From 59d6f7f04e390fb13fcba62bf22cea6ff2030623 Mon Sep 17 00:00:00 2001
From: Ella Charlaix <80481427+echarlaix@users.noreply.github.com>
Date: Fri, 18 Oct 2024 15:59:22 +0200
Subject: [PATCH] Clean up ORT documentation (#2065)
* refactor ort doc
* fix links
* fix
---
.../package_reference/modeling_ort.mdx | 5 +
.../onnxruntime/usage_guides/models.mdx | 275 +++---------------
2 files changed, 48 insertions(+), 232 deletions(-)
diff --git a/docs/source/onnxruntime/package_reference/modeling_ort.mdx b/docs/source/onnxruntime/package_reference/modeling_ort.mdx
index 65b2b60195..2c93ab3ac0 100644
--- a/docs/source/onnxruntime/package_reference/modeling_ort.mdx
+++ b/docs/source/onnxruntime/package_reference/modeling_ort.mdx
@@ -119,6 +119,11 @@ The following ORT classes are available for the following custom tasks.
## Stable Diffusion
+#### ORTDiffusionPipeline
+
+[[autodoc]] onnxruntime.ORTDiffusionPipeline
+ - __call__
+
#### ORTStableDiffusionPipeline
[[autodoc]] onnxruntime.ORTStableDiffusionPipeline
diff --git a/docs/source/onnxruntime/usage_guides/models.mdx b/docs/source/onnxruntime/usage_guides/models.mdx
index 131822e956..1292e755c0 100644
--- a/docs/source/onnxruntime/usage_guides/models.mdx
+++ b/docs/source/onnxruntime/usage_guides/models.mdx
@@ -4,263 +4,74 @@ Optimum is a utility package for building and running inference with accelerated
Optimum can be used to load optimized models from the [Hugging Face Hub](hf.co/models) and create pipelines
to run accelerated inference without rewriting your APIs.
-## Switching from Transformers to Optimum
-The `optimum.onnxruntime.ORTModelForXXX` model classes are API compatible with Hugging Face Transformers models. This
-means you can just replace your `AutoModelForXXX` class with the corresponding `ORTModelForXXX` class in `optimum.onnxruntime`.
+## Loading
-You do not need to adapt your code to get it to work with `ORTModelForXXX` classes:
+### Transformers models
-```diff
-from transformers import AutoTokenizer, pipeline
--from transformers import AutoModelForQuestionAnswering
-+from optimum.onnxruntime import ORTModelForQuestionAnswering
-
--model = AutoModelForQuestionAnswering.from_pretrained("deepset/roberta-base-squad2") # PyTorch checkpoint
-+model = ORTModelForQuestionAnswering.from_pretrained("optimum/roberta-base-squad2") # ONNX checkpoint
-tokenizer = AutoTokenizer.from_pretrained("deepset/roberta-base-squad2")
-
-onnx_qa = pipeline("question-answering",model=model,tokenizer=tokenizer)
-
-question = "What's my name?"
-context = "My name is Philipp and I live in Nuremberg."
-pred = onnx_qa(question, context)
-```
-
-### Loading a vanilla Transformers model
-
-Because the model you want to work with might not be already converted to ONNX, [`~optimum.onnxruntime.ORTModel`]
-includes a method to convert vanilla Transformers models to ONNX ones. Simply pass `export=True` to the
-[`~optimum.onnxruntime.ORTModel.from_pretrained`] method, and your model will be loaded and converted to ONNX on-the-fly:
-
-```python
->>> from optimum.onnxruntime import ORTModelForSequenceClassification
-
->>> # Load the model from the hub and export it to the ONNX format
->>> model = ORTModelForSequenceClassification.from_pretrained(
-... "distilbert-base-uncased-finetuned-sst-2-english", export=True
-... )
-```
-
-### Pushing ONNX models to the Hugging Face Hub
-
-It is also possible, just as with regular [`~transformers.PreTrainedModel`]s, to push your `ORTModelForXXX` to the
-[Hugging Face Model Hub](https://hf.co/models):
-
-```python
->>> from optimum.onnxruntime import ORTModelForSequenceClassification
-
->>> # Load the model from the hub and export it to the ONNX format
->>> model = ORTModelForSequenceClassification.from_pretrained(
-... "distilbert-base-uncased-finetuned-sst-2-english", export=True
-... )
-
->>> # Save the converted model
->>> model.save_pretrained("a_local_path_for_convert_onnx_model")
-
-# Push the onnx model to HF Hub
->>> model.push_to_hub( # doctest: +SKIP
-... "a_local_path_for_convert_onnx_model", repository_id="my-onnx-repo", use_auth_token=True
-... )
-```
-
-## Sequence-to-sequence models
-
-Sequence-to-sequence (Seq2Seq) models can also be used when running inference with ONNX Runtime. When Seq2Seq models
-are exported to the ONNX format, they are decomposed into three parts that are later combined during inference:
-- The encoder part of the model
-- The decoder part of the model + the language modeling head
-- The same decoder part of the model + language modeling head but taking and using pre-computed key / values as inputs and
-outputs. This makes inference faster.
