- 大模型读文献之腾讯元宝--深度阅读 + 蛋白互作网络PPI分析流程示例
@@ -423,11 +432,10 @@
- 简介
-腾讯元宝的深度阅读模式是一种创新的阅读方式,它利用人工智能技术,为用户提供了一种全新的长文阅读体验。这种模式不仅能够处理大量文本,还能生成图文并茂的内容,极大地提升了阅读效率和理解深度。
+ 蛋白互作网络(PPI, Protein-Protein Interaction Networks)可以查看基因之间的联系,进而锁定关键基因。一般使用STRING数据库获取蛋白互作信息。
@@ -458,7 +466,7 @@
-
+
@@ -476,7 +484,7 @@
- 蛋白互作网络PPI分析流程示例
+ 大模型读文献之腾讯元宝--深度阅读
@@ -529,10 +537,11 @@
- 蛋白互作网络(PPI, Protein-Protein Interaction Networks)可以查看基因之间的联系,进而锁定关键基因。一般使用STRING数据库获取蛋白互作信息。
+ 简介
+腾讯元宝的深度阅读模式是一种创新的阅读方式,它利用人工智能技术,为用户提供了一种全新的长文阅读体验。这种模式不仅能够处理大量文本,还能生成图文并茂的内容,极大地提升了阅读效率和理解深度。
diff --git a/page/2/index.html b/page/2/index.html
index ad1aa3da9..ea201e488 100644
--- a/page/2/index.html
+++ b/page/2/index.html
@@ -179,12 +179,21 @@ 损不足
+
+
+
+
+
+
+
diff --git a/page/3/index.html b/page/3/index.html
index 5b29d5e74..9137e04ce 100644
--- a/page/3/index.html
+++ b/page/3/index.html
@@ -179,12 +179,21 @@ 损不足
+
+
+
+
+
+
+
diff --git a/page/4/index.html b/page/4/index.html
index aa1c1d599..778bc5feb 100644
--- a/page/4/index.html
+++ b/page/4/index.html
@@ -179,12 +179,21 @@ 损不足
+
+
+
+
+
+
+
diff --git a/search.xml b/search.xml
index a27348b0a..98ca88462 100644
--- a/search.xml
+++ b/search.xml
@@ -399,97 +399,6 @@
简介
-
- ImageJ--自动将源图或堆栈与目标图配准--StackReg插件
- /2024/05/23/109a1e8436e7/
-
-简介
-StackReg,用于递归对齐图像堆栈(stack)的 ImageJ 插件。
作者: Philippe Thévenaz, Biomedical Imaging Group, Swiss Federal Institute of Technology Lausanne
-
-该插件的目的是配准(换句话说,对齐或匹配)图像堆栈。每张图片用作下一张图片对齐的模板,以便通过转换进行对齐。当插件启动时,当前图片充当全局锚点。StackReg 插件要求安装有TurboReg 插件。该插件(StackReg)是TurboReg 插件的前端。这些插件之间有几种数据交换机制,其中之一是将临时文件写入 ImageJ 定义的临时目录。该目录的位置可以通过宏命令 "print(getDirectory("temp"));
"查看。文件名称是:StackRegSource,StackRegSourceR,StackRegSourceG,StackRegSourceB,StackRegTarget。
-配准完成后,原始堆栈将被销毁,取而代之的是配准结果。堆栈的名称和类型保持不变;除 RGB 堆栈和 HSB 堆栈外,所有数据类型均可使用。(请注意,RGB-stack 是由三个颜色分量组成的堆栈,不应与 RGB 彩色图像堆栈混淆:后者可以使用,而前者不能使用。如有疑问,请尝试一下)。如果提供的是彩色图像堆栈,则会在灰度版本上进行配准,灰度版本是由红色、绿色和蓝色分量加权求和而成的,即使堆栈是伪彩色图像也是如此。权重取决于整个图像堆栈,并通过主成分分析确定,以提供最大可能的对比度。一旦根据中间灰度表示确定了几何图形,就可以重建真正的彩色图像,如果需要生成伪彩色图像,还可以重新量化。
-
-示例
-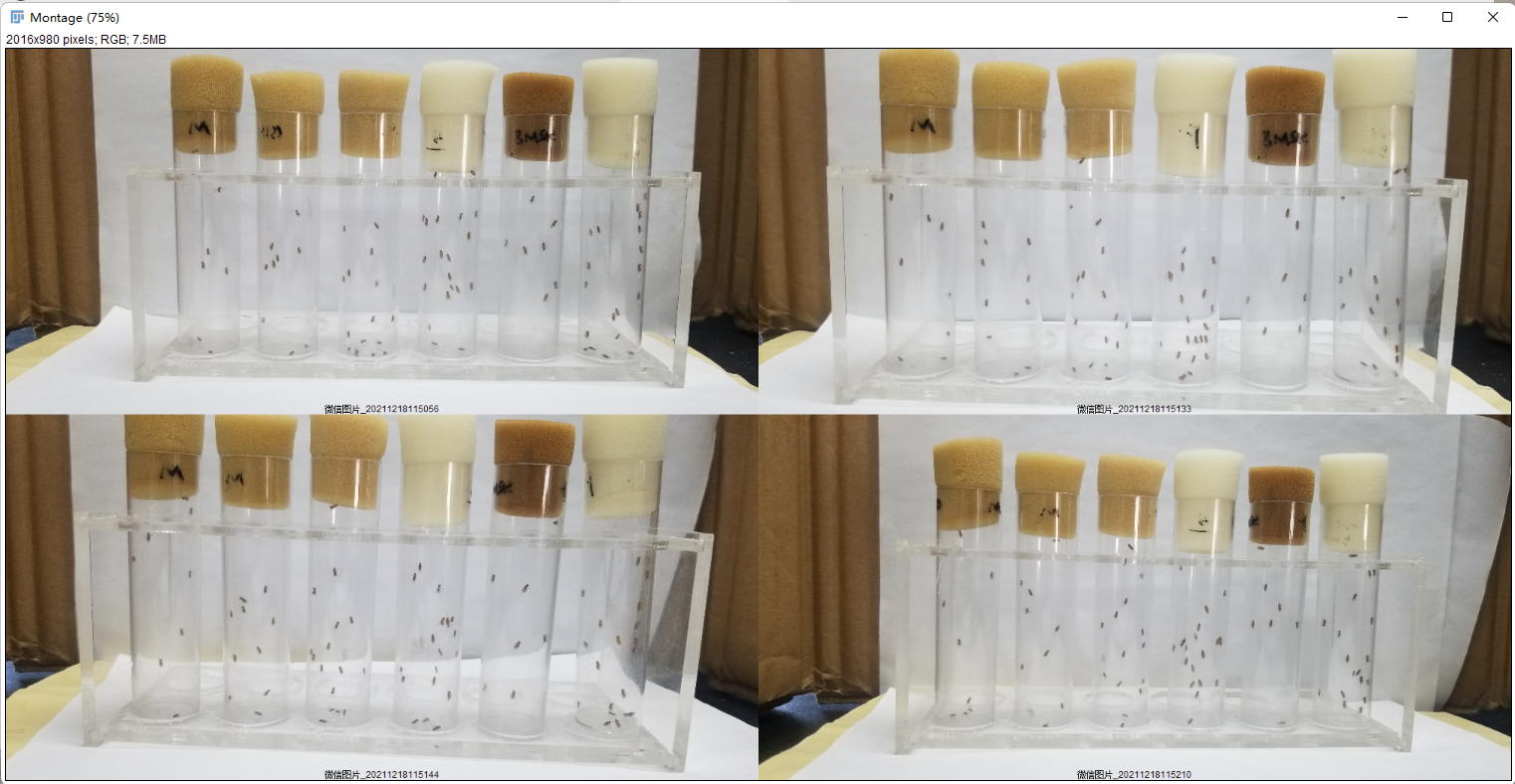
原始图
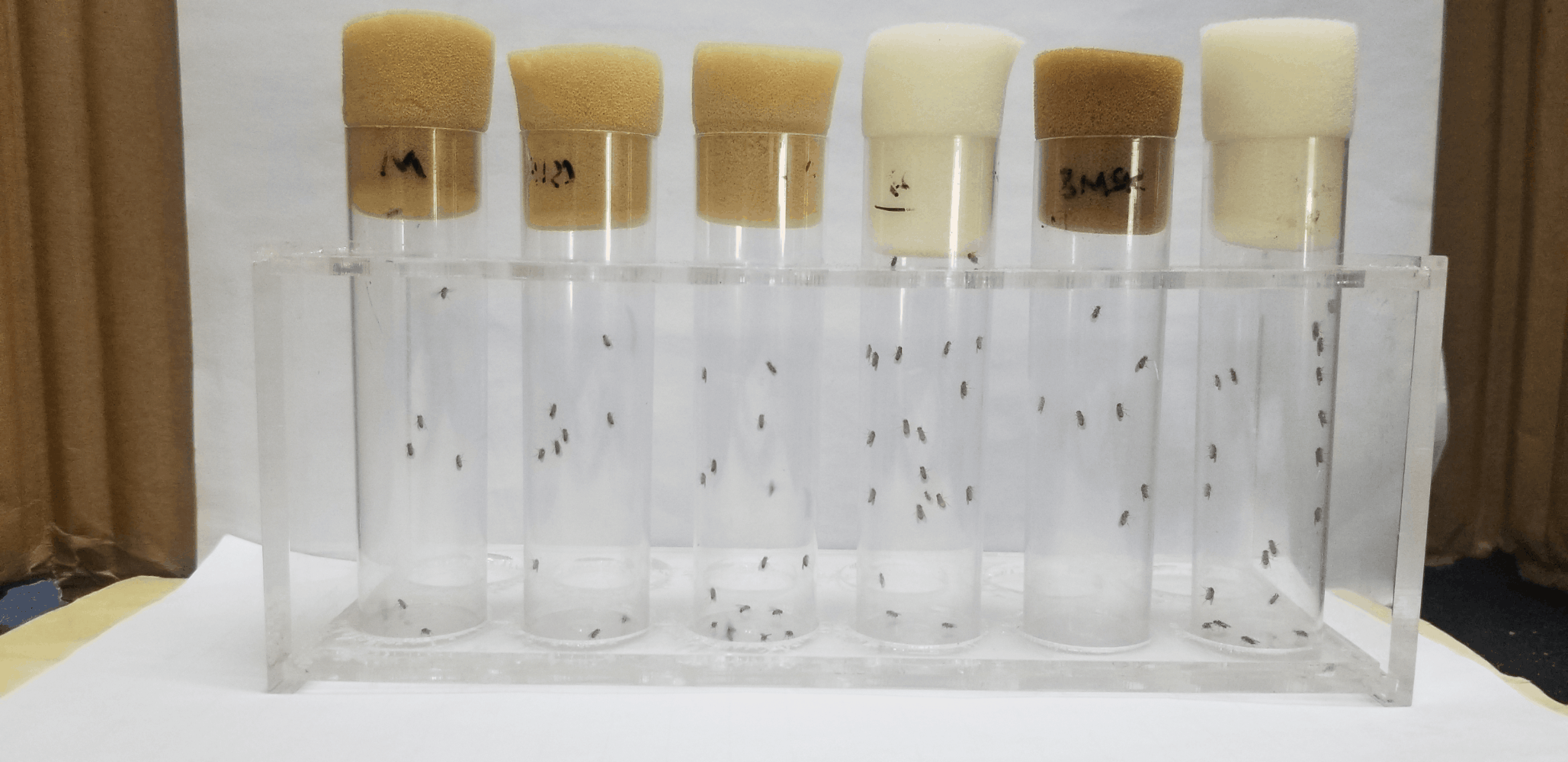
配准后(转换类型Scaled rotation)
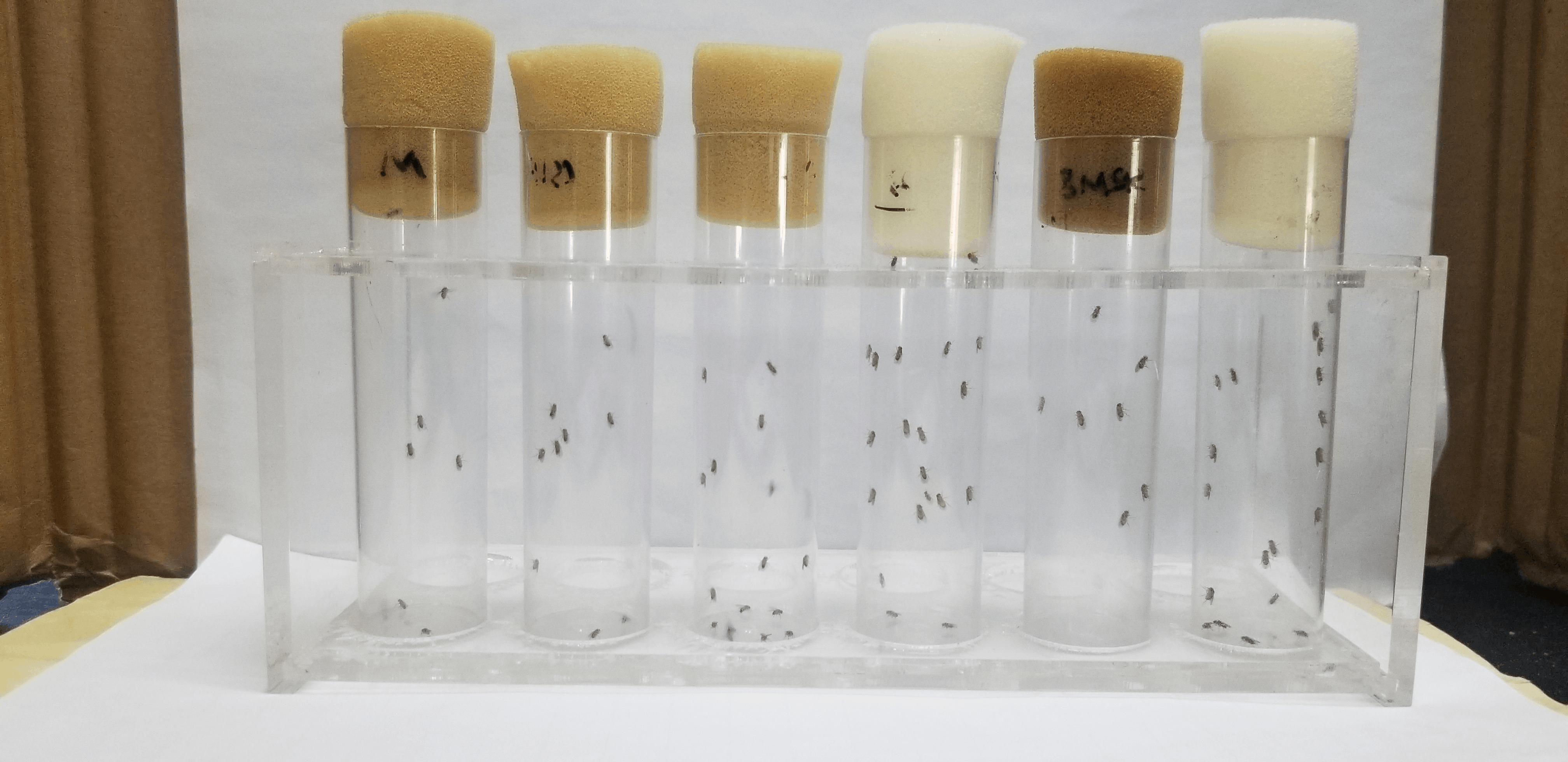
-
-参数
-有四种类型的转换:
-
--
-
Translation:
-
-
-映射公式为
。其中,输入坐标 u 映射到输出坐标 x,常数矢量 Δu 表示平移量。
-
--
-
Rigid Body:
-
-
-映射公式为

