diff --git a/README.zh-CN.md b/README.zh-CN.md
index 315f087ffbc4..f1dc961ee392 100644
--- a/README.zh-CN.md
+++ b/README.zh-CN.md
@@ -4,7 +4,7 @@
-[中文](https://docs.ultralytics.com/zh/) | [한국어](https://docs.ultralytics.com/ko/) | [日本語](https://docs.ultralytics.com/ja/) | [Русский](https://docs.ultralytics.com/ru/) | [Deutsch](https://docs.ultralytics.com/de/) | [Français](https://docs.ultralytics.com/fr/) | [Español](https://docs.ultralytics.com/es/) | [Português](https://docs.ultralytics.com/pt/) | [Türkçe](https://docs.ultralytics.com/tr/) | [Tiếng Việt](https://docs.ultralytics.com/vi/) | [العربية](https://docs.ultralytics.com/ar/)
+[中文](https://docs.ultralytics.com/zh) | [한국어](https://docs.ultralytics.com/ko) | [日本語](https://docs.ultralytics.com/ja) | [Русский](https://docs.ultralytics.com/ru) | [Deutsch](https://docs.ultralytics.com/de) | [Français](https://docs.ultralytics.com/fr) | [Español](https://docs.ultralytics.com/es) | [Português](https://docs.ultralytics.com/pt) | [Türkçe](https://docs.ultralytics.com/tr) | [Tiếng Việt](https://docs.ultralytics.com/vi) | [العربية](https://docs.ultralytics.com/ar)

@@ -22,7 +22,7 @@ YOLOv5 🚀 是世界上最受欢迎的视觉 AI,代表
文档 了解详细信息,在
GitHub 上提交问题以获得支持,并加入我们的
Discord 社区进行问题和讨论!
-如需申请企业许可,请在 [Ultralytics Licensing](https://ultralytics.com/license) 处填写表格
+如需申请企业许可,请在 [Ultralytics Licensing](https://www.ultralytics.com/license) 处填写表格

@@ -180,13 +180,13 @@ python train.py --data coco.yaml --epochs 300 --weights '' --cfg yolov5n.yaml -
-| Roboflow | ClearML ⭐ 新 | Comet ⭐ 新 | Neural Magic ⭐ 新 |
-| :--------------------------------------------------------------------------------------------------------: | :--------------------------------------------------------------------------------------------: | :--------------------------------------------------------------------------------------------------------------: | :----------------------------------------------------------------------------------------------------: |
-| 将您的自定义数据集进行标注并直接导出到 YOLOv5 以进行训练 [Roboflow](https://roboflow.com/?ref=ultralytics) | 自动跟踪、可视化甚至远程训练 YOLOv5 [ClearML](https://cutt.ly/yolov5-readme-clearml)(开源!) | 永远免费,[Comet](https://bit.ly/yolov5-readme-comet2)可让您保存 YOLOv5 模型、恢复训练以及交互式可视化和调试预测 | 使用 [Neural Magic DeepSparse](https://bit.ly/yolov5-neuralmagic),运行 YOLOv5 推理的速度最高可提高6倍 |
+| Roboflow | ClearML ⭐ 新 | Comet ⭐ 新 | Neural Magic ⭐ 新 |
+| :--------------------------------------------------------------------------------------------------------: | :------------------------------------------------------------------------: | :--------------------------------------------------------------------------------------------------------------: | :----------------------------------------------------------------------------------------------------: |
+| 将您的自定义数据集进行标注并直接导出到 YOLOv5 以进行训练 [Roboflow](https://roboflow.com/?ref=ultralytics) | 自动跟踪、可视化甚至远程训练 YOLOv5 [ClearML](https://clear.ml/)(开源!) | 永远免费,[Comet](https://bit.ly/yolov5-readme-comet2)可让您保存 YOLOv5 模型、恢复训练以及交互式可视化和调试预测 | 使用 [Neural Magic DeepSparse](https://bit.ly/yolov5-neuralmagic),运行 YOLOv5 推理的速度最高可提高6倍 |
##
Ultralytics HUB
-[Ultralytics HUB](https://ultralytics.com/hub) 是我们的⭐**新的**用于可视化数据集、训练 YOLOv5 🚀 模型并以无缝体验部署到现实世界的无代码解决方案。现在开始 **免费** 使用他!