-
-Here is an example of how you can load a T5 model to the ONNX format and run inference for a translation task:
-
-
-```python
->>> from transformers import AutoTokenizer, pipeline
->>> from optimum.onnxruntime import ORTModelForSeq2SeqLM
-
-# Load the model from the hub and export it to the ONNX format
->>> model_name = "t5-small"
->>> model = ORTModelForSeq2SeqLM.from_pretrained(model_name, export=True)
->>> tokenizer = AutoTokenizer.from_pretrained(model_name)
-
-# Create a pipeline
->>> onnx_translation = pipeline("translation_en_to_fr", model=model, tokenizer=tokenizer)
->>> text = "He never went out without a book under his arm, and he often came back with two."
->>> result = onnx_translation(text)
->>> # [{'translation_text': "Il n'est jamais sorti sans un livre sous son bras, et il est souvent revenu avec deux."}]
-```
-
-## Stable Diffusion
-
-Stable Diffusion models can also be used when running inference with ONNX Runtime. When Stable Diffusion models
-are exported to the ONNX format, they are split into four components that are later combined during inference:
-- The text encoder
-- The U-NET
-- The VAE encoder
-- The VAE decoder
-
-Make sure you have 🤗 Diffusers installed.
-
-To install `diffusers`:
-```bash
-pip install diffusers
-```
-
-### Text-to-Image
-
-Here is an example of how you can load an ONNX Stable Diffusion model and run inference using ONNX Runtime:
-
-```python
-from optimum.onnxruntime import ORTStableDiffusionPipeline
-
-model_id = "runwayml/stable-diffusion-v1-5"
-pipeline = ORTStableDiffusionPipeline.from_pretrained(model_id, revision="onnx")
-prompt = "sailing ship in storm by Leonardo da Vinci"
-image = pipeline(prompt).images[0]
-```
+Once your model was [exported to the ONNX format](https://huggingface.co/docs/optimum/exporters/onnx/usage_guides/export_a_model), you can load it by replacing the `AutoModelForXxx` class with the corresponding `ORTModelForXxx`.
-To load your PyTorch model and convert it to ONNX on-the-fly, you can set `export=True`.