由于存在 Δu 项,因此除了旋转角度 θ 外,还考虑了平移。
-
--
-
Scaled rotation:
-
-
-映射公式为

与Rigid Body 的区别在于存在标量调整因子 λ。
-
--
-
Affine:
-
-
-映射公式为

由于四个系数
、
、
和
是独立的,因此仿射变换比按比例旋转具有更多的自由度。
-
-操作步骤
-使用的Fiji ImageJ
-
--
-
拖动图片文件夹到Fiji,默认为堆栈。
-
-
-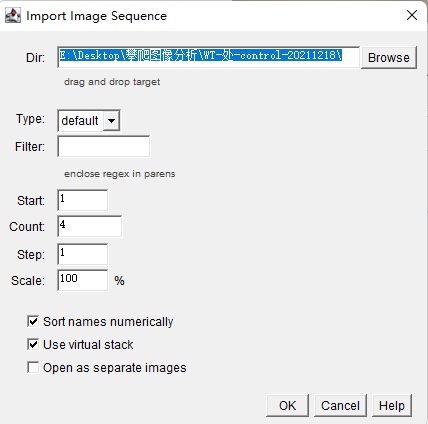
-
--
-
插件启动前,选择合适的图片,当前图片会被充当全局锚点。
-
--
-
打开StackReg。
-
-
-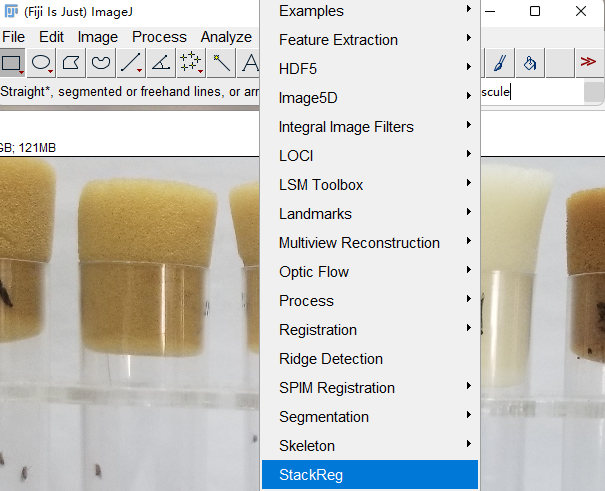
-
--
-
选择对齐方式,点击确定按钮。
-
-
-
-配准结果展示
-Translation
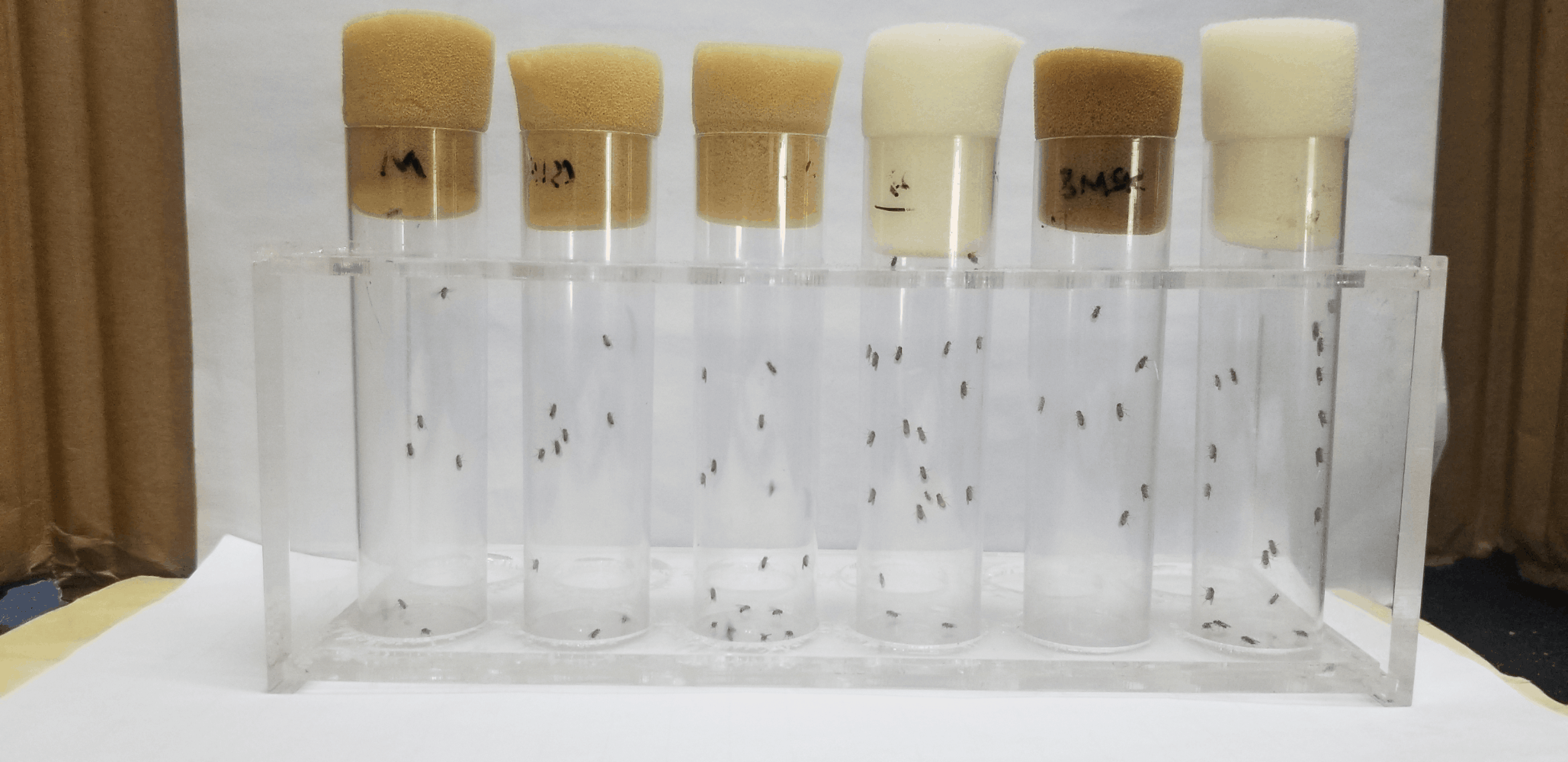
Rigid Body
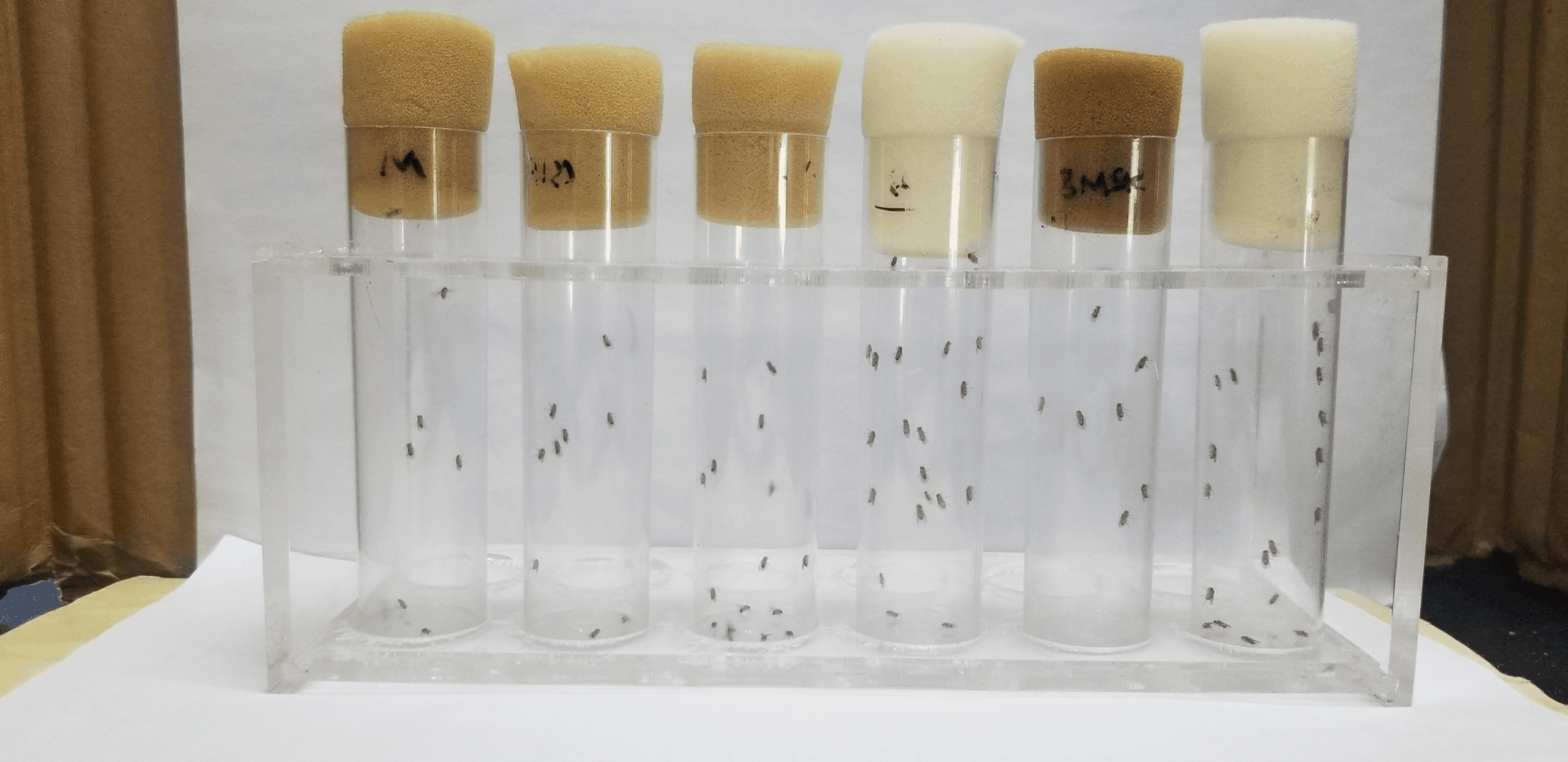
Scaled rotation
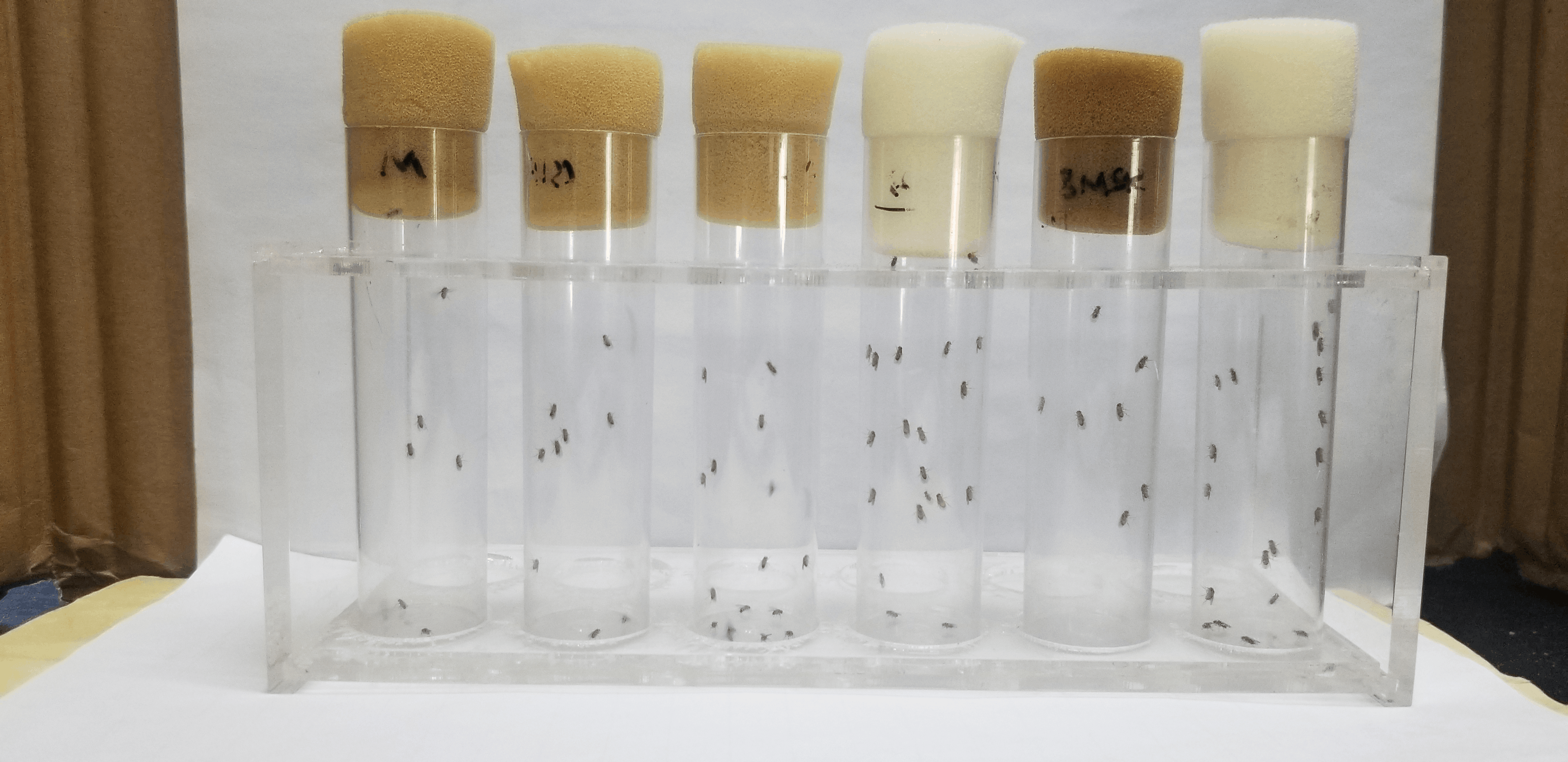
Affine
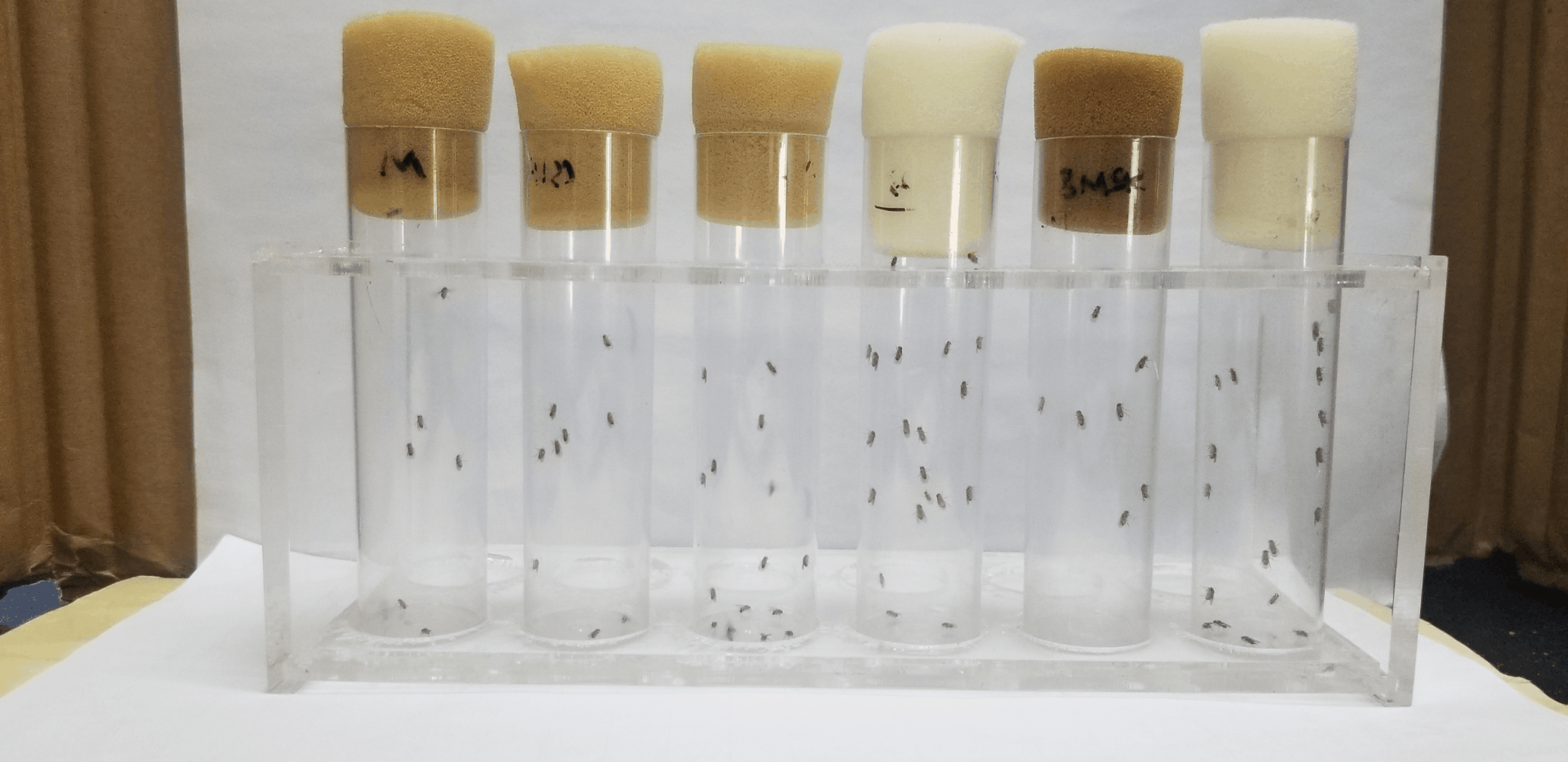
-
-脚本
-StackReg 是可编写脚本和使用宏记录的。下面是一个从宏调用 StackReg 的示例:
-run("StackReg ", "transformation=[Rigid Body]");
-
-参考
-
--
-
https://bigwww.epfl.ch/thevenaz/stackreg/
-
--
-
P. Thévenaz, U.E. Ruttimann, M. Unser, “A Pyramid Approach to Subpixel Registration Based on Intensity,” IEEE Transactions on Image Processing, vol. 7, no. 1, pp. 27-41, January 1998. Other relevant on-line publications are available at http://bigwww.epfl.ch/publications/.
-
-
-]]>
-
- ImageJ
-
-
- 笔记
- ImageJ
- 图像
- 插件
-
-
RNA-Seq Count数据的标准化(RPKM, FPKM, TPM)-学习笔记
/2023/09/21/8308e10eb1be/
@@ -615,22 +524,130 @@
- R语言--果蝇攀爬--ImageJ测量数据处理
- /2024/07/03/edfd8de9558b/
- 目的
-处理ImageJ测量的果蝇攀爬数据
-
-实例
-library(cluster)
# 设定工作目录
setwd("E:/Desktop/")
# 导入数据
X20180609_WF_IF <- read.csv("E:/Desktop/20180609-WF-IF.csv")
X20180609_CM_AM <- read.csv("E:/Desktop/20180609-CM-AM.csv")
X20180609_CF_AF <- read.csv("E:/Desktop/20180609-CF-AF.csv")
clusGap(X20180609_WF_IF$X[which(X20180609_WF_IF$Slice==1)],FUN=kmeans,nstart=25,K.max=10,B=500)
## 自定义函数,获取每管果蝇的攀爬指数----
ppindex <- function(X){
g1 <- c()
g2 <- c()
g3 <- c()
g4 <- c()
g5 <- c()
g6 <- c()
for (i in 1:max(X$Slice)) {
slice_x <- X$X[which(X$Slice==i)]
slice_y <- X$Y[which(X$Slice==i)]
slice_max <- (max(slice_x)+100)/6
par(mfrow = c(1,6))
# 管1
slice_x <- slice_x[which(slice_x < slice_max)]
slice_y <- slice_y[which(slice_x < slice_max)]
plot(slice_x,slice_y)
slice_y_fq0 <- length(slice_y[which(slice_y < 200)])
slice_y_fq1 <- length(slice_y[which(slice_y > 200&slice_y < 400)])
slice_y_fq2 <- length(slice_y[which(slice_y > 400)])-2
index_1 <- (slice_y_fq0*0+slice_y_fq1*1+slice_y_fq2*2)/(slice_y_fq0+slice_y_fq1+slice_y_fq2)
g1 <- c(g1,index_1)
# 管2
slice_x2 <- slice_x[which(slice_max < slice_x&slice_x < slice_max*2)]
slice_y2 <- slice_y[which(slice_max < slice_x&slice_x < slice_max*2)]
plot(slice_x2,slice_y2)
slice_y2_fq0 <- length(slice_y2[which(slice_y2 < 200)])
slice_y2_fq1 <- length(slice_y2[which(slice_y2 > 200&slice_y2 < 400)])
slice_y2_fq2 <- length(slice_y2[which(slice_y2 > 400)])-2
index_2 <- (slice_y2_fq0*0+slice_y2_fq1*1+slice_y2_fq2*2)/(slice_y2_fq0+slice_y2_fq1+slice_y2_fq2)
g2 <- c(g2,index_2)
# 管3
slice_x3 <- slice_x[which(slice_max*2 < slice_x&slice_x < slice_max*3)]
slice_y3 <- slice_y[which(slice_max*2 < slice_x&slice_x < slice_max*3)]
plot(slice_x3,slice_y3)
slice_y3_fq0 <- length(slice_y3[which(slice_y3 < 200)])
slice_y3_fq1 <- length(slice_y3[which(slice_y3 > 200&slice_y3 < 400)])
slice_y3_fq2 <- length(slice_y3[which(slice_y3 > 400)])-2
index_3 <- (slice_y3_fq0*0+slice_y3_fq1*1+slice_y3_fq2*2)/(slice_y3_fq0+slice_y3_fq1+slice_y3_fq2)
g3 <- c(g3,index_3)
# 管4
slice_x4 <- slice_x[which(slice_max*3 < slice_x&slice_x < slice_max*4)]
slice_y4 <- slice_y[which(slice_max*3 < slice_x&slice_x < slice_max*4)]
plot(slice_x4,slice_y4)
slice_y4_fq0 <- length(slice_y4[which(slice_y4 < 200)])
slice_y4_fq1 <- length(slice_y4[which(slice_y4 > 200&slice_y4 < 400)])
slice_y4_fq2 <- length(slice_y4[which(slice_y4 > 400)])-2
index_4 <- (slice_y4_fq0*0+slice_y4_fq1*1+slice_y4_fq2*2)/(slice_y4_fq0+slice_y4_fq1+slice_y4_fq2)
g4 <- c(g4,index_4)
# 管5
slice_x5 <- slice_x[which(slice_max*4 < slice_x&slice_x < slice_max*5)]
slice_y5 <- slice_y[which(slice_max*4 < slice_x&slice_x < slice_max*5)]
plot(slice_x5,slice_y5)
slice_y5_fq0 <- length(slice_y5[which(slice_y5 < 200)])
slice_y5_fq1 <- length(slice_y5[which(slice_y5 > 200&slice_y5 < 400)])
slice_y5_fq2 <- length(slice_y5[which(slice_y5 > 400)])-2