+[Ultralytics HUB](https://www.ultralytics.com/hub) 是我们的⭐**新的**用于可视化数据集、训练 YOLOv5 🚀 模型并以无缝体验部署到现实世界的无代码解决方案。现在开始 **免费** 使用他!

@@ -430,7 +430,7 @@ python export.py --weights yolov5s-cls.pt resnet50.pt efficientnet_b0.pt --inclu
##
贡献
-我们喜欢您的意见或建议!我们希望尽可能简单和透明地为 YOLOv5 做出贡献。请看我们的 [投稿指南](https://docs.ultralytics.com/help/contributing/),并填写 [YOLOv5调查](https://ultralytics.com/survey?utm_source=github&utm_medium=social&utm_campaign=Survey) 向我们发送您的体验反馈。感谢我们所有的贡献者!
+我们喜欢您的意见或建议!我们希望尽可能简单和透明地为 YOLOv5 做出贡献。请看我们的 [投稿指南](https://docs.ultralytics.com/help/contributing/),并填写 [YOLOv5调查](https://www.ultralytics.com/survey?utm_source=github&utm_medium=social&utm_campaign=Survey) 向我们发送您的体验反馈。感谢我们所有的贡献者!
@@ -441,12 +441,12 @@ python export.py --weights yolov5s-cls.pt resnet50.pt efficientnet_b0.pt --inclu
Ultralytics 提供两种许可证选项以适应各种使用场景:
-- **AGPL-3.0 许可证**:这个[OSI 批准](https://opensource.org/licenses/)的开源许可证非常适合学生和爱好者,可以推动开放的协作和知识分享。请查看[LICENSE](https://github.com/ultralytics/yolov5/blob/master/LICENSE) 文件以了解更多细节。
-- **企业许可证**:专为商业用途设计,该许可证允许将 Ultralytics 的软件和 AI 模型无缝集成到商业产品和服务中,从而绕过 AGPL-3.0 的开源要求。如果您的场景涉及将我们的解决方案嵌入到商业产品中,请通过 [Ultralytics Licensing](https://ultralytics.com/license)与我们联系。
+- **AGPL-3.0 许可证**:这个[OSI 批准](https://opensource.org/license)的开源许可证非常适合学生和爱好者,可以推动开放的协作和知识分享。请查看[LICENSE](https://github.com/ultralytics/yolov5/blob/master/LICENSE) 文件以了解更多细节。
+- **企业许可证**:专为商业用途设计,该许可证允许将 Ultralytics 的软件和 AI 模型无缝集成到商业产品和服务中,从而绕过 AGPL-3.0 的开源要求。如果您的场景涉及将我们的解决方案嵌入到商业产品中,请通过 [Ultralytics Licensing](https://www.ultralytics.com/license)与我们联系。
##
联系方式
-对于 Ultralytics 的错误报告和功能请求,请访问 [GitHub Issues](https://github.com/ultralytics/yolov5/issues),并加入我们的 [Discord](https://ultralytics.com/discord) 社区进行问题和讨论!
+对于 Ultralytics 的错误报告和功能请求,请访问 [GitHub Issues](https://github.com/ultralytics/yolov5/issues),并加入我们的 [Discord](https://discord.com/invite/ultralytics) 社区进行问题和讨论!
diff --git a/export.py b/export.py
index 2c697eca1c96..9c6873939f7b 100644
--- a/export.py
+++ b/export.py
@@ -566,11 +566,7 @@ def export_coreml(model, im, file, int8, half, nms, mlmodel, prefix=colorstr("Co
else:
f = file.with_suffix(".mlpackage")
convert_to = "mlprogram"
- if half:
- precision = ct.precision.FLOAT16
- else:
- precision = ct.precision.FLOAT32
-
+ precision = ct.precision.FLOAT16 if half else ct.precision.FLOAT32
if nms:
model = iOSModel(model, im)
ts = torch.jit.trace(model, im, strict=False) # TorchScript model
@@ -1138,11 +1134,7 @@ def pipeline_coreml(model, im, file, names, y, mlmodel, prefix=colorstr("CoreML
import coremltools as ct
from PIL import Image
- if mlmodel:
- f = file.with_suffix(".mlmodel") # filename
- else:
- f = file.with_suffix(".mlpackage") # filename
-
+ f = file.with_suffix(".mlmodel") if mlmodel else file.with_suffix(".mlpackage")
print(f"{prefix} starting pipeline with coremltools {ct.__version__}...")
