projects.
- 
-
+### Export of De-identified tasks
-### Exporting De-identified Tasks:
-Only de-identified completions submitted as **ground truth** are exported. Also, if a task has multiple ground truths from different users, the completion from the user with the **highest priority** will be exported.
-
+**Submitted Completions:** Pre-annotations alone are not sufficient for exporting de-identified data. Only starred completions are considered during the export of de-identified tasks. This means that each task intended for de-identified export must be validated by a human user, with at least one completion marked with a star by an annotator, reviewer, or manager.
-These updates are built on top of the current structure, ensuring ease of use and a smooth transition without disrupting productivity.
+**Multiple Completions:** In cases where multiple submissions exist from various annotators, the de-identification process will prioritize the starred completion from the highest priority user as specified on the Teams page. This ensures that de-identification is based on the most relevant and prioritized annotations.
-
\ No newline at end of file
+This new de-identification feature significantly enhances data privacy by anonymizing sensitive document information. We are confident that this feature will empower users to handle sensitive data responsibly while maintaining the integrity and usability of their datasets.
diff --git a/docs/en/alab/productivity.md b/docs/en/alab/productivity.md
index 106067abe3..89236cf5f7 100644
--- a/docs/en/alab/productivity.md
+++ b/docs/en/alab/productivity.md
@@ -6,7 +6,7 @@ seotitle: Generative AI Lab | John Snow Labs
title: Productivity
permalink: /docs/en/alab/productivity
key: docs-training
-modify_date: "2022-12-13"
+modify_date: "2024-12-03"
use_language_switcher: "Python-Scala"
show_nav: true
sidebar:
@@ -167,3 +167,42 @@ Below are the charts included in the Inter-Annotator Agreement section.
CSV file for specific charts can be downloaded using the new download button which will call specific API endpoints: /api/projects/{project_name}/charts/{chart_type}/download_csv
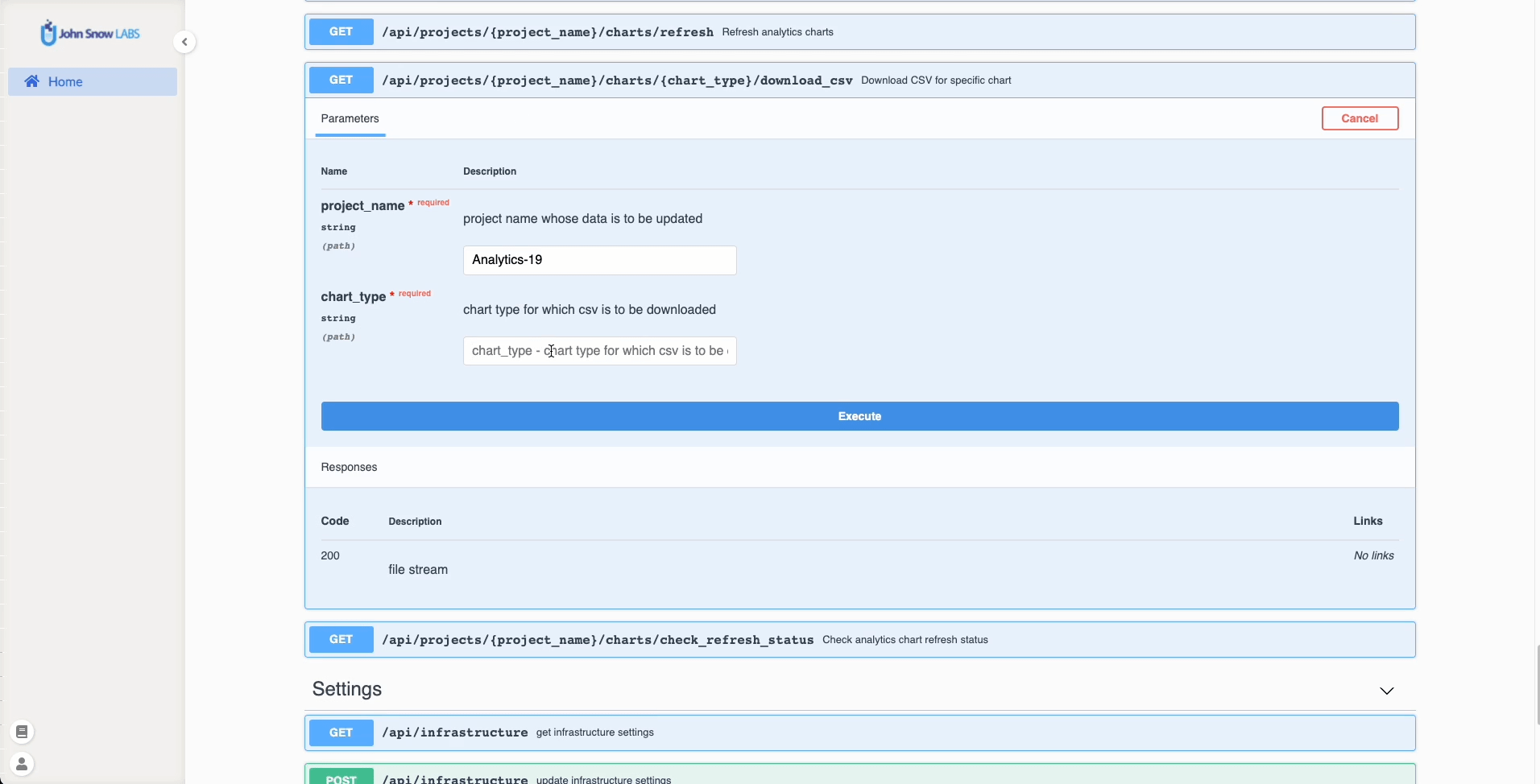
+
+## Annotation Instructions for Labels
+Admin users can add annotation instructions to labels directly from the `Customize Labels` page during project setup.
+
+To add annotation instructions to a label, follow these steps:
+ - Navigate to the `Customize Labels` section, where all your project’s labels are listed.
+ - Click on the `Edit` icon next to the label for which you want to add instructions. This action will take you to the `Edit Label` page.
+ - Enter the guidelines under the `Label Instructions` field.