index_5 <- (slice_y5_fq0*0+slice_y5_fq1*1+slice_y5_fq2*2)/(slice_y5_fq0+slice_y5_fq1+slice_y5_fq2)
g5 <- c(g5,index_5)
# 管6
slice_x6 <- slice_x[which(slice_max*5 < slice_x&slice_x < slice_max*6)]
slice_y6 <- slice_y[which(slice_max*5 < slice_x&slice_x < slice_max*6)]
plot(slice_x6,slice_y6)
slice_y6_fq0 <- length(slice_y6[which(slice_y6 < 200)])
slice_y6_fq1 <- length(slice_y6[which(slice_y6 > 200&slice_y6 < 400)])
slice_y6_fq2 <- length(slice_y6[which(slice_y6 > 400)])-2
index_6 <- (slice_y6_fq0*0+slice_y6_fq1*1+slice_y6_fq2*2)/(slice_y6_fq0+slice_y6_fq1+slice_y6_fq2)
g6 <- c(g6,index_6)
}
flyindex <- data.frame(g1,g2,g3,g4,g5,g6)
return(flyindex)
}
#汇总----
WI_F_Index <- ppindex(X20180609_WF_IF)
names(WI_F_Index) <- c("WF1","WF2","WF3","IF1","IF2","IF3")
WI_F_Index <- ppindex(X20180609_CF_AF)
names(CA_F_Index) <- c("CF1","CF2","CF3","AF1","AF2","AF3")
CA_M_Index <- ppindex(X20180609_CM_AM)
names(CA_M_Index) <- c("CM1","CM2","CM3","AM1","AM2","AM3")
# 保存
library(xlsx)
write.xlsx2(WI_F_Index, sheetName = "WI_F_Index", file='flyIndex.xlsx',append = TRUE)
write.xlsx2(CA_F_Index, sheetName = "CA_F_Index", file='flyIndex.xlsx',append = TRUE)
write.xlsx2(CA_M_Index, sheetName = "CA_M_Index", file='flyIndex.xlsx',append = TRUE)
dev.off()
###########################################################################################
#单管----
# 导入数据
X20180609_IM3 <- read.csv("E:/Desktop/20180609-IM3.csv")
X20180609_IM2 <- read.csv("E:/Desktop/20180609-IM2.csv")
X20180609_IM1 <- read.csv("E:/Desktop/20180609-IM1.csv")
X20180609_WM3 <- read.csv("E:/Desktop/20180609-WM3.csv")
X20180609_WM2 <- read.csv("E:/Desktop/20180609-WM2.csv")
X20180609_WM1 <- read.csv("E:/Desktop/20180609-WM1.csv")
pp2index <- function(x) {
index <- c()
for (i in 1:max(x$Slice)) {
slice_x <- x$X[which(x$Slice == i)]
slice_y <- x$Y[which(x$Slice == i)]
slice_y_fq0 <- length(slice_y[which(slice_y < 340)])
slice_y_fq1 <- length(slice_y[which(slice_y > 340 &
slice_y < 600)])
if ((length(slice_y[which(slice_y > 600 &
slice_y < 900)]) - 1) < 0) {
slice_y_fq2 <- 0
} else {
slice_y_fq2 <- length(slice_y[which(slice_y > 600 &
slice_y < 900)]) - 1
}
if ((length(slice_y[which(slice_y > 900 &
slice_y < 1200)]) - 1) < 0) {
slice_y_fq3 <- 0
} else {
slice_y_fq3 <- length(slice_y[which(slice_y > 900 &
slice_y < 1200)]) - 1
}
if ((length(slice_y[which(slice_y > 1200)]) - 1) < 0) {
slice_y_fq4 <- 0
} else {
slice_y_fq4 <- length(slice_y[which(slice_y > 1200)]) - 1
}
index_1 <- (slice_y_fq0 * 0 + slice_y_fq1 * 1 + slice_y_fq2 * 2 + slice_y_fq3 *
3 + slice_y_fq4 * 4) / (slice_y_fq0 + slice_y_fq1 + slice_y_fq2 + slice_y_fq3 +
slice_y_fq4)
index <- c(index,index_1)
}
return(index)
}
IM3 <- pp2index(X20180609_IM3)
IM2 <- pp2index(X20180609_IM2)
IM1 <- pp2index(X20180609_IM1)
WM1 <- pp2index(X20180609_WM1)
WM2 <- pp2index(X20180609_WM3)
WM3 <- pp2index(X20180609_WM2)
WI_M_Index <- cbind(WM1=WM1,WM2=WM2,WM3=WM3,IM1=IM1,IM2=IM2,IM3=IM3)
write.xlsx2(WI_M_Index, sheetName = "WI_M_Index", file='flyIndex.xlsx',append = TRUE)
]]>
+ ImageJ--自动将源图或堆栈与目标图配准--StackReg插件
+ /2024/05/23/109a1e8436e7/
+
+简介
+StackReg,用于递归对齐图像堆栈(stack)的 ImageJ 插件。
作者: Philippe Thévenaz, Biomedical Imaging Group, Swiss Federal Institute of Technology Lausanne
+
+该插件的目的是配准(换句话说,对齐或匹配)图像堆栈。每张图片用作下一张图片对齐的模板,以便通过转换进行对齐。当插件启动时,当前图片充当全局锚点。StackReg 插件要求安装有TurboReg 插件。该插件(StackReg)是TurboReg 插件的前端。这些插件之间有几种数据交换机制,其中之一是将临时文件写入 ImageJ 定义的临时目录。该目录的位置可以通过宏命令 "print(getDirectory("temp"));
"查看。文件名称是:StackRegSource,StackRegSourceR,StackRegSourceG,StackRegSourceB,StackRegTarget。
+配准完成后,原始堆栈将被销毁,取而代之的是配准结果。堆栈的名称和类型保持不变;除 RGB 堆栈和 HSB 堆栈外,所有数据类型均可使用。(请注意,RGB-stack 是由三个颜色分量组成的堆栈,不应与 RGB 彩色图像堆栈混淆:后者可以使用,而前者不能使用。如有疑问,请尝试一下)。如果提供的是彩色图像堆栈,则会在灰度版本上进行配准,灰度版本是由红色、绿色和蓝色分量加权求和而成的,即使堆栈是伪彩色图像也是如此。权重取决于整个图像堆栈,并通过主成分分析确定,以提供最大可能的对比度。一旦根据中间灰度表示确定了几何图形,就可以重建真正的彩色图像,如果需要生成伪彩色图像,还可以重新量化。
+
+示例
+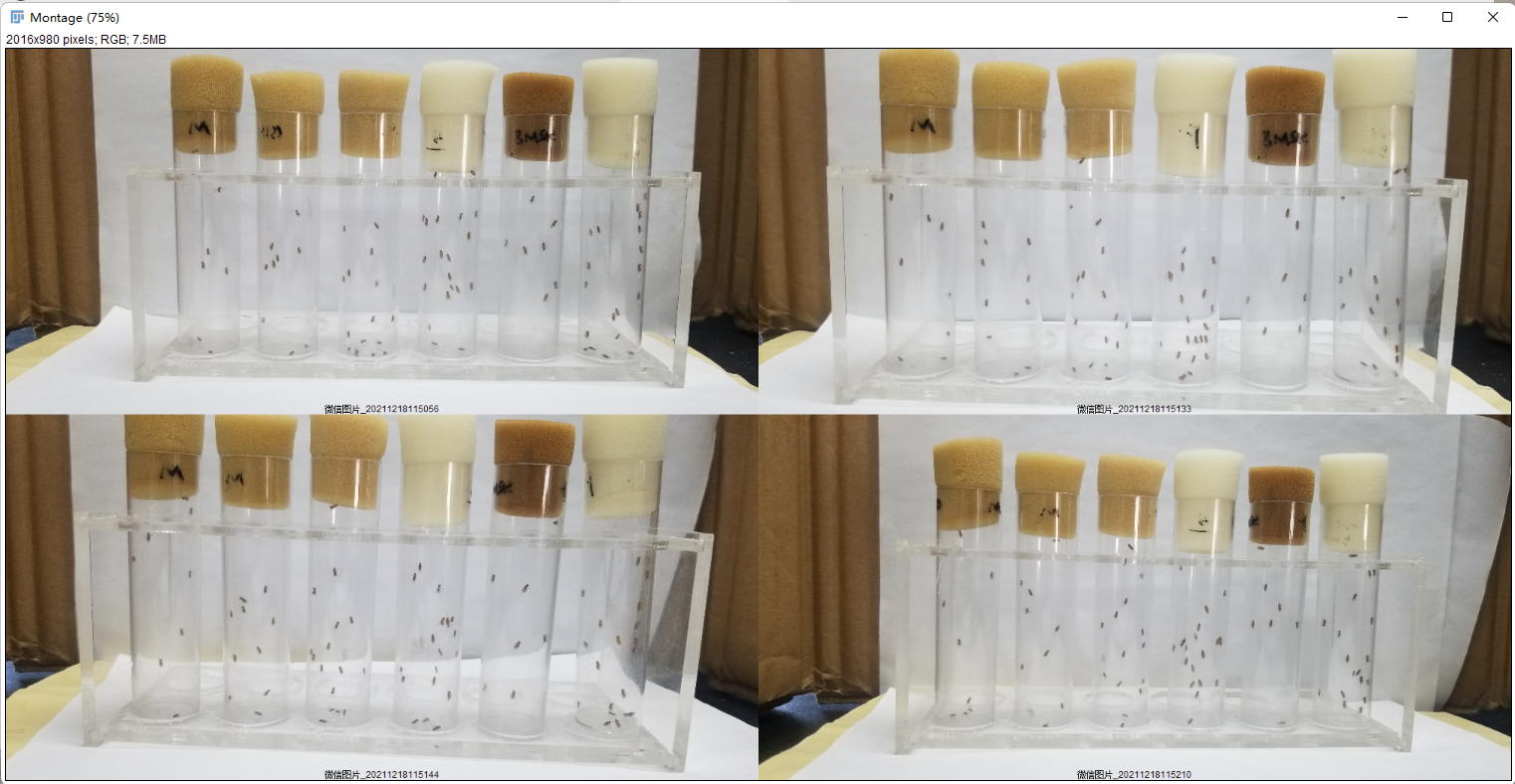
原始图
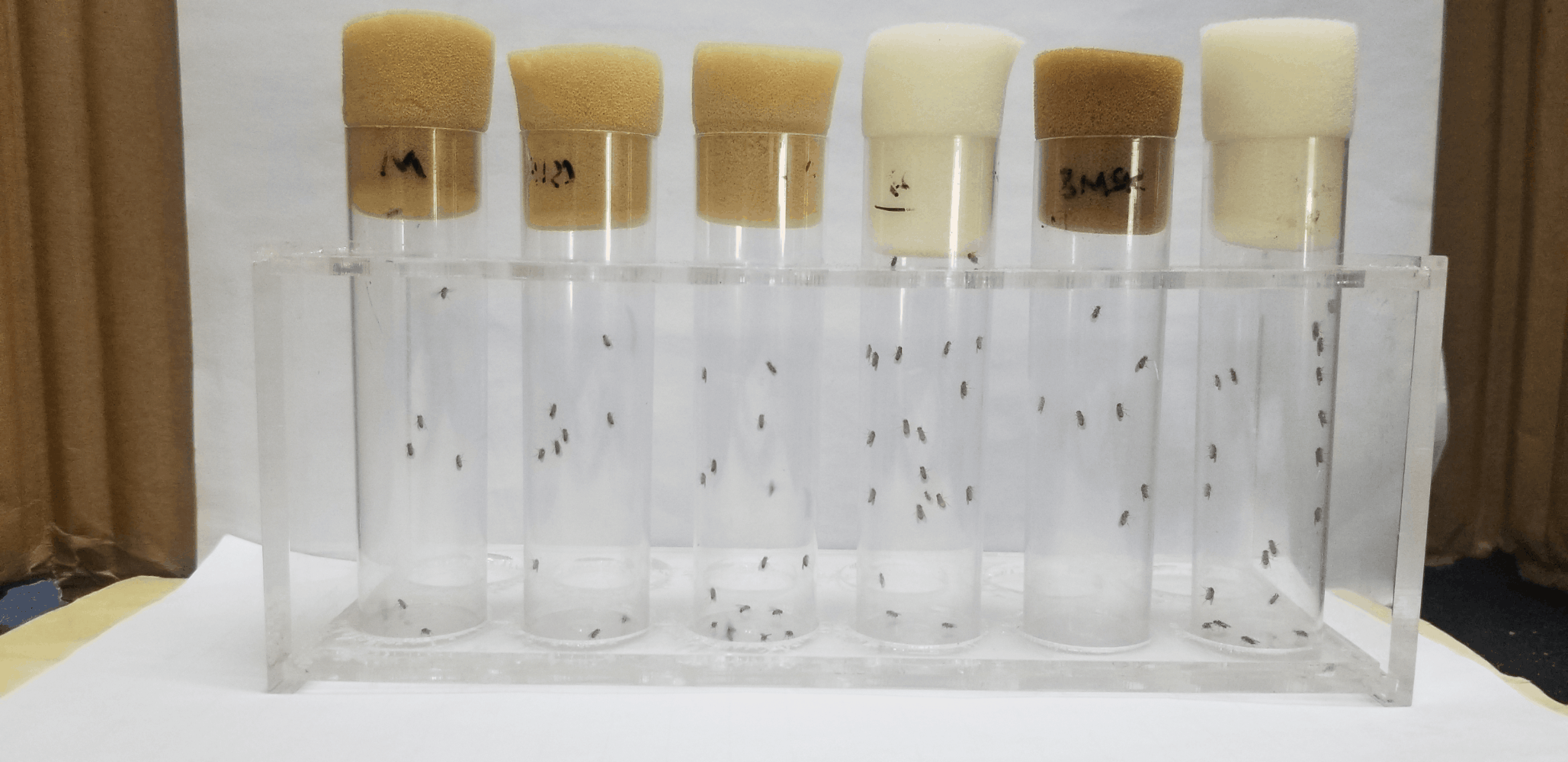
配准后(转换类型Scaled rotation)
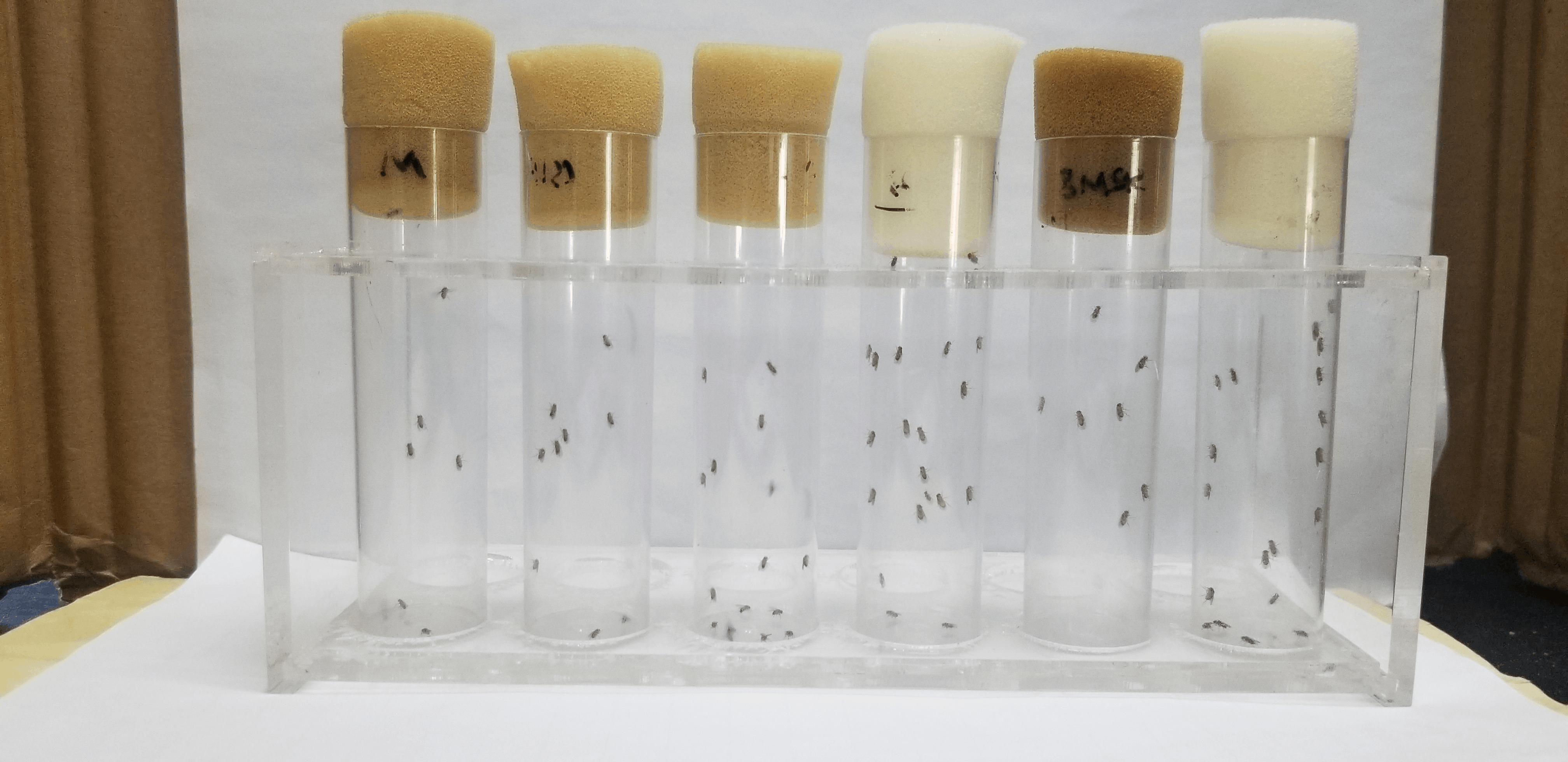
+
+参数
+有四种类型的转换:
+
+-
+
Translation:
+
+
+映射公式为
。其中,输入坐标 u 映射到输出坐标 x,常数矢量 Δu 表示平移量。
+
+-
+
Rigid Body:
+
+
+映射公式为