batch_size, ch, h, w = list(im.shape) # BCHW
t = time.time()
@@ -1186,10 +1178,7 @@ def pipeline_coreml(model, im, file, names, y, mlmodel, prefix=colorstr("CoreML
# Model from spec
weights_dir = None
- if mlmodel:
- weights_dir = None
- else:
- weights_dir = str(f / "Data/com.apple.CoreML/weights")
+ weights_dir = None if mlmodel else str(f / "Data/com.apple.CoreML/weights")
model = ct.models.MLModel(spec, weights_dir=weights_dir)
# 3. Create NMS protobuf
diff --git a/utils/flask_rest_api/README.md b/utils/flask_rest_api/README.md
index b18a3011cf32..d3ffaa2069ac 100644
--- a/utils/flask_rest_api/README.md
+++ b/utils/flask_rest_api/README.md
@@ -4,7 +4,7 @@
## Requirements
-[Flask](https://palletsprojects.com/p/flask/) is required. Install with:
+[Flask](https://palletsprojects.com/projects/flask/) is required. Install with:
```shell
$ pip install Flask
diff --git a/utils/loggers/clearml/README.md b/utils/loggers/clearml/README.md
index a61e3025445f..cf5f787b1a5d 100644
--- a/utils/loggers/clearml/README.md
+++ b/utils/loggers/clearml/README.md
@@ -4,7 +4,7 @@
## About ClearML
-[ClearML](https://cutt.ly/yolov5-tutorial-clearml) is an [open-source](https://github.com/allegroai/clearml) toolbox designed to save you time ⏱️.
+[ClearML](https://clear.ml) is an [open-source](https://github.com/allegroai/clearml) toolbox designed to save you time ⏱️.
🔨 Track every YOLOv5 training run in the experiment manager
@@ -18,13 +18,13 @@