-
-```python
-pipeline = ORTStableDiffusionPipeline.from_pretrained(model_id, export=True)
-
-# Don't forget to save the ONNX model
-save_directory = "a_local_path"
-pipeline.save_pretrained(save_directory)
-```
-
-
-
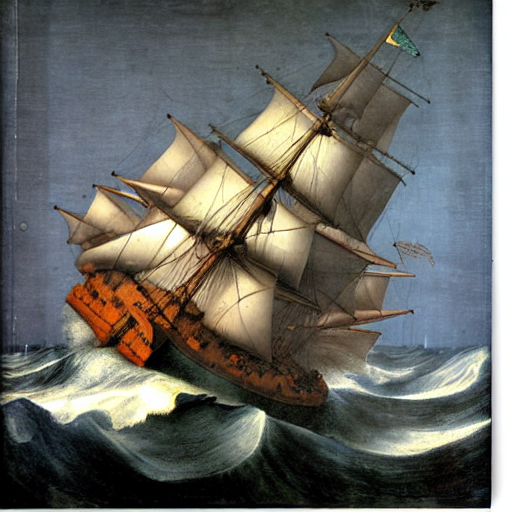
-
-
-### Image-to-Image
-
-```python
-import requests
-import torch
-from PIL import Image
-from io import BytesIO
-from optimum.onnxruntime import ORTStableDiffusionImg2ImgPipeline
-
-model_id = "runwayml/stable-diffusion-v1-5"
-pipeline = ORTStableDiffusionImg2ImgPipeline.from_pretrained(model_id, revision="onnx")
-
-url = "https://raw.githubusercontent.com/CompVis/stable-diffusion/main/assets/stable-samples/img2img/sketch-mountains-input.jpg"
-
-response = requests.get(url)
-init_image = Image.open(BytesIO(response.content)).convert("RGB")
-init_image = init_image.resize((768, 512))
-
-prompt = "A fantasy landscape, trending on artstation"
-
-image = pipeline(prompt=prompt, image=init_image, strength=0.75, guidance_scale=7.5).images[0]
-image.save("fantasy_landscape.png")
-```
-
-### Inpaint
-
-```python
-import PIL
-import requests
-import torch
-from io import BytesIO
-from optimum.onnxruntime import ORTStableDiffusionInpaintPipeline
-
-model_id = "runwayml/stable-diffusion-inpainting"
-pipeline = ORTStableDiffusionInpaintPipeline.from_pretrained(model_id, revision="onnx")
-
-def download_image(url):
- response = requests.get(url)
- return PIL.Image.open(BytesIO(response.content)).convert("RGB")
-
-img_url = "https://raw.githubusercontent.com/CompVis/latent-diffusion/main/data/inpainting_examples/overture-creations-5sI6fQgYIuo.png"
-mask_url = "https://raw.githubusercontent.com/CompVis/latent-diffusion/main/data/inpainting_examples/overture-creations-5sI6fQgYIuo_mask.png"
+```diff
+ from transformers import AutoTokenizer, pipeline
+- from transformers import AutoModelForQuestionAnswering
++ from optimum.onnxruntime import ORTModelForQuestionAnswering
-init_image = download_image(img_url).resize((512, 512))
-mask_image = download_image(mask_url).resize((512, 512))
+- model = AutoModelForQuestionAnswering.from_pretrained("meta-llama/Llama-3.2-1B) # PyTorch checkpoint
++ model = ORTModelForQuestionAnswering.from_pretrained("onnx-community/Llama-3.2-1B", subfolder="onnx") # ONNX checkpoint
+ tokenizer = AutoTokenizer.from_pretrained("meta-llama/Llama-3.2-1B")
-prompt = "Face of a yellow cat, high resolution, sitting on a park bench"
-image = pipeline(prompt=prompt, image=init_image, mask_image=mask_image).images[0]
+ pipe = pipeline("text-generation", model=model, tokenizer=tokenizer)
+ result = pipe("He never went out without a book under his arm")
```
+More information for all the supported `ORTModelForXxx` in our [documentation](https://huggingface.co/docs/optimum/onnxruntime/package_reference/modeling_ort)
-## Stable Diffusion XL
-
-Before using `ORTStableDiffusionXLPipeline` make sure to have `diffusers` and `invisible_watermark` installed. You can install the libraries as follows:
-```bash
-pip install diffusers
-pip install invisible-watermark>=0.2.0
-```
-
-### Text-to-Image
-
-Here is an example of how you can load a SDXL ONNX model from [stabilityai/stable-diffusion-xl-base-1.0](https://huggingface.co/stabilityai/stable-diffusion-xl-base-1.0) and run inference using ONNX Runtime :
+### Diffusers models
-```python
-from optimum.onnxruntime import ORTStableDiffusionXLPipeline
+Once your model was [exported to the ONNX format](https://huggingface.co/docs/optimum/exporters/onnx/usage_guides/export_a_model), you can load it by replacing the `DiffusionPipeline` class with the corresponding `ORTDiffusionPipeline`.