+ - Click on `Save Label` to store the instructions.
+ - Click on `Save Config` to save the configuration.
+
+
+
+Once annotation instructions are added, they can be viewed from the labeling page in the widget area on the right side. Users can enable or disable the annotation guidelines through the `Annotation Guidelines` toggle. To view the guidelines, the label must first be activated by clicking on it, which will display the label under the `Annotation Guideline` section. Clicking on the label text will then reveal the annotation instructions for that label.
+
+
+
+Users with the Project Manager role can edit and delete annotation guidelines directly from the labeling page. However, users with the Annotator and Reviewer roles can only view the guidelines and do not have permission to edit or delete them.
+
+Remove the annotation instructions from the labeling page:
+
+
+
+Edit the annotation instructions from the Labeling page:
+
+
+
+When multiple labels are selected, the guidelines for each label can be viewed one at a time by clicking on the corresponding label text.
+
+
+
+Annotation guidelines can also be downloaded in JSON format by clicking on the Download icon from the Customize Labels page.
+
+
+
+Additionally, annotation guidelines are available for Assertion Labels as well.
+
+
+
diff --git a/docs/en/alab/project_configuration.md b/docs/en/alab/project_configuration.md
index 94bdcfcf3f..6e39455433 100644
--- a/docs/en/alab/project_configuration.md
+++ b/docs/en/alab/project_configuration.md
@@ -6,7 +6,7 @@ seotitle: Generative AI Lab | John Snow Labs
title: Project Configuration
permalink: /docs/en/alab/project_configuration
key: docs-training
-modify_date: "2022-11-02"
+modify_date: "2022-12-03"
use_language_switcher: "Python-Scala"
show_nav: true
sidebar:
@@ -162,4 +162,55 @@ Below you can find a sample Project Configuration with constraints for Relation
```
+
+
+### Using the Visual Menu Editor
+ With the Visual Menu Builder, users can easily create, edit, and manage project configurations through a user-friendly interface without the need to understand XML as demonstrated above. This makes the configuration process much more straightforward, especially for those unfamiliar with XML syntax, while also reducing the risk of errors associated with manual coding.
+
+To see the structure of a project configuration XML file and the definitions of the supported tag types and various parameters and variables, and to better understand how Visual Menu Builder maps and creates these elements when configuring your project, see [Project Configuration Overview](https://nlp.johnsnowlabs.com/docs/en/alab/tags_overview).