由于存在 Δu 项,因此除了旋转角度 θ 外,还考虑了平移。
+
+-
+
Scaled rotation:
+
+
+映射公式为

与Rigid Body 的区别在于存在标量调整因子 λ。
+
+-
+
Affine:
+
+
+映射公式为

由于四个系数
、
、
和
是独立的,因此仿射变换比按比例旋转具有更多的自由度。
+
+操作步骤
+使用的Fiji ImageJ
+
+-
+
拖动图片文件夹到Fiji,默认为堆栈。
+
+
+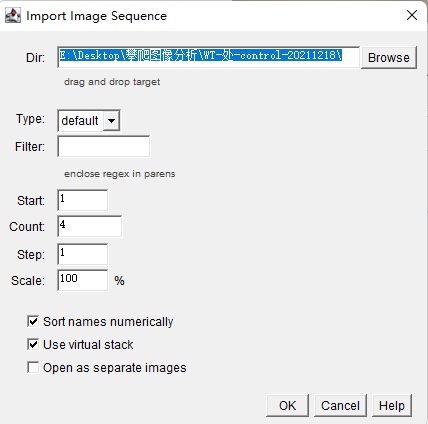
+
+-
+
插件启动前,选择合适的图片,当前图片会被充当全局锚点。
+
+-
+
打开StackReg。
+
+
+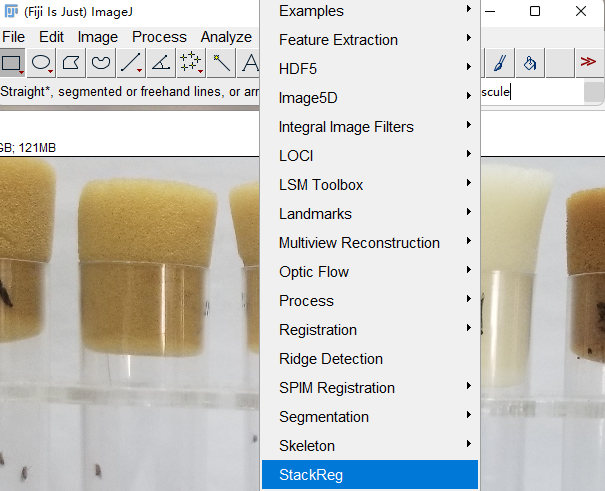
+
+-
+
选择对齐方式,点击确定按钮。
+
+
+
+配准结果展示
+Translation
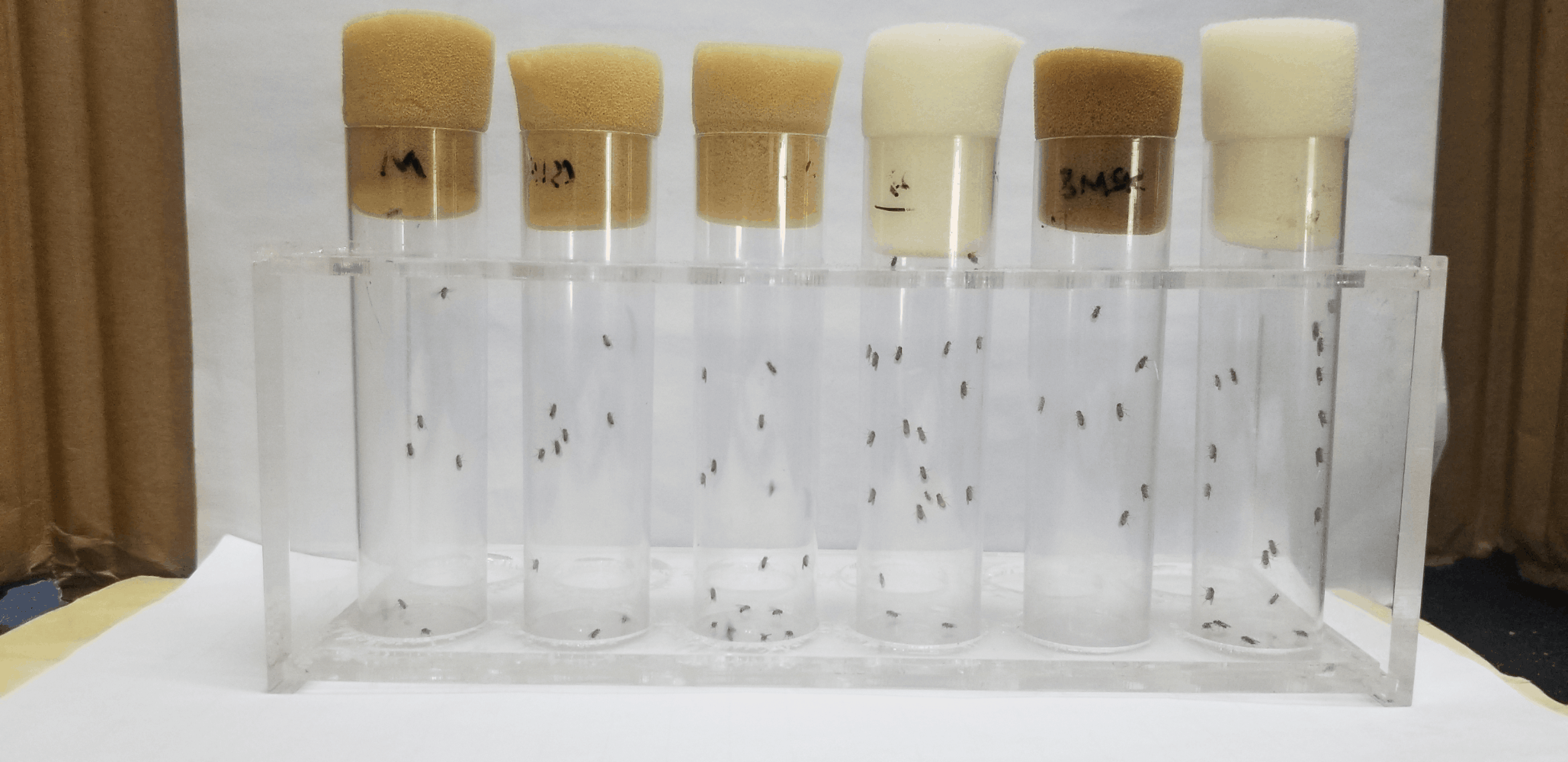
Rigid Body
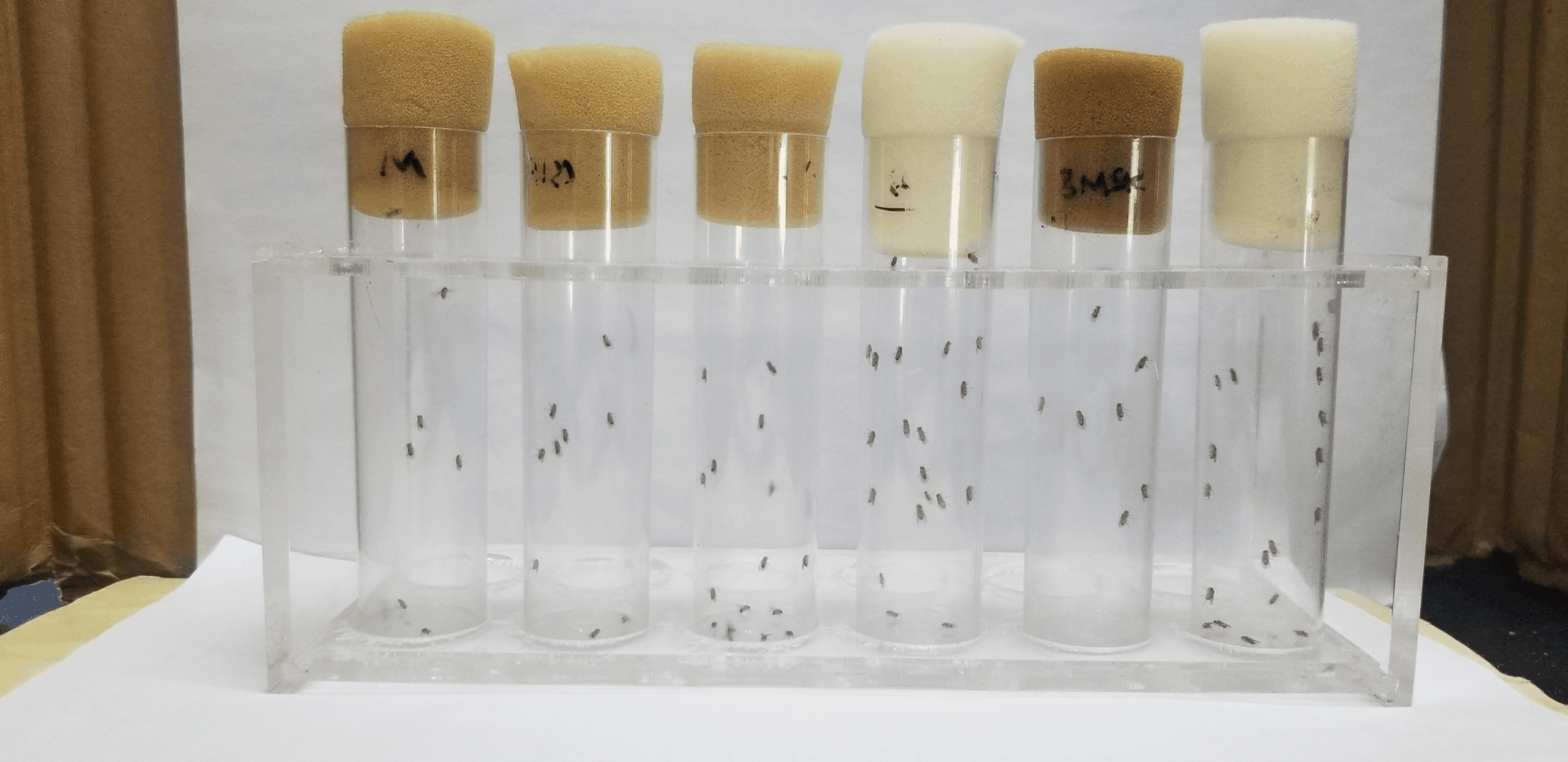
Scaled rotation
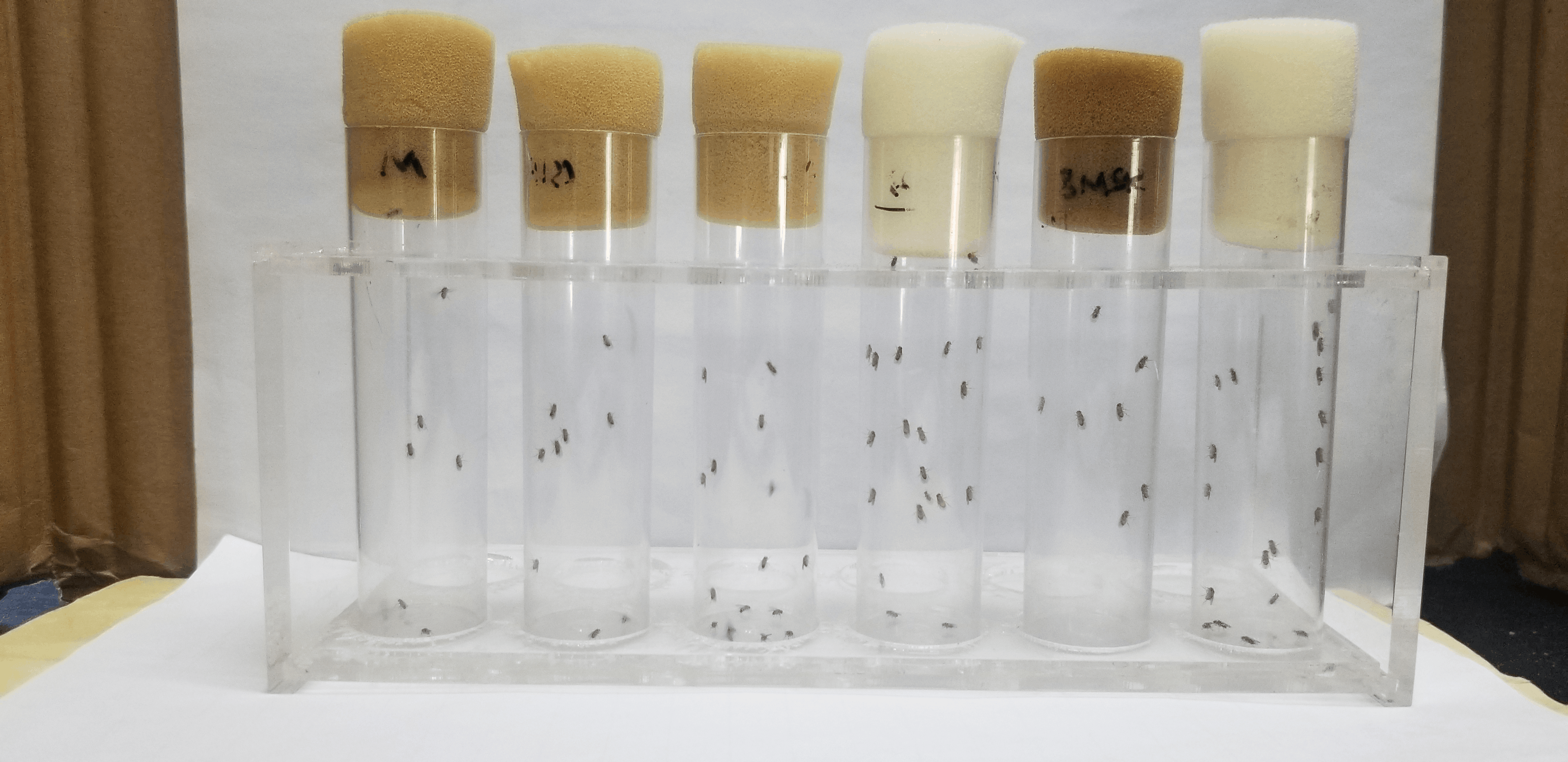
Affine
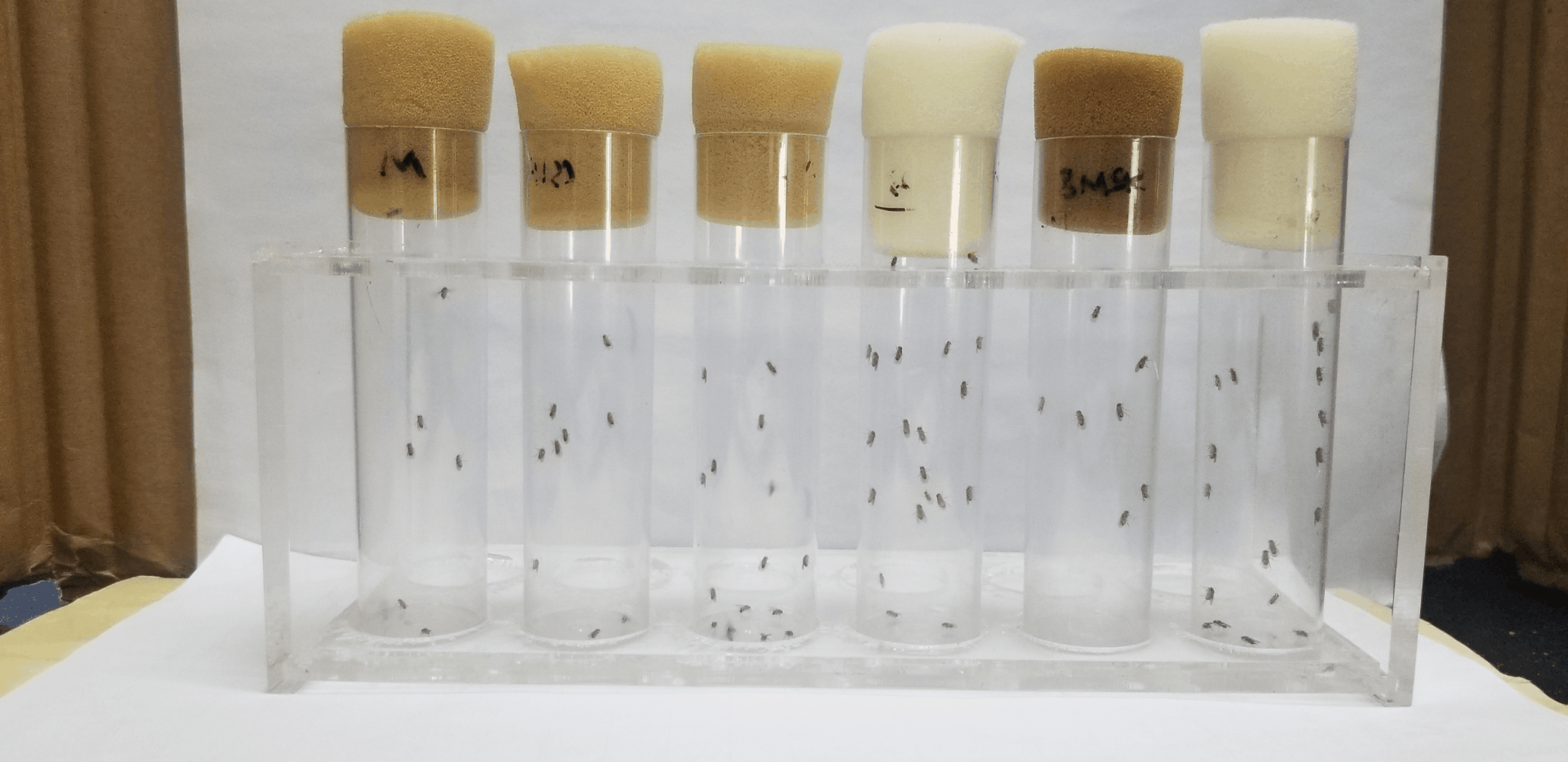
+
+脚本
+StackReg 是可编写脚本和使用宏记录的。下面是一个从宏调用 StackReg 的示例:
+run("StackReg ", "transformation=[Rigid Body]");
+
+参考
+
+-
+
https://bigwww.epfl.ch/thevenaz/stackreg/
+
+-
+
P. Thévenaz, U.E. Ruttimann, M. Unser, “A Pyramid Approach to Subpixel Registration Based on Intensity,” IEEE Transactions on Image Processing, vol. 7, no. 1, pp. 27-41, January 1998. Other relevant on-line publications are available at http://bigwww.epfl.ch/publications/.
+
+
+]]>
- R语言
+ ImageJ
- R语言
- 批量
- 实例
- 数据处理
- 果蝇
+ 笔记
+ ImageJ
+ 图像
+ 插件
+
+
+
+ R语言--SimpleITK
+ /2024/05/16/ef7e309aa1eb/
+ SimpleITK 是 Insight 工具包(ITK)算法和数据结构的简化编程接口。它支持多种编程语言的接口,包括 C++、Python、R、Java、C#、Lua、Ruby 和 TCL。这些绑定使科学家能够用他们最熟悉的编程语言开发图像分析工作流。该工具包支持 15 种以上不同的图像文件格式,提供 280 多种图像分析过滤器,并为 ITK 基于强度的配准框架实现了统一接口。
+
+特点
+使用 Python、R、Java、C#、Lua、Ruby、TCL 和 C++ 进行开源多维图像分析。由 Insight 工具包社区为生物医学科学及其他领域开发。
+
+- 被视为空间对象而非像素阵列的图像。计算在二维或三维物理空间中进行。
+- 利用刚性或可变形变换快速配准二维和三维模内和模间图像的配准框架。
+- 从经典的Otsu thresholding到水平集(level sets) 和分水岭(watersheds),为图像分割工作流程提供了丰富的过滤器。
+- 评估分割结果(豪斯多夫距离、雅卡德值和骰子值、表面距离等<Hausdorff distance, Jaccard and Dice values, surface distances etc.> )和分析分割形状特征(定向边界框、主矩、周长、伸长率、费雷特直径等<oriented bounding box, principal moments, perimeter, elongation, Feret diameter etc.>)的工具。
+- 图像文件输入/输出,支持 20 多种图像文件格式(jpg、png、DICOM、TIFF 等),并可在不同格式间轻松转换。
+- 通过基于进程和线程的并行化,可随时集成到集群或台式机的并行处理框架中。
+
+安装R包-SimpleITKRInstaller
+devtools::install_github("SimpleITK/SimpleITKRInstaller")
+Fiji
+SimpleITK 有一个内置函数 itk::simple::Show()
,可用于在交互会话中查看图像。默认情况下,该 Show 函数会搜索已安装的 Fiji 来显示图像。如果找不到 Fiji,则会搜索 ImageJ。选择 Fiji/ImageJ 是因为它们可以处理 SimpleITK 支持的所有图像类型,包括每像素有 n 个分量的 3D 矢量图像。 Show 函数会在多个标准位置搜索 Fiji/ImageJ。具体位置取决于系统类型(Linux、Mac 或 Windows)。启用 "Show "的 "debugOn "选项后,用户可以看到 SimpleITK 正在搜索 Fiji(或其他查看应用程序)的位置,以及用于启动查看应用程序的实际命令。 Show 是 ImageViewer 类的功能接口。可以使用 ImageViewer 对象配置其他查看应用程序.
+
+图片导入和导出
+library(SimpleITK)
reader <- ImageFileReader()
reader$SetImageIO("PNGImageIO")
reader$SetFileName(inputImageFileName)
image <- reader$Execute()
writer <- ImageFileWriter()
writer$SetFileName(outputImageFileName)
writer$Execute(image)
+上面的示例指定使用 PNGImageIO 来读取文件。如果省略该行,SimpleITK 将根据文件名的后缀和/或文件头自动确定要使用哪个 IO。
+image <- ReadImage(inputImageFileName)
WriteImage(image, outputImageFileName)
+]]>
+
+ ImageJ
+
+
+ 笔记
+ ImageJ
+ 图像
+ 插件
+ 简介
@@ -699,42 +716,6 @@
API
-
- R语言--SimpleITK
- /2024/05/16/ef7e309aa1eb/
- SimpleITK 是 Insight 工具包(ITK)算法和数据结构的简化编程接口。它支持多种编程语言的接口,包括 C++、Python、R、Java、C#、Lua、Ruby 和 TCL。这些绑定使科学家能够用他们最熟悉的编程语言开发图像分析工作流。该工具包支持 15 种以上不同的图像文件格式,提供 280 多种图像分析过滤器,并为 ITK 基于强度的配准框架实现了统一接口。
-
-特点
-使用 Python、R、Java、C#、Lua、Ruby、TCL 和 C++ 进行开源多维图像分析。由 Insight 工具包社区为生物医学科学及其他领域开发。
-
-- 被视为空间对象而非像素阵列的图像。计算在二维或三维物理空间中进行。
-- 利用刚性或可变形变换快速配准二维和三维模内和模间图像的配准框架。
-- 从经典的Otsu thresholding到水平集(level sets) 和分水岭(watersheds),为图像分割工作流程提供了丰富的过滤器。
-- 评估分割结果(豪斯多夫距离、雅卡德值和骰子值、表面距离等<Hausdorff distance, Jaccard and Dice values, surface distances etc.> )和分析分割形状特征(定向边界框、主矩、周长、伸长率、费雷特直径等<oriented bounding box, principal moments, perimeter, elongation, Feret diameter etc.>)的工具。
-- 图像文件输入/输出,支持 20 多种图像文件格式(jpg、png、DICOM、TIFF 等),并可在不同格式间轻松转换。
-- 通过基于进程和线程的并行化,可随时集成到集群或台式机的并行处理框架中。
-
-安装R包-SimpleITKRInstaller
-devtools::install_github("SimpleITK/SimpleITKRInstaller")
-Fiji
-SimpleITK 有一个内置函数 itk::simple::Show()
,可用于在交互会话中查看图像。默认情况下,该 Show 函数会搜索已安装的 Fiji 来显示图像。如果找不到 Fiji,则会搜索 ImageJ。选择 Fiji/ImageJ 是因为它们可以处理 SimpleITK 支持的所有图像类型,包括每像素有 n 个分量的 3D 矢量图像。 Show 函数会在多个标准位置搜索 Fiji/ImageJ。具体位置取决于系统类型(Linux、Mac 或 Windows)。启用 "Show "的 "debugOn "选项后,用户可以看到 SimpleITK 正在搜索 Fiji(或其他查看应用程序)的位置,以及用于启动查看应用程序的实际命令。 Show 是 ImageViewer 类的功能接口。可以使用 ImageViewer 对象配置其他查看应用程序.
-
-图片导入和导出
-library(SimpleITK)
reader <- ImageFileReader()
reader$SetImageIO("PNGImageIO")
reader$SetFileName(inputImageFileName)
image <- reader$Execute()
writer <- ImageFileWriter()
writer$SetFileName(outputImageFileName)
writer$Execute(image)
-上面的示例指定使用 PNGImageIO 来读取文件。如果省略该行,SimpleITK 将根据文件名的后缀和/或文件头自动确定要使用哪个 IO。
-image <- ReadImage(inputImageFileName)
WriteImage(image, outputImageFileName)
-]]>
-
- ImageJ
-
-
- 笔记
- ImageJ
- 图像
- 插件
- 简介
-
-
Hello World
/2021/01/01/145a1920f348/
@@ -928,6 +909,25 @@
行动
+
+ R语言--爬取小说-战略级天使
+ /2021/05/04/dfc6fd522f6c/
+ 目的
+小说离线阅读,保存。练习R语言。示例网站:https://www.-----.com/yuedu/15111/
+
+实例
+library("stringr")
library("rvest")
library('RCurl')
library('curl')
library('downloader')
library('RSelenium')
setwd('H:\\R\\R脚本\\爬虫')
url <- "https://www.-----.com/yuedu/15111/"
htmlpage <- read_html(url)
xs_chapter <- htmlpage %>% html_nodes('div#wrapper div.box_con div#list dl dd a') %>% html_text()
head(xs_chapter)#观察数据形式
xs_link <- htmlpage %>% html_nodes('div#wrapper div.box_con div#list dl dd a') %>% html_attrs()
head(xs_link)#观察数据形式
xs_link <- paste("https://www.-----.com",unlist(xs_link),sep = '')#构建章节链接
xs_content <- cbind(xs_chapter[-(1:10)],xs_link[-(1:10)])#合并目录链接
#尝试获取小说内容,并提取。
ms_f1 <- read_html(xs_content[1,2], encoding = "GB18030") %>% html_nodes('div#content') %>% html_text()
ms_f2 <- read_html(xs_content[2,2], encoding = "GB18030") %>% html_nodes('div#content') %>% html_text()
ms_f <- c(xs_content[1,1],ms_f1,xs_content[2,1],ms_f2)
write(gsub("\u00A0"," ", ms_f),file = "1.txt")
#自定义下载函数
xs_down <- function(x){
xs <- c()
for (i in 1:length(x[,1])) {
xm <- read_html(x[i,2], encoding = "GB18030") %>% html_nodes('div#content') %>% html_text()
xs <- c(xs, x[i,1], xm)
}
xs
}
#导出小说
write(gsub("\u00A0"," ", xs_down(xs_content)),file = "战略级天使.txt")
]]>
+
+ 爬虫
+ R语言
+
+
+ R语言
+ 实例
+ 爬虫
+ 小说
+
+
从出生那天开始,你就是“局外人”
/2024/05/10/51fa0d872217/
@@ -968,56 +968,6 @@
时代
-
- 大模型读文献之腾讯元宝--深度阅读
- /2024/09/25/7453fb1cbc6c/
- 简介
-腾讯元宝的深度阅读模式是一种创新的阅读方式,它利用人工智能技术,为用户提供了一种全新的长文阅读体验。这种模式不仅能够处理大量文本,还能生成图文并茂的内容,极大地提升了阅读效率和理解深度。
-
-深度阅读模式的特点
-
-
-- 长文阅读支持:深度阅读模式支持长达近50万字的专业文档,适用于学术研究、市场分析、财务报告等领域。
-- 图文并茂的内容重塑:通过多模态内容生成技术,深度阅读模式能够展示原始插图或通过算法生成的高级分析图表,使信息传达更加直观。
-- 智慧导航:配备划词搜索和翻译功能,帮助用户无缝理解专业术语,特别适用于跨语言研究。
-- 专业图表的智能生成:针对财报和研究报告,能够自动识别关键数据,生成杜邦分析图等专业图表,并结合内置的计算器功能确保数值的精准计算。
-
-
-
-示例
-
--
-
打开元宝 (https://yuanbao.tencent.com/) ,需要登录使用。界面很简洁,点击输入框上面的“深度阅读”
-
-
-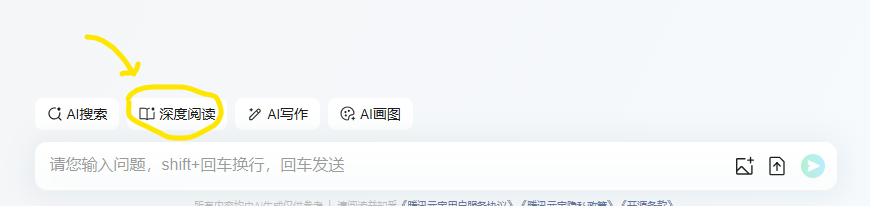
-弹出文件上传框,拖动pdf到上传框。
-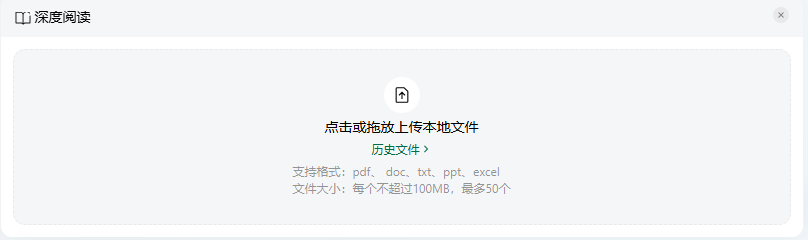
-
--
-
阅读界面如下,可以看到总结、精度、翻译、脑图功能。还可以直接根据文献内容生成学术海报。所有生成结果均可下载。下载格式为图片和pdf,生成的pdf对公示显示不友好。
-
-
-
-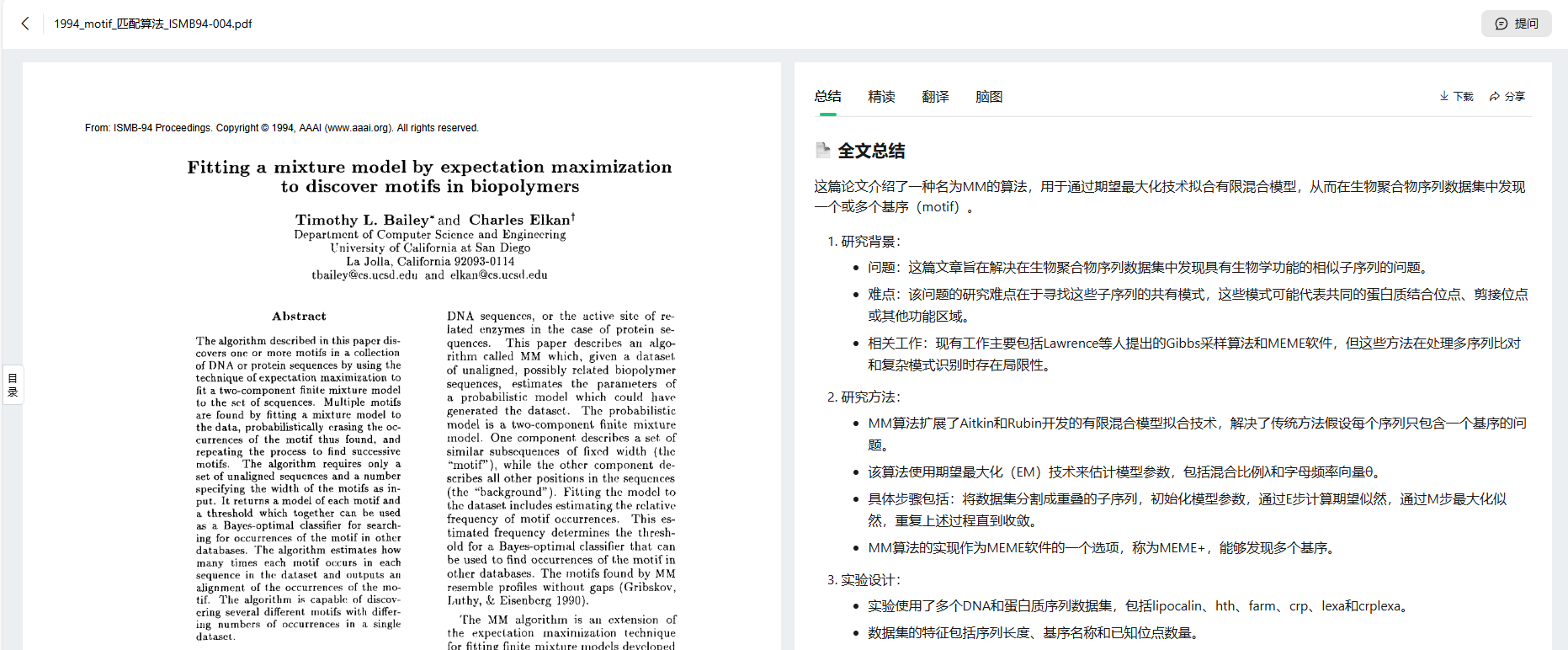
-
--
-
文献阅读部分结果展示:
-
-
-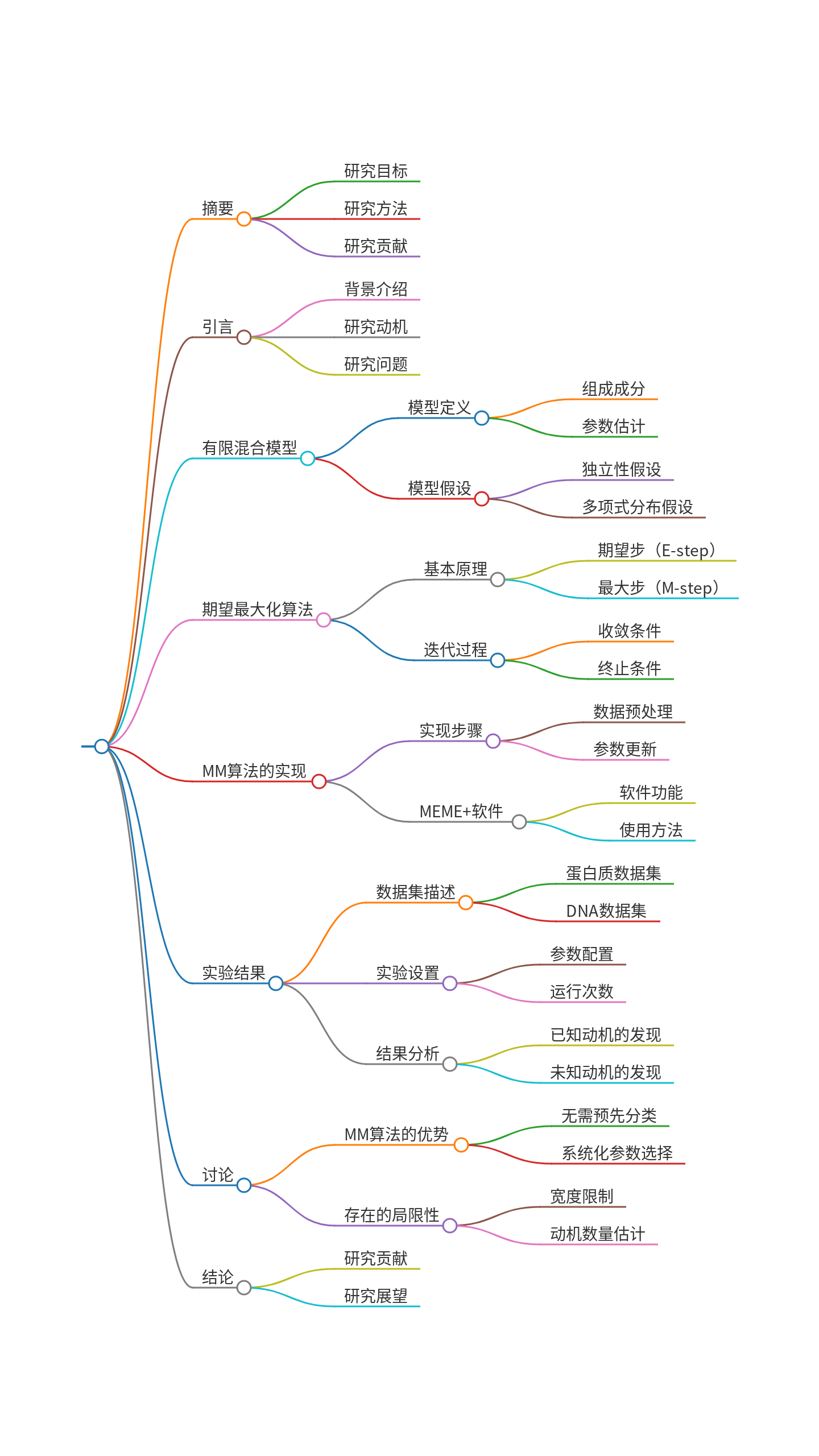
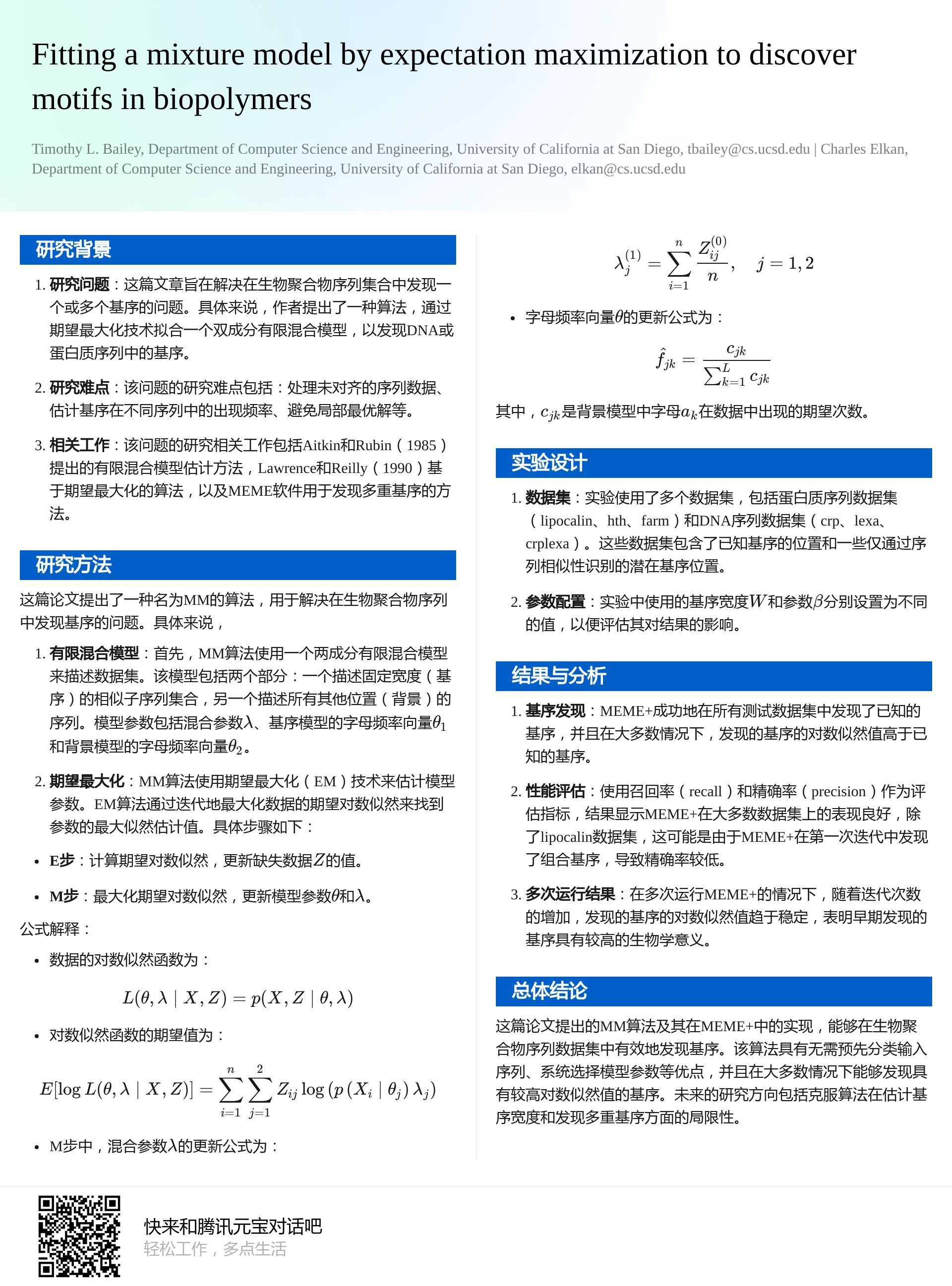
-]]>
-
- 文献阅读
- 大模型
-
-
- 阅读
- 腾讯
- 大模型
- 元宝
-
-
蛋白互作网络PPI分析流程示例
/2024/09/25/35832a441121/
@@ -1136,22 +1086,72 @@
- R语言--爬取小说-战略级天使
- /2021/05/04/dfc6fd522f6c/
+ 大模型读文献之腾讯元宝--深度阅读
+ /2024/09/25/7453fb1cbc6c/
+ 简介
+腾讯元宝的深度阅读模式是一种创新的阅读方式,它利用人工智能技术,为用户提供了一种全新的长文阅读体验。这种模式不仅能够处理大量文本,还能生成图文并茂的内容,极大地提升了阅读效率和理解深度。
+
+深度阅读模式的特点
+
+
+- 长文阅读支持:深度阅读模式支持长达近50万字的专业文档,适用于学术研究、市场分析、财务报告等领域。
+- 图文并茂的内容重塑:通过多模态内容生成技术,深度阅读模式能够展示原始插图或通过算法生成的高级分析图表,使信息传达更加直观。
+- 智慧导航:配备划词搜索和翻译功能,帮助用户无缝理解专业术语,特别适用于跨语言研究。
+- 专业图表的智能生成:针对财报和研究报告,能够自动识别关键数据,生成杜邦分析图等专业图表,并结合内置的计算器功能确保数值的精准计算。
+
+
+
+示例
+
+-
+
打开元宝 (https://yuanbao.tencent.com/) ,需要登录使用。界面很简洁,点击输入框上面的“深度阅读”
+
+
+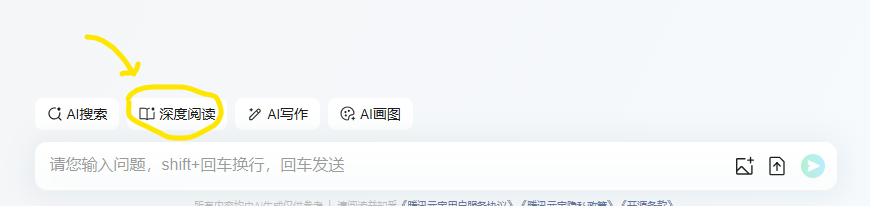
+弹出文件上传框,拖动pdf到上传框。
+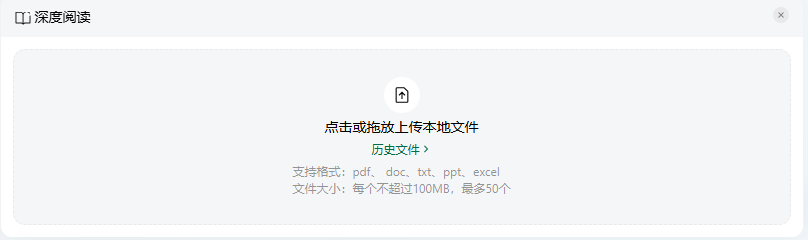
+
+-
+
阅读界面如下,可以看到总结、精度、翻译、脑图功能。还可以直接根据文献内容生成学术海报。所有生成结果均可下载。下载格式为图片和pdf,生成的pdf对公示显示不友好。
+
+
+
+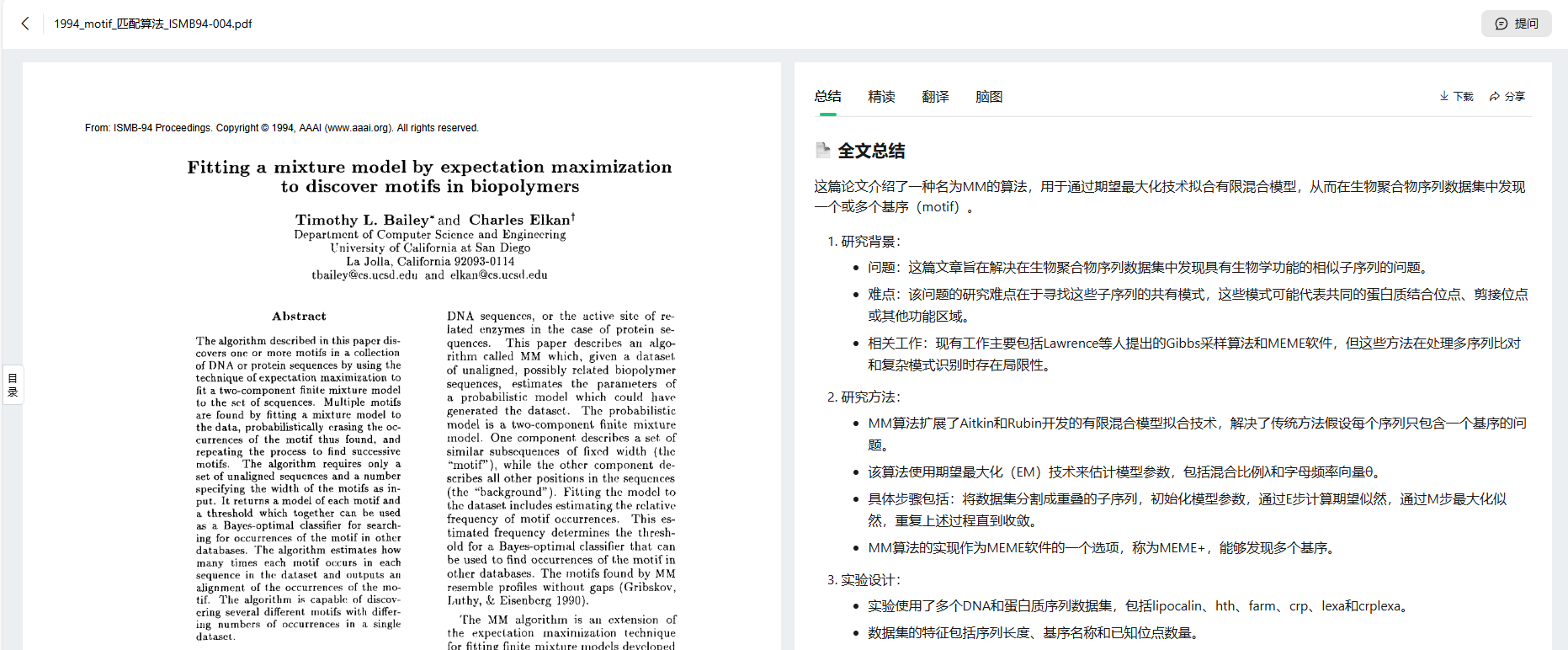
+
+-
+
文献阅读部分结果展示:
+
+
+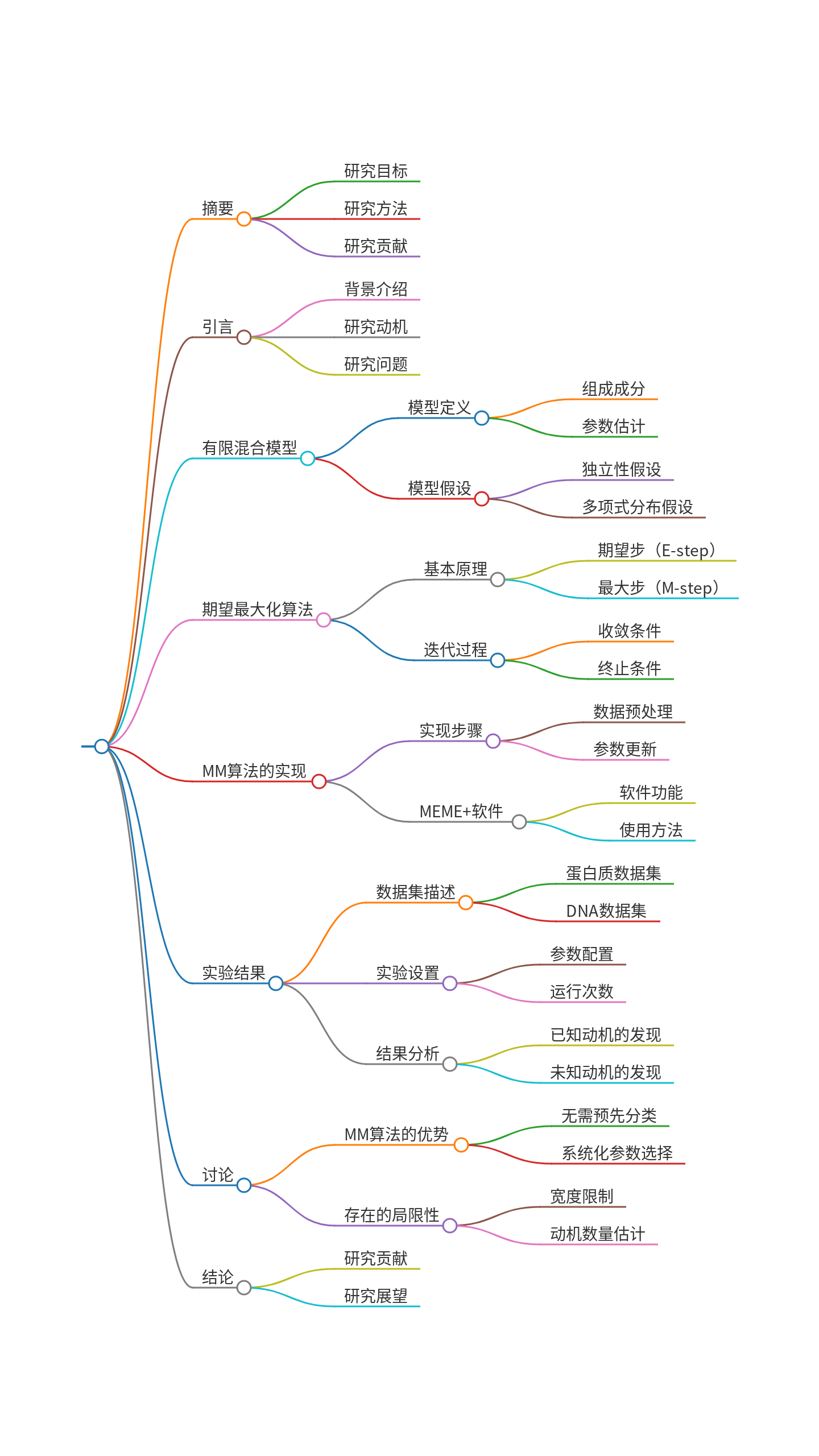
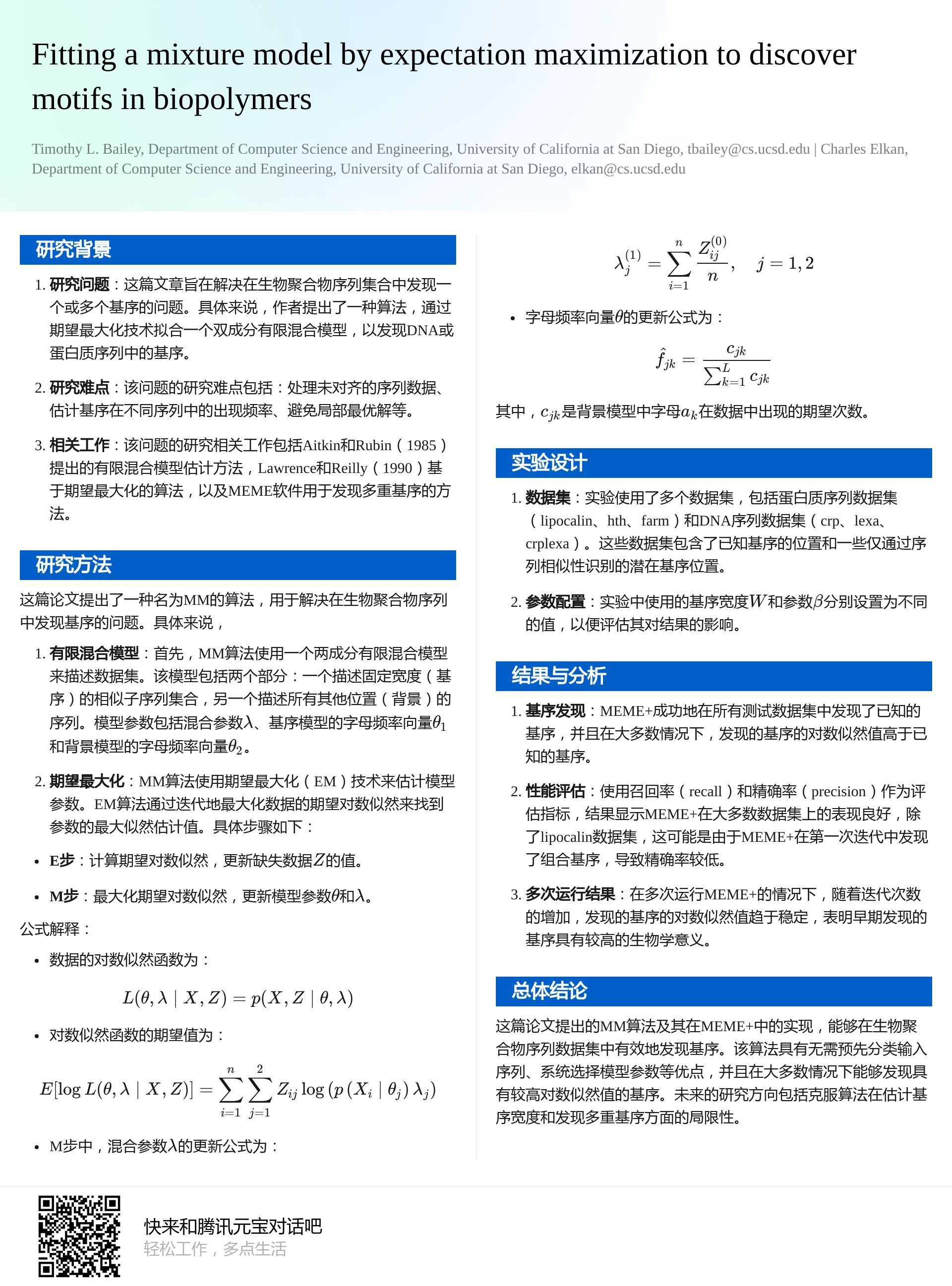
+]]>
+
+ 文献阅读
+ 大模型
+
+
+ 阅读
+ 腾讯
+ 大模型
+ 元宝
+
+
+
+ R语言--果蝇攀爬--ImageJ测量数据处理
+ /2024/07/03/edfd8de9558b/
目的
-小说离线阅读,保存。练习R语言。示例网站:https://www.-----.com/yuedu/15111/
+处理ImageJ测量的果蝇攀爬数据
实例
-library("stringr")
library("rvest")
library('RCurl')
library('curl')
library('downloader')
library('RSelenium')
setwd('H:\\R\\R脚本\\爬虫')
url <- "https://www.-----.com/yuedu/15111/"
htmlpage <- read_html(url)
xs_chapter <- htmlpage %>% html_nodes('div#wrapper div.box_con div#list dl dd a') %>% html_text()
head(xs_chapter)#观察数据形式
xs_link <- htmlpage %>% html_nodes('div#wrapper div.box_con div#list dl dd a') %>% html_attrs()
head(xs_link)#观察数据形式
xs_link <- paste("https://www.-----.com",unlist(xs_link),sep = '')#构建章节链接
xs_content <- cbind(xs_chapter[-(1:10)],xs_link[-(1:10)])#合并目录链接
#尝试获取小说内容,并提取。
ms_f1 <- read_html(xs_content[1,2], encoding = "GB18030") %>% html_nodes('div#content') %>% html_text()
ms_f2 <- read_html(xs_content[2,2], encoding = "GB18030") %>% html_nodes('div#content') %>% html_text()
ms_f <- c(xs_content[1,1],ms_f1,xs_content[2,1],ms_f2)
write(gsub("\u00A0"," ", ms_f),file = "1.txt")
#自定义下载函数
xs_down <- function(x){
xs <- c()
for (i in 1:length(x[,1])) {
