forked from wala/ML
-
Notifications
You must be signed in to change notification settings - Fork 1
Commit
This commit does not belong to any branch on this repository, and may belong to a fork outside of the repository.
Add the ability to process datasets coming out of enumerate() (#71)
* Add neural network test. * New tests. * Change log message. * Add Dataset.repeat(). * Add log. * Add prefetch. * Add Dataset.take(). * Add tf.nn.softmax(). * Add the ability to process datasets coming out of enumerate().
- Loading branch information
Showing
4 changed files
with
297 additions
and
16 deletions.
There are no files selected for viewing
This file contains bidirectional Unicode text that may be interpreted or compiled differently than what appears below. To review, open the file in an editor that reveals hidden Unicode characters.
Learn more about bidirectional Unicode characters
This file contains bidirectional Unicode text that may be interpreted or compiled differently than what appears below. To review, open the file in an editor that reveals hidden Unicode characters.
Learn more about bidirectional Unicode characters
This file contains bidirectional Unicode text that may be interpreted or compiled differently than what appears below. To review, open the file in an editor that reveals hidden Unicode characters.
Learn more about bidirectional Unicode characters
This file contains bidirectional Unicode text that may be interpreted or compiled differently than what appears below. To review, open the file in an editor that reveals hidden Unicode characters.
Learn more about bidirectional Unicode characters
Original file line number | Diff line number | Diff line change |
---|---|---|
@@ -0,0 +1,184 @@ | ||
# From https://github.com/aymericdamien/TensorFlow-Examples/blob/6dcbe14649163814e72a22a999f20c5e247ce988/tensorflow_v2/notebooks/3_NeuralNetworks/neural_network.ipynb. | ||
|
||
# %% | ||
# # Neural Network Example | ||
|
||
# Build a 2-hidden layers fully connected neural network (a.k.a multilayer perceptron) with TensorFlow v2. | ||
|
||
# This example is using a low-level approach to better understand all mechanics behind building neural networks and the training process. | ||
|
||
# - Author: Aymeric Damien | ||
# - Project: https://github.com/aymericdamien/TensorFlow-Examples/ | ||
# """ | ||
|
||
# %% | ||
# ## Neural Network Overview | ||
|
||
# <img src="http://cs231n.github.io/assets/nn1/neural_net2.jpeg" alt="nn" style="width: 400px;"/> | ||
|
||
# ## MNIST Dataset Overview | ||
|
||
# This example is using MNIST handwritten digits. The dataset contains 60,000 examples for training and 10,000 examples for testing. The digits have been size-normalized and centered in a fixed-size image (28x28 pixels) with values from 0 to 255. | ||
|
||
# In this example, each image will be converted to float32, normalized to [0, 1] and flattened to a 1-D array of 784 features (28*28). | ||
|
||
# 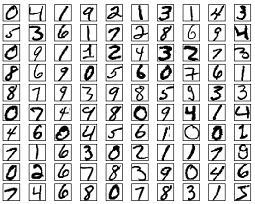 | ||
|
||
# More info: http://yann.lecun.com/exdb/mnist/ | ||
|
||
# %% | ||
from __future__ import absolute_import, division, print_function | ||
|
||
import tensorflow as tf | ||
print("TensorFlow version:", tf.__version__) | ||
assert(tf.__version__ == "2.15.0") | ||
from tensorflow.keras import Model, layers | ||
import numpy as np | ||
import timeit | ||
|
||
start_time = timeit.default_timer() | ||
skipped_time = 0 | ||
|
||
# %% | ||
# MNIST dataset parameters. | ||
num_classes = 10 # total classes (0-9 digits). | ||
num_features = 784 # data features (img shape: 28*28). | ||
|
||
# Training parameters. | ||
learning_rate = 0.1 | ||
training_steps = 20000 | ||
batch_size = 256 | ||
display_step = 100 | ||
|
||
# Network parameters. | ||
n_hidden_1 = 128 # 1st layer number of neurons. | ||
n_hidden_2 = 256 # 2nd layer number of neurons. | ||
|
||
# %% | ||
# Prepare MNIST data. | ||
from tensorflow.keras.datasets import mnist | ||
(x_train, y_train), (x_test, y_test) = mnist.load_data() | ||
# Convert to float32. | ||
x_train, x_test = np.array(x_train, np.float32), np.array(x_test, np.float32) | ||