+
+**Key Features:**
+
+**Add New Element**
+
+The new menu user interface allows users to easily add new elements to their project configurations. Users can click on the plus icon ("+") within the Visual Menu Builder interface to add a new element. Once the element is added, users can further customize it by configuring additional parameters directly in the interface. This might include setting attributes, defining properties, or linking to other project components.
+
+
+
+**Edit an Element**
+
+Users can modify the properties and configurations of existing elements within the project. By clicking on the edit icon (a pencil icon), users can access the settings for an existing element. This opens an editable interface where users can adjust the element's parameters to suit the evolving needs of the project.
+
+
+
+**Delete an Element**
+
+Users can remove unwanted elements from the project configuration. Users can click on the cross button ("x") associated with a specific element to remove it from the project. This feature helps in keeping the project configuration clean and relevant by allowing users to easily remove elements that are no longer needed.
+
+
+
+**Drag and Move Element**
+
+The new visual menu builder allows users to easily rearrange elements within the project configuration using a drag-and-drop interface. To move an element, users can click and hold on the "Handle" icon, which is represented by a set of six dots (three parallel dots in two vertical rows) next to the element. After clicking on the Handle, users can drag the element to the desired position within the project configuration. Release the mouse button to drop the element in its new location. This feature provides flexibility in organizing the project structure, allowing users to quickly and intuitively reorder elements.
+
+
+
+**Show Element Boundaries**
+
+The **Show element Boundaries** button in the visual menu builder highlights the borders of each element within the project configuration, making it easier to visualize and distinguish the different components. By clicking on the "**Show element Boundaries**" button, users can toggle the visibility of the boundaries for all elements in the configuration. When enabled, a visible border will appear around each element, clearly outlining its scope and separation from other elements. This feature is particularly helpful when working with complex configurations where multiple elements are closely positioned. By showing the boundaries, users can easily identify and select the correct element they want to edit, move, or delete.
+
+
+
+**Show Parent Action Buttons on Hover**
+
+The **Show parent action buttons on hover** button in the Visual Menu Builder allows users to quickly access action buttons (such as edit, delete, or add) for parent elements by hovering over them. By hiding the action buttons until needed, it reduces visual clutter and allows users to concentrate on their current tasks. The ability to quickly access these buttons by hovering ensures that they remain easily accessible without overwhelming the interface.
+
+
+
+**Fullscreen Mode**
+
+The "**Fullscreen**" button in the visual menu builder allows users to expand the workspace to occupy the entire screen, providing a larger and more focused view of the project configuration. Clicking on the "**Fullscreen**" button maximizes the Visual Menu Builder, hiding other UI elements so the entire screen is dedicated to the project configuration. To exit fullscreen mode, users can click the "**Fullscreen**" button again or use the Esc key to return to the normal view with all standard UI elements visible.
+
+
+
\ No newline at end of file
diff --git a/docs/en/alab/synthetic_task.md b/docs/en/alab/synthetic_task.md
index f2df43964e..ca548ef7b7 100644
--- a/docs/en/alab/synthetic_task.md
+++ b/docs/en/alab/synthetic_task.md
@@ -3,7 +3,7 @@ layout: docs
comment: no
header: true
seotitle: Generative AI Lab | John Snow Labs
-title: Synthetic task generation
+title: Synthetic Task Generation
permalink: /docs/en/alab/synthetic_task
key: docs-training
modify_date: "2024-03-19"
@@ -15,40 +15,50 @@ sidebar:
-With Generative AI Lab 5.2, you can harness the potential of synthetic documents generated by LLMs such as ChatGPT. This integration allows you to easily create diverse and customizable synthetic text for your annotation tasks, enabling you to balance any entity skewness in your data and to train and evaluate your models more efficiently.
+Starting in Generative AI Lab 5.2, you can harness the potential of synthetic documents generated by LLMs such as ChatGPT. This integration allows you to easily create diverse and customizable synthetic text for your annotation tasks, enabling you to balance any entity skewness in your data and to train and evaluate your models more efficiently.
-Generative AI Lab offers seamless integration with ChatGPT, enabling on-the-fly text generation. Additionally, Generative AI Labs provides the flexibility to manage multiple service providers key pairs for robust and flexible integration. These service providers can be assigned to specific projects, simplifying resource management. During the integration process, Each Service Provider Key can be validated via the UI (User Interface), ensuring seamless integration.
+In Generative AI Lab 6.5.0, additional types of synthetic documents are supported: proportional and augmented.