And so much more. It's up to you how many of these tools you want to use, you can stick to the experiment manager, or chain them all together into an impressive pipeline!
-
+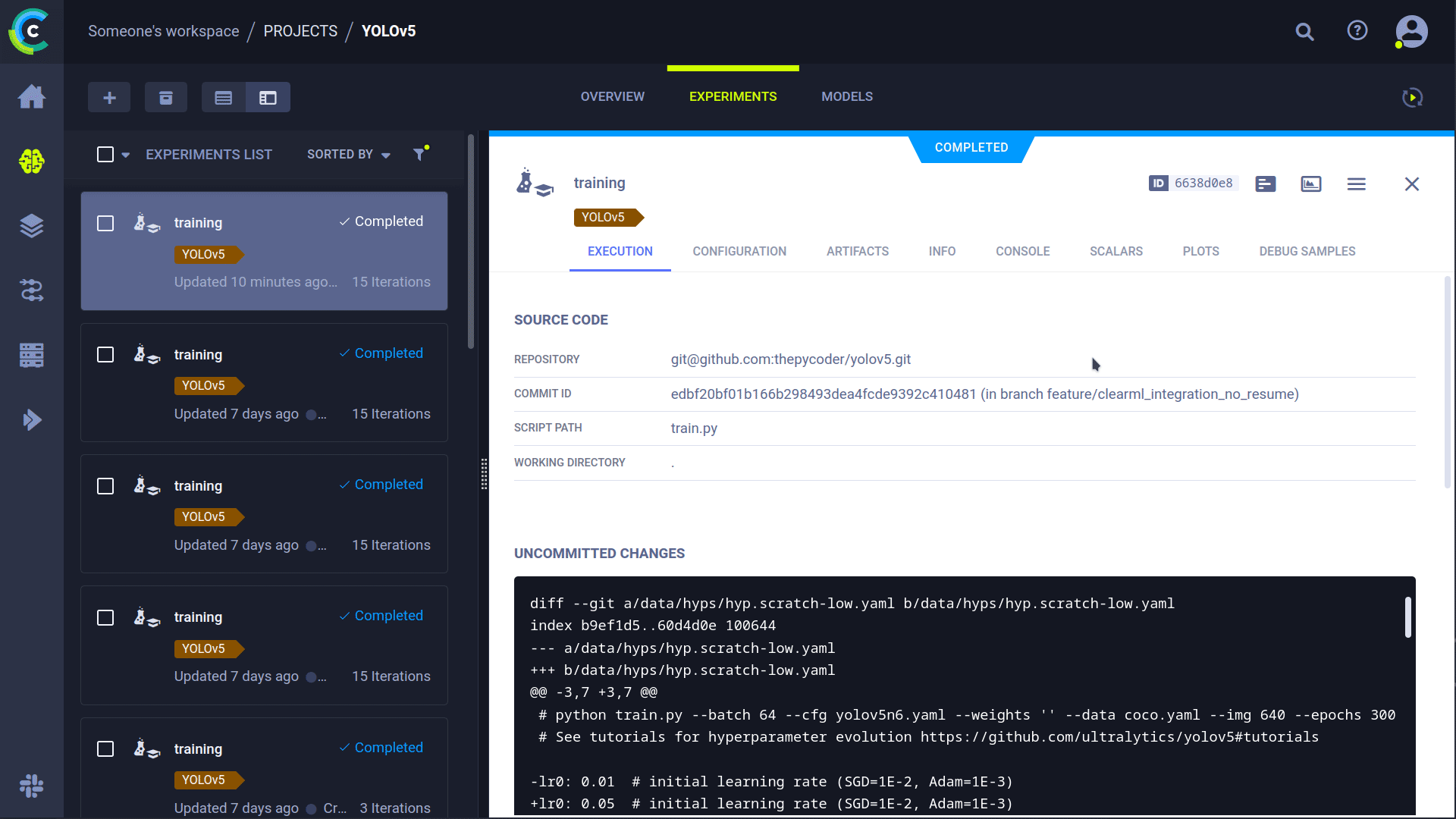
## 🦾 Setting Things Up
To keep track of your experiments and/or data, ClearML needs to communicate to a server. You have 2 options to get one:
-Either sign up for free to the [ClearML Hosted Service](https://cutt.ly/yolov5-tutorial-clearml) or you can set up your own server, see [here](https://clear.ml/docs/latest/docs/deploying_clearml/clearml_server). Even the server is open-source, so even if you're dealing with sensitive data, you should be good to go!
+Either sign up for free to the [ClearML Hosted Service](https://clear.ml) or you can set up your own server, see [here](https://clear.ml/docs/latest/docs/deploying_clearml/clearml_server). Even the server is open-source, so even if you're dealing with sensitive data, you should be good to go!
1. Install the `clearml` python package:
@@ -85,7 +85,7 @@ There even more we can do with all of this information, like hyperparameter opti
Versioning your data separately from your code is generally a good idea and makes it easy to acquire the latest version too. This repository supports supplying a dataset version ID, and it will make sure to get the data if it's not there yet. Next to that, this workflow also saves the used dataset ID as part of the task parameters, so you will always know for sure which data was used in which experiment!