xm <- read_html(x[i,2], encoding = "GB18030") %>% html_nodes('div#content') %>% html_text()
xs <- c(xs, x[i,1], xm)
}
xs
}
#导出小说
write(gsub("\u00A0"," ", xs_down(xs_content)),file = "战略级天使.txt")
]]>
+library(cluster)
# 设定工作目录
setwd("E:/Desktop/")
# 导入数据
X20180609_WF_IF <- read.csv("E:/Desktop/20180609-WF-IF.csv")
X20180609_CM_AM <- read.csv("E:/Desktop/20180609-CM-AM.csv")
X20180609_CF_AF <- read.csv("E:/Desktop/20180609-CF-AF.csv")
clusGap(X20180609_WF_IF$X[which(X20180609_WF_IF$Slice==1)],FUN=kmeans,nstart=25,K.max=10,B=500)
## 自定义函数,获取每管果蝇的攀爬指数----
ppindex <- function(X){
g1 <- c()
g2 <- c()
g3 <- c()
g4 <- c()
g5 <- c()
g6 <- c()
for (i in 1:max(X$Slice)) {
slice_x <- X$X[which(X$Slice==i)]
slice_y <- X$Y[which(X$Slice==i)]
slice_max <- (max(slice_x)+100)/6
par(mfrow = c(1,6))
# 管1
slice_x <- slice_x[which(slice_x < slice_max)]
slice_y <- slice_y[which(slice_x < slice_max)]
plot(slice_x,slice_y)
slice_y_fq0 <- length(slice_y[which(slice_y < 200)])
slice_y_fq1 <- length(slice_y[which(slice_y > 200&slice_y < 400)])
slice_y_fq2 <- length(slice_y[which(slice_y > 400)])-2
index_1 <- (slice_y_fq0*0+slice_y_fq1*1+slice_y_fq2*2)/(slice_y_fq0+slice_y_fq1+slice_y_fq2)
g1 <- c(g1,index_1)
# 管2
slice_x2 <- slice_x[which(slice_max < slice_x&slice_x < slice_max*2)]
slice_y2 <- slice_y[which(slice_max < slice_x&slice_x < slice_max*2)]
plot(slice_x2,slice_y2)
slice_y2_fq0 <- length(slice_y2[which(slice_y2 < 200)])
slice_y2_fq1 <- length(slice_y2[which(slice_y2 > 200&slice_y2 < 400)])
slice_y2_fq2 <- length(slice_y2[which(slice_y2 > 400)])-2
index_2 <- (slice_y2_fq0*0+slice_y2_fq1*1+slice_y2_fq2*2)/(slice_y2_fq0+slice_y2_fq1+slice_y2_fq2)
g2 <- c(g2,index_2)
# 管3
slice_x3 <- slice_x[which(slice_max*2 < slice_x&slice_x < slice_max*3)]
slice_y3 <- slice_y[which(slice_max*2 < slice_x&slice_x < slice_max*3)]
plot(slice_x3,slice_y3)
slice_y3_fq0 <- length(slice_y3[which(slice_y3 < 200)])
slice_y3_fq1 <- length(slice_y3[which(slice_y3 > 200&slice_y3 < 400)])
slice_y3_fq2 <- length(slice_y3[which(slice_y3 > 400)])-2
index_3 <- (slice_y3_fq0*0+slice_y3_fq1*1+slice_y3_fq2*2)/(slice_y3_fq0+slice_y3_fq1+slice_y3_fq2)
g3 <- c(g3,index_3)
# 管4
slice_x4 <- slice_x[which(slice_max*3 < slice_x&slice_x < slice_max*4)]
slice_y4 <- slice_y[which(slice_max*3 < slice_x&slice_x < slice_max*4)]
plot(slice_x4,slice_y4)
slice_y4_fq0 <- length(slice_y4[which(slice_y4 < 200)])
slice_y4_fq1 <- length(slice_y4[which(slice_y4 > 200&slice_y4 < 400)])
slice_y4_fq2 <- length(slice_y4[which(slice_y4 > 400)])-2
index_4 <- (slice_y4_fq0*0+slice_y4_fq1*1+slice_y4_fq2*2)/(slice_y4_fq0+slice_y4_fq1+slice_y4_fq2)
g4 <- c(g4,index_4)
# 管5
slice_x5 <- slice_x[which(slice_max*4 < slice_x&slice_x < slice_max*5)]
slice_y5 <- slice_y[which(slice_max*4 < slice_x&slice_x < slice_max*5)]
plot(slice_x5,slice_y5)
slice_y5_fq0 <- length(slice_y5[which(slice_y5 < 200)])
slice_y5_fq1 <- length(slice_y5[which(slice_y5 > 200&slice_y5 < 400)])
slice_y5_fq2 <- length(slice_y5[which(slice_y5 > 400)])-2
index_5 <- (slice_y5_fq0*0+slice_y5_fq1*1+slice_y5_fq2*2)/(slice_y5_fq0+slice_y5_fq1+slice_y5_fq2)
g5 <- c(g5,index_5)
# 管6
slice_x6 <- slice_x[which(slice_max*5 < slice_x&slice_x < slice_max*6)]
slice_y6 <- slice_y[which(slice_max*5 < slice_x&slice_x < slice_max*6)]
plot(slice_x6,slice_y6)
slice_y6_fq0 <- length(slice_y6[which(slice_y6 < 200)])
slice_y6_fq1 <- length(slice_y6[which(slice_y6 > 200&slice_y6 < 400)])
slice_y6_fq2 <- length(slice_y6[which(slice_y6 > 400)])-2
index_6 <- (slice_y6_fq0*0+slice_y6_fq1*1+slice_y6_fq2*2)/(slice_y6_fq0+slice_y6_fq1+slice_y6_fq2)
g6 <- c(g6,index_6)
}
flyindex <- data.frame(g1,g2,g3,g4,g5,g6)
return(flyindex)
}
#汇总----
WI_F_Index <- ppindex(X20180609_WF_IF)
names(WI_F_Index) <- c("WF1","WF2","WF3","IF1","IF2","IF3")
WI_F_Index <- ppindex(X20180609_CF_AF)
names(CA_F_Index) <- c("CF1","CF2","CF3","AF1","AF2","AF3")
CA_M_Index <- ppindex(X20180609_CM_AM)
names(CA_M_Index) <- c("CM1","CM2","CM3","AM1","AM2","AM3")
# 保存
library(xlsx)
write.xlsx2(WI_F_Index, sheetName = "WI_F_Index", file='flyIndex.xlsx',append = TRUE)
write.xlsx2(CA_F_Index, sheetName = "CA_F_Index", file='flyIndex.xlsx',append = TRUE)
write.xlsx2(CA_M_Index, sheetName = "CA_M_Index", file='flyIndex.xlsx',append = TRUE)
dev.off()
###########################################################################################
#单管----
# 导入数据
X20180609_IM3 <- read.csv("E:/Desktop/20180609-IM3.csv")
X20180609_IM2 <- read.csv("E:/Desktop/20180609-IM2.csv")
X20180609_IM1 <- read.csv("E:/Desktop/20180609-IM1.csv")
X20180609_WM3 <- read.csv("E:/Desktop/20180609-WM3.csv")
X20180609_WM2 <- read.csv("E:/Desktop/20180609-WM2.csv")
X20180609_WM1 <- read.csv("E:/Desktop/20180609-WM1.csv")
pp2index <- function(x) {
index <- c()
for (i in 1:max(x$Slice)) {
slice_x <- x$X[which(x$Slice == i)]
slice_y <- x$Y[which(x$Slice == i)]
slice_y_fq0 <- length(slice_y[which(slice_y < 340)])
slice_y_fq1 <- length(slice_y[which(slice_y > 340 &
slice_y < 600)])
if ((length(slice_y[which(slice_y > 600 &
slice_y < 900)]) - 1) < 0) {
slice_y_fq2 <- 0
} else {
slice_y_fq2 <- length(slice_y[which(slice_y > 600 &
slice_y < 900)]) - 1
}
if ((length(slice_y[which(slice_y > 900 &
slice_y < 1200)]) - 1) < 0) {
slice_y_fq3 <- 0
} else {
slice_y_fq3 <- length(slice_y[which(slice_y > 900 &
slice_y < 1200)]) - 1
}
if ((length(slice_y[which(slice_y > 1200)]) - 1) < 0) {
slice_y_fq4 <- 0
} else {
slice_y_fq4 <- length(slice_y[which(slice_y > 1200)]) - 1
}
index_1 <- (slice_y_fq0 * 0 + slice_y_fq1 * 1 + slice_y_fq2 * 2 + slice_y_fq3 *
3 + slice_y_fq4 * 4) / (slice_y_fq0 + slice_y_fq1 + slice_y_fq2 + slice_y_fq3 +
slice_y_fq4)
index <- c(index,index_1)
}
return(index)
}
IM3 <- pp2index(X20180609_IM3)
IM2 <- pp2index(X20180609_IM2)
IM1 <- pp2index(X20180609_IM1)
WM1 <- pp2index(X20180609_WM1)
WM2 <- pp2index(X20180609_WM3)
WM3 <- pp2index(X20180609_WM2)
WI_M_Index <- cbind(WM1=WM1,WM2=WM2,WM3=WM3,IM1=IM1,IM2=IM2,IM3=IM3)
write.xlsx2(WI_M_Index, sheetName = "WI_M_Index", file='flyIndex.xlsx',append = TRUE)
]]>
- 爬虫
R语言
R语言
+ 批量
实例
- 爬虫
- 小说
+ 数据处理
+ 果蝇
diff --git a/sitemap.txt b/sitemap.txt
index 122d6539c..b12391853 100644
--- a/sitemap.txt
+++ b/sitemap.txt
@@ -36,8 +36,6 @@ https://leafminer.github.io/tags/R%E8%AF%AD%E8%A8%80/
https://leafminer.github.io/tags/%E6%89%B9%E9%87%8F/
https://leafminer.github.io/tags/%E8%A7%A3%E5%8E%8B/
https://leafminer.github.io/tags/%E5%AE%9E%E4%BE%8B/
-https://leafminer.github.io/tags/%E6%95%B0%E6%8D%AE%E5%A4%84%E7%90%86/
-https://leafminer.github.io/tags/%E6%9E%9C%E8%9D%87/
https://leafminer.github.io/tags/%E5%BB%BA%E7%AB%99/
https://leafminer.github.io/tags/API/
https://leafminer.github.io/tags/%E9%BB%98%E8%AE%A4/
@@ -52,19 +50,21 @@ https://leafminer.github.io/tags/%E9%98%85%E8%AF%BB/
https://leafminer.github.io/tags/%E6%96%B9%E6%B3%95/
https://leafminer.github.io/tags/%E5%85%B1%E6%8C%AF%E9%98%85%E8%AF%BB%E6%B3%95/
https://leafminer.github.io/tags/%E8%A1%8C%E5%8A%A8/
+https://leafminer.github.io/tags/%E7%88%AC%E8%99%AB/
+https://leafminer.github.io/tags/%E5%B0%8F%E8%AF%B4/
https://leafminer.github.io/tags/%E5%B1%80%E5%A4%96%E4%BA%BA/
https://leafminer.github.io/tags/%E9%9A%8F%E7%AC%94/
https://leafminer.github.io/tags/%E7%89%A2%E9%AA%9A/
https://leafminer.github.io/tags/%E6%97%B6%E4%BB%A3/
-https://leafminer.github.io/tags/%E8%85%BE%E8%AE%AF/
-https://leafminer.github.io/tags/%E5%A4%A7%E6%A8%A1%E5%9E%8B/
-https://leafminer.github.io/tags/%E5%85%83%E5%AE%9D/
https://leafminer.github.io/tags/STRING/
https://leafminer.github.io/tags/%E5%88%86%E6%9E%90/
https://leafminer.github.io/tags/PPI/
https://leafminer.github.io/tags/%E8%9B%8B%E7%99%BD%E4%BA%92%E4%BD%9C%E7%BD%91%E7%BB%9C/
-https://leafminer.github.io/tags/%E7%88%AC%E8%99%AB/
-https://leafminer.github.io/tags/%E5%B0%8F%E8%AF%B4/
+https://leafminer.github.io/tags/%E8%85%BE%E8%AE%AF/
+https://leafminer.github.io/tags/%E5%A4%A7%E6%A8%A1%E5%9E%8B/
+https://leafminer.github.io/tags/%E5%85%83%E5%AE%9D/
+https://leafminer.github.io/tags/%E6%95%B0%E6%8D%AE%E5%A4%84%E7%90%86/
+https://leafminer.github.io/tags/%E6%9E%9C%E8%9D%87/
https://leafminer.github.io/categories/%E7%AC%94%E8%AE%B0/
https://leafminer.github.io/categories/ImageJ/
https://leafminer.github.io/categories/%E7%94%9F%E4%BF%A1/
@@ -74,14 +74,14 @@ https://leafminer.github.io/categories/%E5%88%9D%E5%A7%8B/
https://leafminer.github.io/categories/%E7%AC%94%E8%AE%B0/%E5%BF%83%E7%90%86/
https://leafminer.github.io/categories/%E7%BF%BB%E8%AF%91/
https://leafminer.github.io/categories/%E9%98%85%E8%AF%BB/
+https://leafminer.github.io/categories/%E7%88%AC%E8%99%AB/
https://leafminer.github.io/categories/%E6%B4%BB%E7%9D%80/
https://leafminer.github.io/categories/%E5%BB%BA%E7%AB%99/%E7%AC%94%E8%AE%B0/
-https://leafminer.github.io/categories/%E6%96%87%E7%8C%AE%E9%98%85%E8%AF%BB/
-https://leafminer.github.io/categories/%E6%95%B0%E6%8D%AE%E5%88%86%E6%9E%90/
https://leafminer.github.io/categories/%E7%AC%94%E8%AE%B0/%E5%BF%83%E7%90%86/%E9%98%85%E8%AF%BB/
https://leafminer.github.io/categories/%E7%BF%BB%E8%AF%91/%E5%BF%83%E7%90%86/
https://leafminer.github.io/categories/%E9%98%85%E8%AF%BB/%E4%B9%A6%E6%91%98/