-Once the service provider integration is completed, it can be utilized in projects that can benefit from the robust capabilities of this new integration. Text generation becomes straightforward and effortless. Provide a prompt adapted to your data needs (you can test it via the ChatGPT app and copy/paste it to Generative AI Lab when ready) to initiate the generation process and obtain the required tasks. Users can further control the results by setting the "Temperature" and the "Number of text to generate." The "Temperature" parameter governs the "creativity" or randomness of the LLM-generated text. Higher temperature values (e.g., 0.7) yield more diverse and creative outputs, whereas lower values (e.g., 0.2) produce more deterministic and focused outputs.
+This use of the feature depends on an LLM being configured.
-The Generative AI Lab integration delivers the generated text in a dedicated UI that allows users to review, edit, and tag it in place. After an initial verification and editing, the generated texts can be imported into the project as Tasks, serving as annotation tasks for model training. Additionally, the generated texts can be downloaded locally in CSV format, facilitating their reuse in other projects.
+Once the service provider integration is completed, it can be utilized in projects that can benefit from the robust capabilities of this new integration. Text generation becomes straightforward and effortless.
-Generative AI Labs will soon support integration with additional service providers, further empowering our users with more powerful capabilities for even more efficient and robust model generation.
-
-
-
-
+
### Generate synthetic tasks using Azure OpenAI
Azure OpenAI can also be used to generate synthetic tasks. Here's a quick guide:
**Setting up and Validating the New Service Provider:**
-1. From the task page, click on the "Import" button and navigate to the "Generate Synthetic Task" page.
-2. Provide an appropriate prompt in the "Write Prompt" text box and click on the settings icon located on the right side of the page.
-3. Enter the API endpoint URL and secret key, then click on "validate."
-4. After validating the connection, set the desired temperature and the number of tasks to generate.
-5. Click on the "Generate" button to create synthetic tasks.
+1. From the task page, click on the "Import" button and navigate to the "Generate Synthetic Task" page.
+2. Provide an appropriate prompt in the "Write Prompt" text box and click on the settings icon located on the right side of the page.
+3. Enter the API endpoint URL and secret key, then click on "validate."
+4. After validating the connection, set the desired temperature and the number of tasks to generate.
+5. Click on the "Generate" button to create synthetic tasks.

-
+
+
+For synthetic tasks, provide a prompt adapted to your data needs to initiate the generation process and obtain the required tasks. Users can further control the results by setting the "Temperature" and the "Number of text to generate." The "Temperature" parameter governs the "creativity" or randomness of the LLM-generated text. Higher temperature values (e.g., 0.7) yield more diverse and creative outputs, whereas lower values (e.g., 0.2) produce more deterministic and focused outputs.
+
+
+
+
+
+#### Proportional Augmentaiton
+
+This method enhances data quality by using various testing techniques to generate new data based on an existing dataset. Proportional Augmentation is particularly effective in improving model performance by addressing specific weaknesses, such as the inability to recognize lowercase text, uppercase text, typos, and more. It is especially beneficial for bias and robustness testing, ensuring that the model produces high-quality and accurate results for machine learning, predictive modeling, and decision-making tasks. After setting the test types and max_proportion, click on "**Generate Results**" to create augmented tasks. Based on your configuration, data augmentation will enhance the existing tasks and generate new ones.
+
+
-### By default "synthetic" tag is added for imported synthetic tasks
-In previous versions, users had to manually add tags to synthetically generated tasks or else tasks imported into the task page lacked any associated tags. Starting with version 6.5.0, when tasks are imported, they now come with synthetic tags already associated with them during import in the task page.
+Another way to generate augmented tasks is through "**Templatic augmentation**".
-
+#### Templatic Augementation
+Templatic Augmentation creates new data by using templates or patterns that are similar in structure and context to the original input. This method depends a lot on the templates provided by the user. There are two options for using this approach:
+
+ **A. Manually Add Templates**
+ Users can manually choose templates along with the available labels. They can choose how many results to generate for each template using a scroll bar, which can be set from 1 to 50.
-This improvement saves time by eliminating the need for manual tag assignment and ensures that imported tasks are accurately tagged from the start, improving organization and searchability. Also, this enhancement streamlines the workflow for managing and organizing synthetic tasks, making it easier to work with large datasets as well.
+
-
\ No newline at end of file