-
+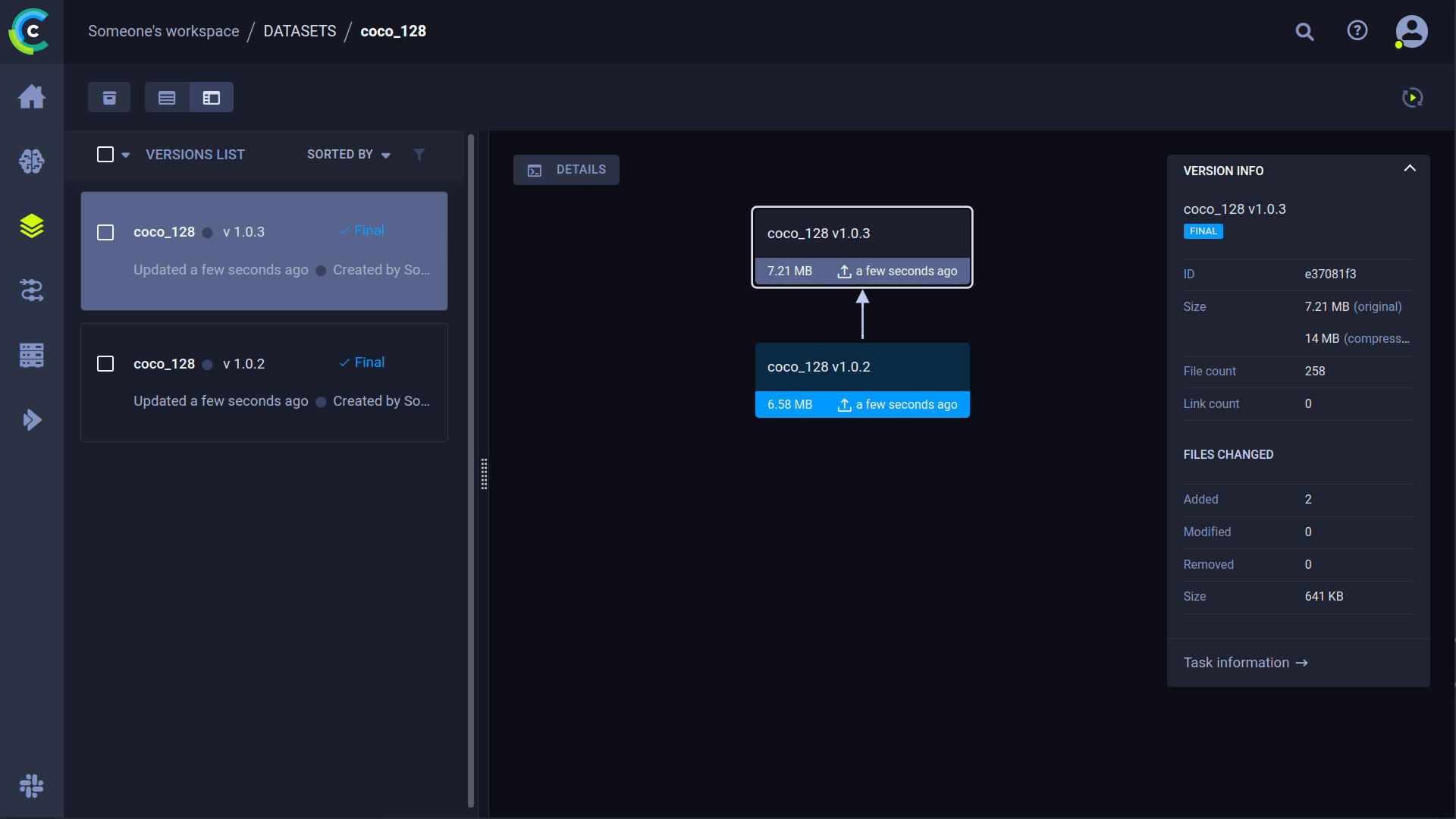
### Prepare Your Dataset
@@ -163,13 +163,13 @@ pip install optuna
python utils/loggers/clearml/hpo.py
```
-
+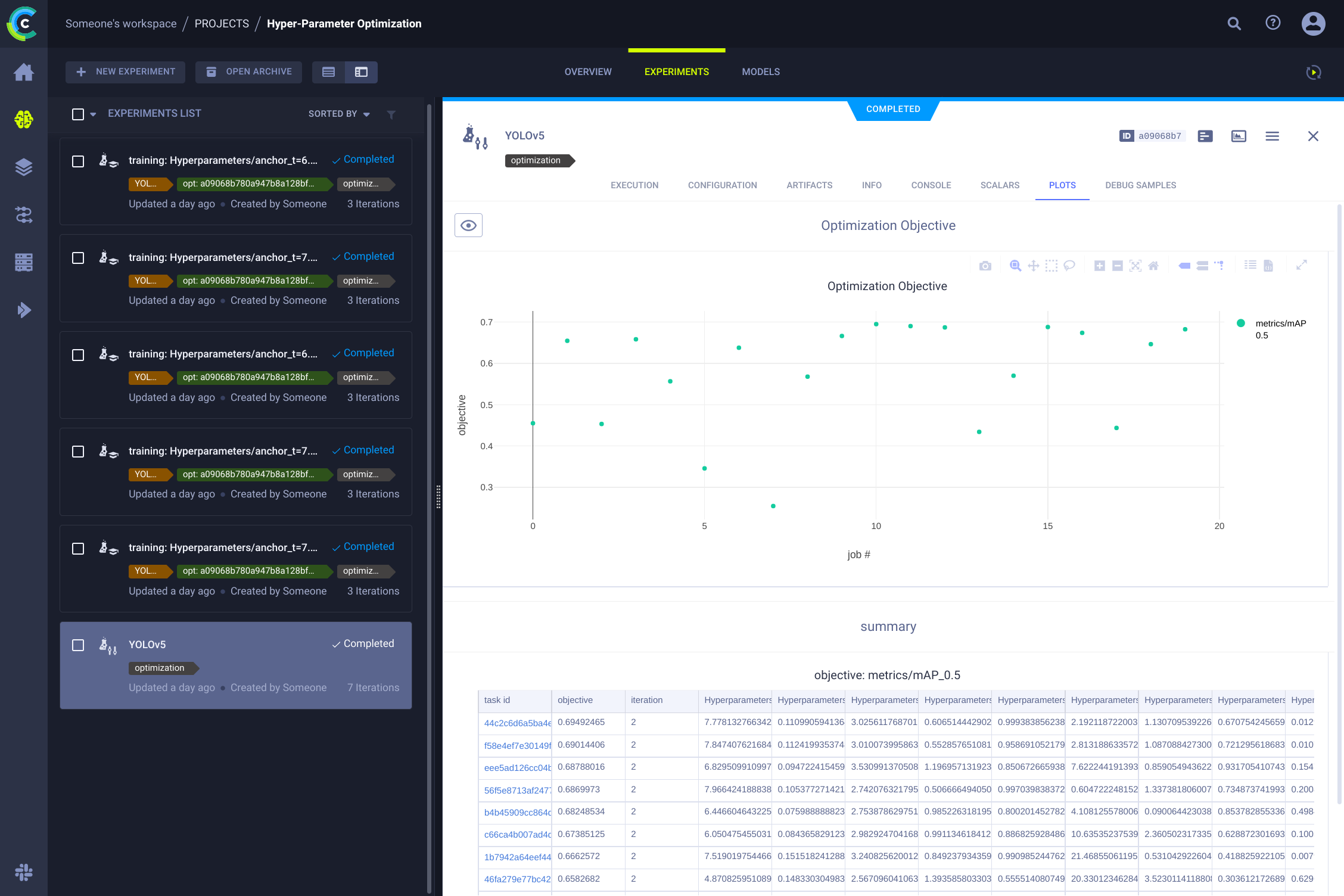
## 🤯 Remote Execution (advanced)
Running HPO locally is really handy, but what if we want to run our experiments on a remote machine instead? Maybe you have access to a very powerful GPU machine on-site, or you have some budget to use cloud GPUs. This is where the ClearML Agent comes into play. Check out what the agent can do here:
-- [YouTube video](https://youtu.be/MX3BrXnaULs)
+- [YouTube video](https://www.youtube.com/watch?v=MX3BrXnaULs&feature=youtu.be)
- [Documentation](https://clear.ml/docs/latest/docs/clearml_agent)
In short: every experiment tracked by the experiment manager contains enough information to reproduce it on a different machine (installed packages, uncommitted changes etc.). So a ClearML agent does just that: it listens to a queue for incoming tasks and when it finds one, it recreates the environment and runs it while still reporting scalars, plots etc. to the experiment manager.