-https://leafminer.github.io/categories/%E6%96%87%E7%8C%AE%E9%98%85%E8%AF%BB/%E5%A4%A7%E6%A8%A1%E5%9E%8B/
-https://leafminer.github.io/categories/%E6%95%B0%E6%8D%AE%E5%88%86%E6%9E%90/%E7%94%9F%E7%89%A9/
-https://leafminer.github.io/categories/%E7%88%AC%E8%99%AB/
https://leafminer.github.io/categories/%E7%88%AC%E8%99%AB/R%E8%AF%AD%E8%A8%80/
+https://leafminer.github.io/categories/%E6%95%B0%E6%8D%AE%E5%88%86%E6%9E%90/
+https://leafminer.github.io/categories/%E6%96%87%E7%8C%AE%E9%98%85%E8%AF%BB/
+https://leafminer.github.io/categories/%E6%95%B0%E6%8D%AE%E5%88%86%E6%9E%90/%E7%94%9F%E7%89%A9/
+https://leafminer.github.io/categories/%E6%96%87%E7%8C%AE%E9%98%85%E8%AF%BB/%E5%A4%A7%E6%A8%A1%E5%9E%8B/
diff --git a/sitemap.xml b/sitemap.xml
index ae26ef1e9..2aa41eb0e 100644
--- a/sitemap.xml
+++ b/sitemap.xml
@@ -313,20 +313,6 @@
0.2
-
- https://leafminer.github.io/tags/%E6%95%B0%E6%8D%AE%E5%A4%84%E7%90%86/
- 2024-10-10
- weekly
- 0.2
-
-
-
- https://leafminer.github.io/tags/%E6%9E%9C%E8%9D%87/
- 2024-10-10
- weekly
- 0.2
-
-
https://leafminer.github.io/tags/%E5%BB%BA%E7%AB%99/
2024-10-10
@@ -425,6 +411,20 @@
0.2
+
+ https://leafminer.github.io/tags/%E7%88%AC%E8%99%AB/
+ 2024-10-10
+ weekly
+ 0.2
+
+
+
+ https://leafminer.github.io/tags/%E5%B0%8F%E8%AF%B4/
+ 2024-10-10
+ weekly
+ 0.2
+
+
https://leafminer.github.io/tags/%E5%B1%80%E5%A4%96%E4%BA%BA/
2024-10-10
@@ -454,63 +454,63 @@
- https://leafminer.github.io/tags/%E8%85%BE%E8%AE%AF/
+ https://leafminer.github.io/tags/STRING/
2024-10-10
weekly
0.2
- https://leafminer.github.io/tags/%E5%A4%A7%E6%A8%A1%E5%9E%8B/
+ https://leafminer.github.io/tags/%E5%88%86%E6%9E%90/
2024-10-10
weekly
0.2
- https://leafminer.github.io/tags/%E5%85%83%E5%AE%9D/
+ https://leafminer.github.io/tags/PPI/
2024-10-10
weekly
0.2
- https://leafminer.github.io/tags/STRING/
+ https://leafminer.github.io/tags/%E8%9B%8B%E7%99%BD%E4%BA%92%E4%BD%9C%E7%BD%91%E7%BB%9C/
2024-10-10
weekly
0.2
- https://leafminer.github.io/tags/%E5%88%86%E6%9E%90/
+ https://leafminer.github.io/tags/%E8%85%BE%E8%AE%AF/
2024-10-10
weekly
0.2
- https://leafminer.github.io/tags/PPI/
+ https://leafminer.github.io/tags/%E5%A4%A7%E6%A8%A1%E5%9E%8B/
2024-10-10
weekly
0.2
- https://leafminer.github.io/tags/%E8%9B%8B%E7%99%BD%E4%BA%92%E4%BD%9C%E7%BD%91%E7%BB%9C/
+ https://leafminer.github.io/tags/%E5%85%83%E5%AE%9D/
2024-10-10
weekly
0.2
- https://leafminer.github.io/tags/%E7%88%AC%E8%99%AB/
+ https://leafminer.github.io/tags/%E6%95%B0%E6%8D%AE%E5%A4%84%E7%90%86/
2024-10-10
weekly
0.2
- https://leafminer.github.io/tags/%E5%B0%8F%E8%AF%B4/
+ https://leafminer.github.io/tags/%E6%9E%9C%E8%9D%87/
2024-10-10
weekly
0.2
@@ -582,77 +582,77 @@
- https://leafminer.github.io/categories/%E6%B4%BB%E7%9D%80/
+ https://leafminer.github.io/categories/%E7%88%AC%E8%99%AB/
2024-10-10
weekly
0.2
- https://leafminer.github.io/categories/%E5%BB%BA%E7%AB%99/%E7%AC%94%E8%AE%B0/
+ https://leafminer.github.io/categories/%E6%B4%BB%E7%9D%80/
2024-10-10
weekly
0.2
- https://leafminer.github.io/categories/%E6%96%87%E7%8C%AE%E9%98%85%E8%AF%BB/
+ https://leafminer.github.io/categories/%E5%BB%BA%E7%AB%99/%E7%AC%94%E8%AE%B0/
2024-10-10
weekly
0.2
- https://leafminer.github.io/categories/%E6%95%B0%E6%8D%AE%E5%88%86%E6%9E%90/
+ https://leafminer.github.io/categories/%E7%AC%94%E8%AE%B0/%E5%BF%83%E7%90%86/%E9%98%85%E8%AF%BB/
2024-10-10
weekly
0.2
- https://leafminer.github.io/categories/%E7%AC%94%E8%AE%B0/%E5%BF%83%E7%90%86/%E9%98%85%E8%AF%BB/
+ https://leafminer.github.io/categories/%E7%BF%BB%E8%AF%91/%E5%BF%83%E7%90%86/
2024-10-10
weekly
0.2
- https://leafminer.github.io/categories/%E7%BF%BB%E8%AF%91/%E5%BF%83%E7%90%86/
+ https://leafminer.github.io/categories/%E9%98%85%E8%AF%BB/%E4%B9%A6%E6%91%98/
2024-10-10
weekly
0.2
- https://leafminer.github.io/categories/%E9%98%85%E8%AF%BB/%E4%B9%A6%E6%91%98/
+ https://leafminer.github.io/categories/%E7%88%AC%E8%99%AB/R%E8%AF%AD%E8%A8%80/
2024-10-10
weekly
0.2
- https://leafminer.github.io/categories/%E6%96%87%E7%8C%AE%E9%98%85%E8%AF%BB/%E5%A4%A7%E6%A8%A1%E5%9E%8B/
+ https://leafminer.github.io/categories/%E6%95%B0%E6%8D%AE%E5%88%86%E6%9E%90/
2024-10-10
weekly
0.2
- https://leafminer.github.io/categories/%E6%95%B0%E6%8D%AE%E5%88%86%E6%9E%90/%E7%94%9F%E7%89%A9/
+ https://leafminer.github.io/categories/%E6%96%87%E7%8C%AE%E9%98%85%E8%AF%BB/
2024-10-10
weekly
0.2
- https://leafminer.github.io/categories/%E7%88%AC%E8%99%AB/
+ https://leafminer.github.io/categories/%E6%95%B0%E6%8D%AE%E5%88%86%E6%9E%90/%E7%94%9F%E7%89%A9/
2024-10-10
weekly
0.2
- https://leafminer.github.io/categories/%E7%88%AC%E8%99%AB/R%E8%AF%AD%E8%A8%80/
+ https://leafminer.github.io/categories/%E6%96%87%E7%8C%AE%E9%98%85%E8%AF%BB/%E5%A4%A7%E6%A8%A1%E5%9E%8B/
2024-10-10
weekly
0.2
diff --git a/tags/API/index.html b/tags/API/index.html
index 0eabe1a01..a6ce92b3c 100644
--- a/tags/API/index.html
+++ b/tags/API/index.html
@@ -179,12 +179,21 @@
+
+
+
+
+
+
+
diff --git a/tags/Hexo/index.html b/tags/Hexo/index.html
index 53d9bcfc0..b7dd89e45 100644
--- a/tags/Hexo/index.html
+++ b/tags/Hexo/index.html
@@ -179,12 +179,21 @@
+
+
+
+
+
+
+
diff --git a/tags/ImageJ/index.html b/tags/ImageJ/index.html
index ea8a69406..4336640f7 100644
--- a/tags/ImageJ/index.html
+++ b/tags/ImageJ/index.html
@@ -179,12 +179,21 @@
+
+
+
+
+
+
+
diff --git a/tags/PPI/index.html b/tags/PPI/index.html
index 64103a404..85c651f75 100644
--- a/tags/PPI/index.html
+++ b/tags/PPI/index.html
@@ -179,12 +179,21 @@
+
+
+
+
+
+
+
diff --git a/tags/RNA-seq/index.html b/tags/RNA-seq/index.html
index 21c44e8be..478aa71db 100644
--- a/tags/RNA-seq/index.html
+++ b/tags/RNA-seq/index.html
@@ -179,12 +179,21 @@
+
+
+
+
+
+
+
diff --git "a/tags/R\350\257\255\350\250\200/index.html" "b/tags/R\350\257\255\350\250\200/index.html"
index e6897e494..c05a15306 100644
--- "a/tags/R\350\257\255\350\250\200/index.html"
+++ "b/tags/R\350\257\255\350\250\200/index.html"
@@ -179,12 +179,21 @@
+
+
+
+
+
+
+
diff --git a/tags/STRING/index.html b/tags/STRING/index.html
index 3dc196766..6d8cced76 100644
--- a/tags/STRING/index.html
+++ b/tags/STRING/index.html
@@ -179,12 +179,21 @@
+
+
+
+
+
+
+
diff --git a/tags/index.html b/tags/index.html
index 5ab90c2b9..1bcb4704f 100644
--- a/tags/index.html
+++ b/tags/index.html
@@ -180,12 +180,21 @@
+
+
+
+
+
+
+
diff --git "a/tags/\344\275\240\345\245\275/index.html" "b/tags/\344\275\240\345\245\275/index.html"
index 8492d5b69..72c88d7ba 100644
--- "a/tags/\344\275\240\345\245\275/index.html"
+++ "b/tags/\344\275\240\345\245\275/index.html"
@@ -179,12 +179,21 @@
+
+
+
+
+
+
+
diff --git "a/tags/\345\205\203\345\256\235/index.html" "b/tags/\345\205\203\345\256\235/index.html"
index 93476c2d5..c042ff3e8 100644
--- "a/tags/\345\205\203\345\256\235/index.html"
+++ "b/tags/\345\205\203\345\256\235/index.html"
@@ -179,12 +179,21 @@
+
+
+
+
+
+
+
diff --git "a/tags/\345\205\261\346\214\257\351\230\205\350\257\273\346\263\225/index.html" "b/tags/\345\205\261\346\214\257\351\230\205\350\257\273\346\263\225/index.html"
index b64736699..4a58dbc5f 100644
--- "a/tags/\345\205\261\346\214\257\351\230\205\350\257\273\346\263\225/index.html"
+++ "b/tags/\345\205\261\346\214\257\351\230\205\350\257\273\346\263\225/index.html"
@@ -179,12 +179,21 @@
+
+
+
+
+
+
+
diff --git "a/tags/\345\210\206\346\236\220/index.html" "b/tags/\345\210\206\346\236\220/index.html"
index 38cf04fe4..8fc99b32e 100644
--- "a/tags/\345\210\206\346\236\220/index.html"
+++ "b/tags/\345\210\206\346\236\220/index.html"
@@ -179,12 +179,21 @@
+
+
+
+
+
+
+
diff --git "a/tags/\345\215\232\345\256\242/index.html" "b/tags/\345\215\232\345\256\242/index.html"
index 8eac29a21..f6dfdb3ee 100644
--- "a/tags/\345\215\232\345\256\242/index.html"
+++ "b/tags/\345\215\232\345\256\242/index.html"
@@ -179,12 +179,21 @@
+
+
+
+
+
+
+
diff --git "a/tags/\345\233\276\345\203\217/index.html" "b/tags/\345\233\276\345\203\217/index.html"
index a5660a09e..fd437687e 100644
--- "a/tags/\345\233\276\345\203\217/index.html"
+++ "b/tags/\345\233\276\345\203\217/index.html"
@@ -179,12 +179,21 @@
+
+
+
+
+
+
+
diff --git "a/tags/\345\244\247\346\250\241\345\236\213/index.html" "b/tags/\345\244\247\346\250\241\345\236\213/index.html"
index b1a8e0986..309840710 100644
--- "a/tags/\345\244\247\346\250\241\345\236\213/index.html"
+++ "b/tags/\345\244\247\346\250\241\345\236\213/index.html"
@@ -179,12 +179,21 @@
+
+
+
+
+
+
+
diff --git "a/tags/\345\256\236\344\276\213/index.html" "b/tags/\345\256\236\344\276\213/index.html"
index 6af5c1ba0..714557004 100644
--- "a/tags/\345\256\236\344\276\213/index.html"
+++ "b/tags/\345\256\236\344\276\213/index.html"
@@ -179,12 +179,21 @@
+
+
+
+
+
+
+
diff --git "a/tags/\345\260\217\350\257\264/index.html" "b/tags/\345\260\217\350\257\264/index.html"
index b4cd810f5..32dc3d39c 100644
--- "a/tags/\345\260\217\350\257\264/index.html"
+++ "b/tags/\345\260\217\350\257\264/index.html"
@@ -179,12 +179,21 @@
+
+
+
+
+
+
+
diff --git "a/tags/\345\261\200\345\244\226\344\272\272/index.html" "b/tags/\345\261\200\345\244\226\344\272\272/index.html"
index 5d5829cba..3fb80cf68 100644
--- "a/tags/\345\261\200\345\244\226\344\272\272/index.html"