# Flatten images to 1-D vector of 784 features (28*28). | ||
x_train, x_test = x_train.reshape([-1, num_features]), x_test.reshape([-1, num_features]) | ||
# Normalize images value from [0, 255] to [0, 1]. | ||
x_train, x_test = x_train / 255., x_test / 255. | ||
|
||
# %% | ||
# Use tf.data API to shuffle and batch data. | ||
train_data = tf.data.Dataset.from_tensor_slices((x_train, y_train)) | ||
train_data = train_data.repeat().shuffle(5000).batch(batch_size).prefetch(1) | ||
|
||
|
||
# %% | ||
# Create TF Model. | ||
class NeuralNet(Model): | ||
|
||
# Set layers. | ||
def __init__(self): | ||
super(NeuralNet, self).__init__() | ||
# First fully-connected hidden layer. | ||
self.fc1 = layers.Dense(n_hidden_1, activation=tf.nn.relu) | ||
# First fully-connected hidden layer. | ||
self.fc2 = layers.Dense(n_hidden_2, activation=tf.nn.relu) | ||
# Second fully-connecter hidden layer. | ||
self.out = layers.Dense(num_classes) | ||
|
||
# Set forward pass. | ||
def call(self, x, is_training=False): | ||
x = self.fc1(x) | ||
x = self.fc2(x) | ||
x = self.out(x) | ||
if not is_training: | ||
# tf cross entropy expect logits without softmax, so only | ||
# apply softmax when not training. | ||
x = tf.nn.softmax(x) | ||
return x | ||
|
||
|
||
# Build neural network model. | ||
neural_net = NeuralNet() | ||
|
||
|
||
# %% | ||
# Cross-Entropy Loss. | ||
# Note that this will apply 'softmax' to the logits. | ||
def cross_entropy_loss(x, y): | ||
# Convert labels to int 64 for tf cross-entropy function. | ||
y = tf.cast(y, tf.int64) | ||
# Apply softmax to logits and compute cross-entropy. | ||
loss = tf.nn.sparse_softmax_cross_entropy_with_logits(labels=y, logits=x) | ||
# Average loss across the batch. | ||
return tf.reduce_mean(loss) | ||
|
||
|
||
# Accuracy metric. | ||
def accuracy(y_pred, y_true): | ||
# Predicted class is the index of highest score in prediction vector (i.e. argmax). | ||
correct_prediction = tf.equal(tf.argmax(y_pred, 1), tf.cast(y_true, tf.int64)) | ||
return tf.reduce_mean(tf.cast(correct_prediction, tf.float32), axis=-1) | ||
|
||
|
||
# Stochastic gradient descent optimizer. | ||
optimizer = tf.optimizers.SGD(learning_rate) | ||
|
||
|
||
# %% | ||
# Optimization process. | ||
def run_optimization(x, y): | ||
# Wrap computation inside a GradientTape for automatic differentiation. | ||
with tf.GradientTape() as g: | ||
# Forward pass. | ||
pred = neural_net(x, is_training=True) | ||
# Compute loss. | ||
loss = cross_entropy_loss(pred, y) | ||
|
||
# Variables to update, i.e. trainable variables. | ||
trainable_variables = neural_net.trainable_variables | ||
|
||
# Compute gradients. | ||
gradients = g.gradient(loss, trainable_variables) | ||
|
||
# Update W and b following gradients. | ||
optimizer.apply_gradients(zip(gradients, trainable_variables)) | ||
|
||
|
||
# %% | ||
# Run training for the given number of steps. | ||
for step, (batch_x, batch_y) in enumerate(train_data.take(training_steps), 1): | ||
# Run the optimization to update W and b values. | ||
run_optimization(batch_x, batch_y) | ||
|
||
if step % display_step == 0: | ||
pred = neural_net(batch_x, is_training=True) | ||
loss = cross_entropy_loss(pred, batch_y) | ||
acc = accuracy(pred, batch_y) | ||
print_time = timeit.default_timer() | ||
print("step: %i, loss: %f, accuracy: %f" % (step, loss, acc)) | ||
skipped_time += timeit.default_timer() - print_time | ||
|
||
# %% | ||
# Test model on validation set. | ||
pred = neural_net(x_test, is_training=False) | ||
print_time = timeit.default_timer() | ||
print("Test Accuracy: %f" % accuracy(pred, y_test)) | ||
skipped_time += timeit.default_timer() - print_time | ||
|
||
# %% | ||
# Visualize predictions. | ||
import matplotlib.pyplot as plt | ||
|
||
# %% | ||
# Predict 5 images from validation set. | ||
n_images = 5 | ||
test_images = x_test[:n_images] | ||
predictions = neural_net(test_images) | ||
|
||
print("Elapsed time: ", timeit.default_timer() - start_time - skipped_time) | ||
|
||
# Display image and model prediction. | ||
for i in range(n_images): | ||
plt.imshow(np.reshape(test_images[i], [28, 28]), cmap='gray') | ||
plt.show() | ||
print("Model prediction: %i" % np.argmax(predictions.numpy()[i])) |