@@ -190,7 +190,7 @@ With our agent running, we can give it some work. Remember from the HPO section
⏳ Enqueue the task to any of the queues by right-clicking it
-
+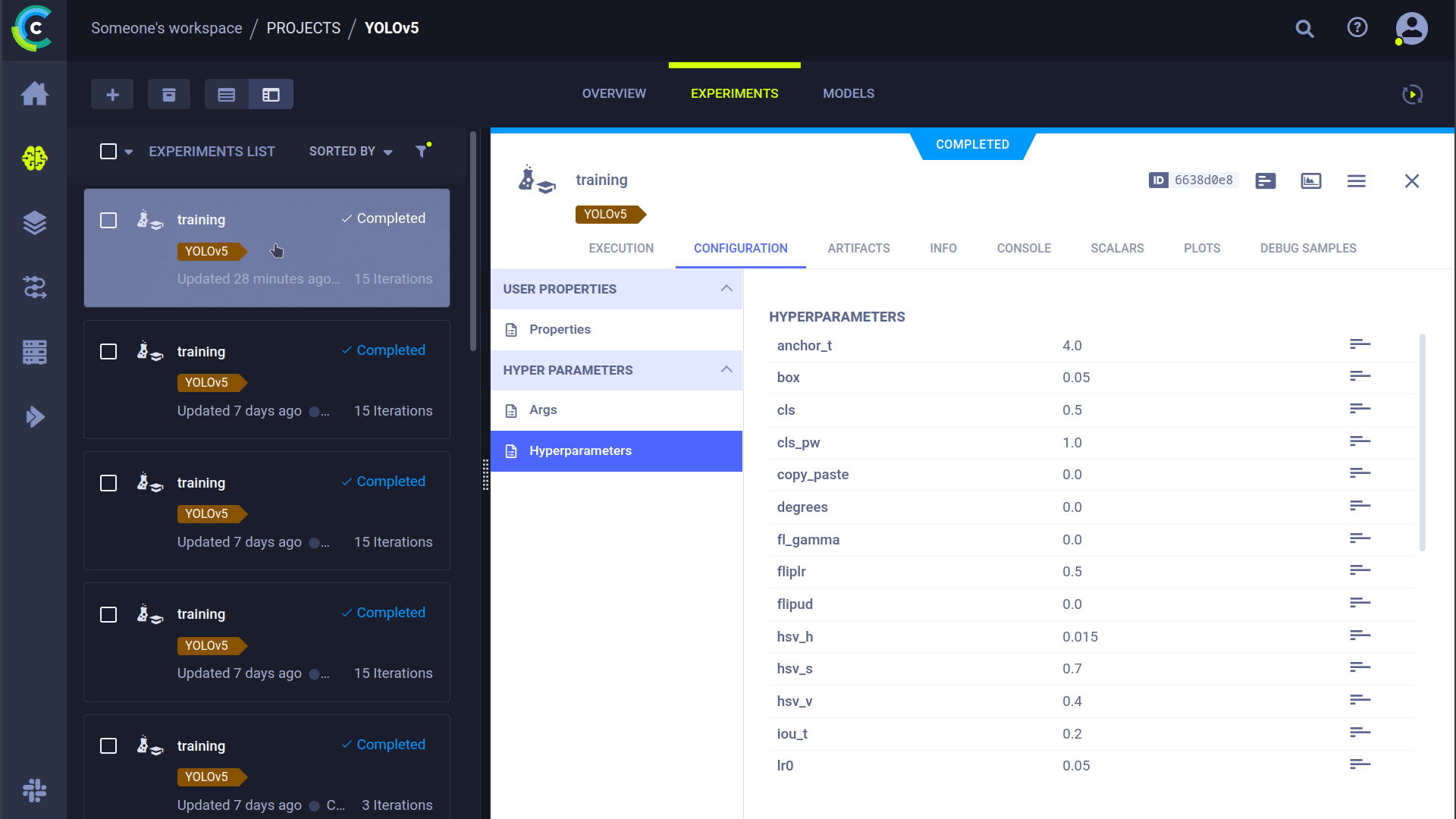
### Executing A Task Remotely