+++ "b/tags/\345\261\200\345\244\226\344\272\272/index.html"
@@ -179,12 +179,21 @@
+
+
+
+
+
+
+
diff --git "a/tags/\345\273\272\347\253\231/index.html" "b/tags/\345\273\272\347\253\231/index.html"
index c126d70f1..5a67f8d53 100644
--- "a/tags/\345\273\272\347\253\231/index.html"
+++ "b/tags/\345\273\272\347\253\231/index.html"
@@ -179,12 +179,21 @@
+
+
+
+
+
+
+
diff --git "a/tags/\345\277\203\347\220\206/index.html" "b/tags/\345\277\203\347\220\206/index.html"
index eb28b960b..784b12317 100644
--- "a/tags/\345\277\203\347\220\206/index.html"
+++ "b/tags/\345\277\203\347\220\206/index.html"
@@ -179,12 +179,21 @@
+
+
+
+
+
+
+
diff --git "a/tags/\346\203\205\350\257\227/index.html" "b/tags/\346\203\205\350\257\227/index.html"
index 55a2c2338..2f24090dd 100644
--- "a/tags/\346\203\205\350\257\227/index.html"
+++ "b/tags/\346\203\205\350\257\227/index.html"
@@ -179,12 +179,21 @@
+
+
+
+
+
+
+
diff --git "a/tags/\346\211\271\351\207\217/index.html" "b/tags/\346\211\271\351\207\217/index.html"
index fa96b8088..b311653cf 100644
--- "a/tags/\346\211\271\351\207\217/index.html"
+++ "b/tags/\346\211\271\351\207\217/index.html"
@@ -179,12 +179,21 @@
+
+
+
+
+
+
+
diff --git "a/tags/\346\214\207\344\273\244/index.html" "b/tags/\346\214\207\344\273\244/index.html"
index a80a39595..639ac606d 100644
--- "a/tags/\346\214\207\344\273\244/index.html"
+++ "b/tags/\346\214\207\344\273\244/index.html"
@@ -179,12 +179,21 @@
+
+
+
+
+
+
+
diff --git "a/tags/\346\217\222\344\273\266/index.html" "b/tags/\346\217\222\344\273\266/index.html"
index a7f1f55d0..083c4d9b9 100644
--- "a/tags/\346\217\222\344\273\266/index.html"
+++ "b/tags/\346\217\222\344\273\266/index.html"
@@ -179,12 +179,21 @@
+
+
+
+
+
+
+
diff --git "a/tags/\346\225\210\345\272\224/index.html" "b/tags/\346\225\210\345\272\224/index.html"
index 9b41b075c..9a0dd478d 100644
--- "a/tags/\346\225\210\345\272\224/index.html"
+++ "b/tags/\346\225\210\345\272\224/index.html"
@@ -179,12 +179,21 @@
+
+
+
+
+
+
+
diff --git "a/tags/\346\225\260\346\215\256\345\244\204\347\220\206/index.html" "b/tags/\346\225\260\346\215\256\345\244\204\347\220\206/index.html"
index 1d1c1a012..7fd5c7d4c 100644
--- "a/tags/\346\225\260\346\215\256\345\244\204\347\220\206/index.html"
+++ "b/tags/\346\225\260\346\215\256\345\244\204\347\220\206/index.html"
@@ -179,12 +179,21 @@
+
+
+
+
+
+
+
diff --git "a/tags/\346\226\271\346\263\225/index.html" "b/tags/\346\226\271\346\263\225/index.html"
index e30b33090..355c8e238 100644
--- "a/tags/\346\226\271\346\263\225/index.html"
+++ "b/tags/\346\226\271\346\263\225/index.html"
@@ -179,12 +179,21 @@
+
+
+
+
+
+
+
diff --git "a/tags/\346\227\266\344\273\243/index.html" "b/tags/\346\227\266\344\273\243/index.html"
index ab21a0610..fb29933d1 100644
--- "a/tags/\346\227\266\344\273\243/index.html"
+++ "b/tags/\346\227\266\344\273\243/index.html"
@@ -179,12 +179,21 @@
+
+
+
+
+
+
+
diff --git "a/tags/\346\236\234\350\235\207/index.html" "b/tags/\346\236\234\350\235\207/index.html"
index 8c660ec70..60de14dbf 100644
--- "a/tags/\346\236\234\350\235\207/index.html"
+++ "b/tags/\346\236\234\350\235\207/index.html"
@@ -179,12 +179,21 @@
+
+
+
+
+
+
+
diff --git "a/tags/\347\210\254\350\231\253/index.html" "b/tags/\347\210\254\350\231\253/index.html"
index 1d01fb465..358431c5b 100644
--- "a/tags/\347\210\254\350\231\253/index.html"
+++ "b/tags/\347\210\254\350\231\253/index.html"
@@ -179,12 +179,21 @@
+
+
+
+
+
+
+
diff --git "a/tags/\347\211\242\351\252\232/index.html" "b/tags/\347\211\242\351\252\232/index.html"
index d96d7d03d..4473e01f8 100644
--- "a/tags/\347\211\242\351\252\232/index.html"
+++ "b/tags/\347\211\242\351\252\232/index.html"
@@ -179,12 +179,21 @@
+
+
+
+
+
+
+
diff --git "a/tags/\347\224\237\344\277\241/index.html" "b/tags/\347\224\237\344\277\241/index.html"
index 25f8af46f..1f2401b81 100644
--- "a/tags/\347\224\237\344\277\241/index.html"
+++ "b/tags/\347\224\237\344\277\241/index.html"
@@ -179,12 +179,21 @@
+
+
+
+
+
+
+
diff --git "a/tags/\347\226\276\347\227\205/index.html" "b/tags/\347\226\276\347\227\205/index.html"
index 8ce1e043b..9bb4a6488 100644
--- "a/tags/\347\226\276\347\227\205/index.html"
+++ "b/tags/\347\226\276\347\227\205/index.html"
@@ -179,12 +179,21 @@
+
+
+
+
+
+
+
diff --git "a/tags/\347\254\224\350\256\260/index.html" "b/tags/\347\254\224\350\256\260/index.html"
index 17ad8db53..7a5037b81 100644
--- "a/tags/\347\254\224\350\256\260/index.html"
+++ "b/tags/\347\254\224\350\256\260/index.html"
@@ -179,12 +179,21 @@
+
+
+
+
+
+
+
diff --git "a/tags/\347\256\200\344\273\213/index.html" "b/tags/\347\256\200\344\273\213/index.html"
index 329c166ce..ad7b9654b 100644
--- "a/tags/\347\256\200\344\273\213/index.html"
+++ "b/tags/\347\256\200\344\273\213/index.html"
@@ -179,12 +179,21 @@
+
+
+
+
+
+
+
diff --git "a/tags/\347\256\227\346\263\225/index.html" "b/tags/\347\256\227\346\263\225/index.html"
index 61ff26bfb..f22cb62ce 100644
--- "a/tags/\347\256\227\346\263\225/index.html"
+++ "b/tags/\347\256\227\346\263\225/index.html"
@@ -179,12 +179,21 @@
+
+
+
+
+
+
+
diff --git "a/tags/\347\275\221\347\273\234/index.html" "b/tags/\347\275\221\347\273\234/index.html"
index 5c94987ca..df72045ae 100644
--- "a/tags/\347\275\221\347\273\234/index.html"
+++ "b/tags/\347\275\221\347\273\234/index.html"
@@ -179,12 +179,21 @@
+
+
+
+
+
+
+
diff --git "a/tags/\350\205\276\350\256\257/index.html" "b/tags/\350\205\276\350\256\257/index.html"
index 6ea6b624c..9869769aa 100644
--- "a/tags/\350\205\276\350\256\257/index.html"
+++ "b/tags/\350\205\276\350\256\257/index.html"
@@ -179,12 +179,21 @@
+
+
+
+
+
+
+
diff --git "a/tags/\350\233\213\347\231\275\344\272\222\344\275\234\347\275\221\347\273\234/index.html" "b/tags/\350\233\213\347\231\275\344\272\222\344\275\234\347\275\221\347\273\234/index.html"
index a6dfcfc21..1ecdcb104 100644
--- "a/tags/\350\233\213\347\231\275\344\272\222\344\275\234\347\275\221\347\273\234/index.html"
+++ "b/tags/\350\233\213\347\231\275\344\272\222\344\275\234\347\275\221\347\273\234/index.html"
@@ -179,12 +179,21 @@
+
+
+
+
+
+
+
diff --git "a/tags/\350\241\214\345\212\250/index.html" "b/tags/\350\241\214\345\212\250/index.html"
index 0d0653a56..6f6366ccc 100644
--- "a/tags/\350\241\214\345\212\250/index.html"
+++ "b/tags/\350\241\214\345\212\250/index.html"
@@ -179,12 +179,21 @@
+
+
+
+
+
+
+
diff --git "a/tags/\350\247\243\345\216\213/index.html" "b/tags/\350\247\243\345\216\213/index.html"
index 896f88545..7a803f370 100644
--- "a/tags/\350\247\243\345\216\213/index.html"
+++ "b/tags/\350\247\243\345\216\213/index.html"
@@ -179,12 +179,21 @@
+
+
+
+
+
+
+
diff --git "a/tags/\351\230\205\350\257\273/index.html" "b/tags/\351\230\205\350\257\273/index.html"
index ac31ee831..761ee251f 100644
--- "a/tags/\351\230\205\350\257\273/index.html"
+++ "b/tags/\351\230\205\350\257\273/index.html"
@@ -179,12 +179,21 @@
+
+
+
+
+
+
+
diff --git "a/tags/\351\231\215\345\231\252/index.html" "b/tags/\351\231\215\345\231\252/index.html"
index 62268ff12..b226cdf12 100644
--- "a/tags/\351\231\215\345\231\252/index.html"
+++ "b/tags/\351\231\215\345\231\252/index.html"
@@ -179,12 +179,21 @@
+
+
+
+
+
+
+
diff --git "a/tags/\351\232\217\347\254\224/index.html" "b/tags/\351\232\217\347\254\224/index.html"
index 51c093b43..773d385e0 100644
--- "a/tags/\351\232\217\347\254\224/index.html"
+++ "b/tags/\351\232\217\347\254\224/index.html"
@@ -179,12 +179,21 @@
+
+
+
+
+
+
+
diff --git "a/tags/\351\273\230\350\256\244/index.html" "b/tags/\351\273\230\350\256\244/index.html"
index b86587fe8..e1b57f95c 100644
--- "a/tags/\351\273\230\350\256\244/index.html"
+++ "b/tags/\351\273\230\350\256\244/index.html"
@@ -179,12 +179,21 @@
+
+
+
+
+
+
+
简介
-腾讯元宝的深度阅读模式是一种创新的阅读方式,它利用人工智能技术,为用户提供了一种全新的长文阅读体验。这种模式不仅能够处理大量文本,还能生成图文并茂的内容,极大地提升了阅读效率和理解深度。
+蛋白互作网络(PPI, Protein-Protein Interaction Networks)可以查看基因之间的联系,进而锁定关键基因。一般使用STRING数据库获取蛋白互作信息。
@@ -458,7 +466,7 @@
-
+
@@ -476,7 +484,7 @@
- 蛋白互作网络PPI分析流程示例
+ 大模型读文献之腾讯元宝--深度阅读
@@ -529,10 +537,11 @@
- 蛋白互作网络(PPI, Protein-Protein Interaction Networks)可以查看基因之间的联系,进而锁定关键基因。一般使用STRING数据库获取蛋白互作信息。
+ 简介
+腾讯元宝的深度阅读模式是一种创新的阅读方式,它利用人工智能技术,为用户提供了一种全新的长文阅读体验。这种模式不仅能够处理大量文本,还能生成图文并茂的内容,极大地提升了阅读效率和理解深度。
diff --git a/page/2/index.html b/page/2/index.html
index ad1aa3da9..ea201e488 100644
--- a/page/2/index.html
+++ b/page/2/index.html
@@ -179,12 +179,21 @@ 损不足
+
- 蛋白互作网络PPI分析流程示例 + 大模型读文献之腾讯元宝--深度阅读
@@ -529,10 +537,11 @@
- 蛋白互作网络(PPI, Protein-Protein Interaction Networks)可以查看基因之间的联系,进而锁定关键基因。一般使用STRING数据库获取蛋白互作信息。
+ 简介
+腾讯元宝的深度阅读模式是一种创新的阅读方式,它利用人工智能技术,为用户提供了一种全新的长文阅读体验。这种模式不仅能够处理大量文本,还能生成图文并茂的内容,极大地提升了阅读效率和理解深度。
diff --git a/page/2/index.html b/page/2/index.html
index ad1aa3da9..ea201e488 100644
--- a/page/2/index.html
+++ b/page/2/index.html
@@ -179,12 +179,21 @@ 损不足
+
蛋白互作网络(PPI, Protein-Protein Interaction Networks)可以查看基因之间的联系,进而锁定关键基因。一般使用STRING数据库获取蛋白互作信息。
+简介
+腾讯元宝的深度阅读模式是一种创新的阅读方式,它利用人工智能技术,为用户提供了一种全新的长文阅读体验。这种模式不仅能够处理大量文本,还能生成图文并茂的内容,极大地提升了阅读效率和理解深度。
diff --git a/page/2/index.html b/page/2/index.html index ad1aa3da9..ea201e488 100644 --- a/page/2/index.html +++ b/page/2/index.html @@ -179,12 +179,21 @@损不足
++ +