-model_id = "stabilityai/stable-diffusion-xl-base-1.0"
-base = ORTStableDiffusionXLPipeline.from_pretrained(model_id)
-prompt = "sailing ship in storm by Leonardo da Vinci"
-image = base(prompt).images[0]
-# Don't forget to save the ONNX model
-save_directory = "sd_xl_base"
-base.save_pretrained(save_directory)
+```diff
+- from diffusers import DiffusionPipeline
++ from optimum.onnxruntime import ORTDiffusionPipeline
+
+ model_id = "runwayml/stable-diffusion-v1-5"
+- pipeline = DiffusionPipeline.from_pretrained(model_id)
++ pipeline = ORTDiffusionPipeline.from_pretrained(model_id, revision="onnx")
+ prompt = "sailing ship in storm by Leonardo da Vinci"
+ image = pipeline(prompt).images[0]
```
+## Converting your model to ONNX on-the-fly
-### Image-to-Image
-
-Here is an example of how you can load a PyTorch SDXL model, convert it to ONNX on-the-fly and run inference using ONNX Runtime for *image-to-image* :
+In case your model wasn't already [converted to ONNX](https://huggingface.co/docs/optimum/exporters/onnx/usage_guides/export_a_model), [`~optimum.onnxruntime.ORTModel`] includes a method to convert your model to ONNX on-the-fly.
+Simply pass `export=True` to the [`~optimum.onnxruntime.ORTModel.from_pretrained`] method, and your model will be loaded and converted to ONNX on-the-fly:
```python
-from optimum.onnxruntime import ORTStableDiffusionXLImg2ImgPipeline
-from diffusers.utils import load_image
-
-model_id = "stabilityai/stable-diffusion-xl-refiner-1.0"
-pipeline = ORTStableDiffusionXLImg2ImgPipeline.from_pretrained(model_id, export=True)
+>>> from optimum.onnxruntime import ORTModelForSequenceClassification
-url = "https://huggingface.co/datasets/optimum/documentation-images/resolve/main/intel/openvino/sd_xl/castle_friedrich.png"
-image = load_image(url).convert("RGB")
-prompt = "medieval castle by Caspar David Friedrich"
-image = pipeline(prompt, image=image).images[0]
-image.save("medieval_castle.png")
+>>> # Load the model from the hub and export it to the ONNX format
+>>> model_id = "distilbert-base-uncased-finetuned-sst-2-english"
+>>> model = ORTModelForSequenceClassification.from_pretrained(model_id, export=True)
```
-### Refining the image output
-
-The image can be refined by making use of a model like [stabilityai/stable-diffusion-xl-refiner-1.0](https://huggingface.co/stabilityai/stable-diffusion-xl-refiner-1.0). In this case, you only have to output the latents from the base model.
+## Pushing your model to the Hub
+You can also call `push_to_hub` directly on your model to upload it to the [Hub](https://hf.co/models).
```python
-from optimum.onnxruntime import ORTStableDiffusionXLImg2ImgPipeline
-
-model_id = "stabilityai/stable-diffusion-xl-refiner-1.0"
-refiner = ORTStableDiffusionXLImg2ImgPipeline.from_pretrained(model_id, export=True)
-
-image = base(prompt=prompt, output_type="latent").images[0]
-image = refiner(prompt=prompt, image=image[None, :]).images[0]
-image.save("sailing_ship.png")
-```
-
-
-
-## Latent Consistency Models
-
-### Text-to-Image
+>>> from optimum.onnxruntime import ORTModelForSequenceClassification
-Here is an example of how you can load a Latent Consistency Models (LCMs) from [SimianLuo/LCM_Dreamshaper_v7](https://huggingface.co/SimianLuo/LCM_Dreamshaper_v7) and run inference using ONNX Runtime :
+>>> # Load the model from the hub and export it to the ONNX format
+>>> model_id = "distilbert-base-uncased-finetuned-sst-2-english"
+>>> model = ORTModelForSequenceClassification.from_pretrained(model_id, export=True)
-```python
-from optimum.onnxruntime import ORTLatentConsistencyModelPipeline
+>>> # Save the converted model locally
+>>> output_dir = "a_local_path_for_convert_onnx_model"
+>>> model.save_pretrained(output_dir)
-model_id = "SimianLuo/LCM_Dreamshaper_v7"
-pipeline = ORTLatentConsistencyModelPipeline.from_pretrained(model_id, export=True)
-prompt = "sailing ship in storm by Leonardo da Vinci"
-images = pipeline(prompt, num_inference_steps=4, guidance_scale=8.0).images
-```
+# Push the onnx model to HF Hub
+>>> model.push_to_hub(output_dir, repository_id="my-onnx-repo") # doctest: +SKIP
+```
\ No newline at end of file