+ +

+ +

简介
-StackReg,用于递归对齐图像堆栈(stack)的 ImageJ 插件。
作者: Philippe Thévenaz, Biomedical Imaging Group, Swiss Federal Institute of Technology Lausanne
该插件的目的是配准(换句话说,对齐或匹配)图像堆栈。每张图片用作下一张图片对齐的模板,以便通过转换进行对齐。当插件启动时,当前图片充当全局锚点。StackReg 插件要求安装有TurboReg 插件。该插件(StackReg)是TurboReg 插件的前端。这些插件之间有几种数据交换机制,其中之一是将临时文件写入 ImageJ 定义的临时目录。该目录的位置可以通过宏命令 "print(getDirectory("temp"));
"查看。文件名称是:StackRegSource,StackRegSourceR,StackRegSourceG,StackRegSourceB,StackRegTarget。
配准完成后,原始堆栈将被销毁,取而代之的是配准结果。堆栈的名称和类型保持不变;除 RGB 堆栈和 HSB 堆栈外,所有数据类型均可使用。(请注意,RGB-stack 是由三个颜色分量组成的堆栈,不应与 RGB 彩色图像堆栈混淆:后者可以使用,而前者不能使用。如有疑问,请尝试一下)。如果提供的是彩色图像堆栈,则会在灰度版本上进行配准,灰度版本是由红色、绿色和蓝色分量加权求和而成的,即使堆栈是伪彩色图像也是如此。权重取决于整个图像堆栈,并通过主成分分析确定,以提供最大可能的对比度。一旦根据中间灰度表示确定了几何图形,就可以重建真正的彩色图像,如果需要生成伪彩色图像,还可以重新量化。
- -示例
-
原始图
配准后(转换类型Scaled rotation)
参数
-有四种类型的转换:
--
-
-
-
Translation:
-
-
映射公式为 。其中,输入坐标 u 映射到输出坐标 x,常数矢量 Δu 表示平移量。
-
-
-
-
Rigid Body:
-
-
映射公式为
由于存在 Δu 项,因此除了旋转角度 θ 外,还考虑了平移。
-
-
-
-
Scaled rotation:
-
-
映射公式为
与Rigid Body 的区别在于存在标量调整因子 λ。
-
-
-
-
Affine:
-
-
映射公式为
由于四个系数 、
、
和
是独立的,因此仿射变换比按比例旋转具有更多的自由度。
-
操作步骤
-使用的Fiji ImageJ
--
-
-
-
拖动图片文件夹到Fiji,默认为堆栈。
-
-
-
-
-
-
插件启动前,选择合适的图片,当前图片会被充当全局锚点。
-
- -
-
打开StackReg。
-
-
-
-
-
-
选择对齐方式,点击确定按钮。
-
-
配准结果展示
-Translation
Rigid Body
Scaled rotation
Affine
-
脚本
-StackReg 是可编写脚本和使用宏记录的。下面是一个从宏调用 StackReg 的示例:
-run("StackReg ", "transformation=[Rigid Body]"); |
参考
--
-
-
-
https://bigwww.epfl.ch/thevenaz/stackreg/
-
- -
-
P. Thévenaz, U.E. Ruttimann, M. Unser, “A Pyramid Approach to Subpixel Registration Based on Intensity,” IEEE Transactions on Image Processing, vol. 7, no. 1, pp. 27-41, January 1998. Other relevant on-line publications are available at http://bigwww.epfl.ch/publications/.
-
-
目的
-处理ImageJ测量的果蝇攀爬数据
- -实例
-library(cluster) |
简介
+StackReg,用于递归对齐图像堆栈(stack)的 ImageJ 插件。
作者: Philippe Thévenaz, Biomedical Imaging Group, Swiss Federal Institute of Technology Lausanne
该插件的目的是配准(换句话说,对齐或匹配)图像堆栈。每张图片用作下一张图片对齐的模板,以便通过转换进行对齐。当插件启动时,当前图片充当全局锚点。StackReg 插件要求安装有TurboReg 插件。该插件(StackReg)是TurboReg 插件的前端。这些插件之间有几种数据交换机制,其中之一是将临时文件写入 ImageJ 定义的临时目录。该目录的位置可以通过宏命令 "print(getDirectory("temp"));
"查看。文件名称是:StackRegSource,StackRegSourceR,StackRegSourceG,StackRegSourceB,StackRegTarget。
配准完成后,原始堆栈将被销毁,取而代之的是配准结果。堆栈的名称和类型保持不变;除 RGB 堆栈和 HSB 堆栈外,所有数据类型均可使用。(请注意,RGB-stack 是由三个颜色分量组成的堆栈,不应与 RGB 彩色图像堆栈混淆:后者可以使用,而前者不能使用。如有疑问,请尝试一下)。如果提供的是彩色图像堆栈,则会在灰度版本上进行配准,灰度版本是由红色、绿色和蓝色分量加权求和而成的,即使堆栈是伪彩色图像也是如此。权重取决于整个图像堆栈,并通过主成分分析确定,以提供最大可能的对比度。一旦根据中间灰度表示确定了几何图形,就可以重建真正的彩色图像,如果需要生成伪彩色图像,还可以重新量化。
+ +示例
+
原始图
配准后(转换类型Scaled rotation)
参数
+有四种类型的转换:
+-
+
-
+
Translation:
+
+
映射公式为 。其中,输入坐标 u 映射到输出坐标 x,常数矢量 Δu 表示平移量。
-
+
-
+
Rigid Body:
+
+
映射公式为
由于存在 Δu 项,因此除了旋转角度 θ 外,还考虑了平移。
-
+
-
+
Scaled rotation:
+
+
映射公式为
与Rigid Body 的区别在于存在标量调整因子 λ。
-
+
-
+
Affine:
+
+
映射公式为
由于四个系数 、
、
和
是独立的,因此仿射变换比按比例旋转具有更多的自由度。
+
操作步骤
+使用的Fiji ImageJ
+-
+
-
+
拖动图片文件夹到Fiji,默认为堆栈。
+
+
-
+
-
+
插件启动前,选择合适的图片,当前图片会被充当全局锚点。
+
+ -
+
打开StackReg。
+
+
-
+
-
+
选择对齐方式,点击确定按钮。
+
+
配准结果展示
+Translation
Rigid Body
Scaled rotation
Affine
+
脚本
+StackReg 是可编写脚本和使用宏记录的。下面是一个从宏调用 StackReg 的示例:
+run("StackReg ", "transformation=[Rigid Body]"); |
参考
+-
+
-
+
https://bigwww.epfl.ch/thevenaz/stackreg/
+
+ -
+
P. Thévenaz, U.E. Ruttimann, M. Unser, “A Pyramid Approach to Subpixel Registration Based on Intensity,” IEEE Transactions on Image Processing, vol. 7, no. 1, pp. 27-41, January 1998. Other relevant on-line publications are available at http://bigwww.epfl.ch/publications/.
+
+
SimpleITK 是 Insight 工具包(ITK)算法和数据结构的简化编程接口。它支持多种编程语言的接口,包括 C++、Python、R、Java、C#、Lua、Ruby 和 TCL。这些绑定使科学家能够用他们最熟悉的编程语言开发图像分析工作流。该工具包支持 15 种以上不同的图像文件格式,提供 280 多种图像分析过滤器,并为 ITK 基于强度的配准框架实现了统一接口。
+ +特点
+使用 Python、R、Java、C#、Lua、Ruby、TCL 和 C++ 进行开源多维图像分析。由 Insight 工具包社区为生物医学科学及其他领域开发。
+-
+
- 被视为空间对象而非像素阵列的图像。计算在二维或三维物理空间中进行。 +
- 利用刚性或可变形变换快速配准二维和三维模内和模间图像的配准框架。 +
- 从经典的Otsu thresholding到水平集(level sets) 和分水岭(watersheds),为图像分割工作流程提供了丰富的过滤器。 +
- 评估分割结果(豪斯多夫距离、雅卡德值和骰子值、表面距离等<Hausdorff distance, Jaccard and Dice values, surface distances etc.> )和分析分割形状特征(定向边界框、主矩、周长、伸长率、费雷特直径等<oriented bounding box, principal moments, perimeter, elongation, Feret diameter etc.>)的工具。 +
- 图像文件输入/输出,支持 20 多种图像文件格式(jpg、png、DICOM、TIFF 等),并可在不同格式间轻松转换。 +
- 通过基于进程和线程的并行化,可随时集成到集群或台式机的并行处理框架中。 +
安装R包-SimpleITKRInstaller
+devtools::install_github("SimpleITK/SimpleITKRInstaller")
Fiji
+SimpleITK 有一个内置函数 itk::simple::Show()
,可用于在交互会话中查看图像。默认情况下,该 Show 函数会搜索已安装的 Fiji 来显示图像。如果找不到 Fiji,则会搜索 ImageJ。选择 Fiji/ImageJ 是因为它们可以处理 SimpleITK 支持的所有图像类型,包括每像素有 n 个分量的 3D 矢量图像。 Show 函数会在多个标准位置搜索 Fiji/ImageJ。具体位置取决于系统类型(Linux、Mac 或 Windows)。启用 "Show "的 "debugOn "选项后,用户可以看到 SimpleITK 正在搜索 Fiji(或其他查看应用程序)的位置,以及用于启动查看应用程序的实际命令。 Show 是 ImageViewer 类的功能接口。可以使用 ImageViewer 对象配置其他查看应用程序.
+
图片导入和导出
+library(SimpleITK) |
上面的示例指定使用 PNGImageIO 来读取文件。如果省略该行,SimpleITK 将根据文件名的后缀和/或文件头自动确定要使用哪个 IO。
+image <- ReadImage(inputImageFileName) |
SimpleITK 是 Insight 工具包(ITK)算法和数据结构的简化编程接口。它支持多种编程语言的接口,包括 C++、Python、R、Java、C#、Lua、Ruby 和 TCL。这些绑定使科学家能够用他们最熟悉的编程语言开发图像分析工作流。该工具包支持 15 种以上不同的图像文件格式,提供 280 多种图像分析过滤器,并为 ITK 基于强度的配准框架实现了统一接口。
- -特点
-使用 Python、R、Java、C#、Lua、Ruby、TCL 和 C++ 进行开源多维图像分析。由 Insight 工具包社区为生物医学科学及其他领域开发。
--
-
- 被视为空间对象而非像素阵列的图像。计算在二维或三维物理空间中进行。 -
- 利用刚性或可变形变换快速配准二维和三维模内和模间图像的配准框架。 -
- 从经典的Otsu thresholding到水平集(level sets) 和分水岭(watersheds),为图像分割工作流程提供了丰富的过滤器。 -
- 评估分割结果(豪斯多夫距离、雅卡德值和骰子值、表面距离等<Hausdorff distance, Jaccard and Dice values, surface distances etc.> )和分析分割形状特征(定向边界框、主矩、周长、伸长率、费雷特直径等<oriented bounding box, principal moments, perimeter, elongation, Feret diameter etc.>)的工具。 -
- 图像文件输入/输出,支持 20 多种图像文件格式(jpg、png、DICOM、TIFF 等),并可在不同格式间轻松转换。 -
- 通过基于进程和线程的并行化,可随时集成到集群或台式机的并行处理框架中。 -
安装R包-SimpleITKRInstaller
-devtools::install_github("SimpleITK/SimpleITKRInstaller")
Fiji
-SimpleITK 有一个内置函数 itk::simple::Show()
,可用于在交互会话中查看图像。默认情况下,该 Show 函数会搜索已安装的 Fiji 来显示图像。如果找不到 Fiji,则会搜索 ImageJ。选择 Fiji/ImageJ 是因为它们可以处理 SimpleITK 支持的所有图像类型,包括每像素有 n 个分量的 3D 矢量图像。 Show 函数会在多个标准位置搜索 Fiji/ImageJ。具体位置取决于系统类型(Linux、Mac 或 Windows)。启用 "Show "的 "debugOn "选项后,用户可以看到 SimpleITK 正在搜索 Fiji(或其他查看应用程序)的位置,以及用于启动查看应用程序的实际命令。 Show 是 ImageViewer 类的功能接口。可以使用 ImageViewer 对象配置其他查看应用程序.
-
图片导入和导出
-library(SimpleITK) |
上面的示例指定使用 PNGImageIO 来读取文件。如果省略该行,SimpleITK 将根据文件名的后缀和/或文件头自动确定要使用哪个 IO。
-image <- ReadImage(inputImageFileName) |
目的
+小说离线阅读,保存。练习R语言。示例网站:https://www.-----.com/yuedu/15111/
+ +实例
+library("stringr") |
简介
-腾讯元宝的深度阅读模式是一种创新的阅读方式,它利用人工智能技术,为用户提供了一种全新的长文阅读体验。这种模式不仅能够处理大量文本,还能生成图文并茂的内容,极大地提升了阅读效率和理解深度。
- -深度阅读模式的特点
----
-- 长文阅读支持:深度阅读模式支持长达近50万字的专业文档,适用于学术研究、市场分析、财务报告等领域。
-- 图文并茂的内容重塑:通过多模态内容生成技术,深度阅读模式能够展示原始插图或通过算法生成的高级分析图表,使信息传达更加直观。
-- 智慧导航:配备划词搜索和翻译功能,帮助用户无缝理解专业术语,特别适用于跨语言研究。
-- 专业图表的智能生成:针对财报和研究报告,能够自动识别关键数据,生成杜邦分析图等专业图表,并结合内置的计算器功能确保数值的精准计算。
-
-
示例
--
-
-
-
打开元宝 (https://yuanbao.tencent.com/) ,需要登录使用。界面很简洁,点击输入框上面的“深度阅读”
-
-
弹出文件上传框,拖动pdf到上传框。
--
-
-
-
阅读界面如下,可以看到总结、精度、翻译、脑图功能。还可以直接根据文献内容生成学术海报。所有生成结果均可下载。下载格式为图片和pdf,生成的pdf对公示显示不友好。
-
-
-
-
-
-
文献阅读部分结果展示:
-
-
简介
+腾讯元宝的深度阅读模式是一种创新的阅读方式,它利用人工智能技术,为用户提供了一种全新的长文阅读体验。这种模式不仅能够处理大量文本,还能生成图文并茂的内容,极大地提升了阅读效率和理解深度。
+ +深度阅读模式的特点
++++
+- 长文阅读支持:深度阅读模式支持长达近50万字的专业文档,适用于学术研究、市场分析、财务报告等领域。
+- 图文并茂的内容重塑:通过多模态内容生成技术,深度阅读模式能够展示原始插图或通过算法生成的高级分析图表,使信息传达更加直观。
+- 智慧导航:配备划词搜索和翻译功能,帮助用户无缝理解专业术语,特别适用于跨语言研究。
+- 专业图表的智能生成:针对财报和研究报告,能够自动识别关键数据,生成杜邦分析图等专业图表,并结合内置的计算器功能确保数值的精准计算。
+
+
示例
+-
+
-
+
打开元宝 (https://yuanbao.tencent.com/) ,需要登录使用。界面很简洁,点击输入框上面的“深度阅读”
+
+
弹出文件上传框,拖动pdf到上传框。
+-
+
-
+
阅读界面如下,可以看到总结、精度、翻译、脑图功能。还可以直接根据文献内容生成学术海报。所有生成结果均可下载。下载格式为图片和pdf,生成的pdf对公示显示不友好。
+
+
-
+
-
+
文献阅读部分结果展示:
+
+
目的
-小说离线阅读,保存。练习R语言。示例网站:https://www.-----.com/yuedu/15111/
+处理ImageJ测量的果蝇攀爬数据
实例
-library("stringr") |
library(cluster) |
+ +

+ +

+ +

+ +

+ +

+ +

+ +

+ +

+ +

+ +

+ +

+ +

+ +

+ +

+ +

+ +

+ +

+ +

+ +

+ +

+ +

+ +

+ +

+ +

+ +

+ +

+ +

+ +

+ +

+ +

+ +

+ +

+ +

+ +

+ +

+ +

+ +

+ +

+ +

+ +

+ +

+ +

+ +

+ +

+ +
